BACKGROUND AND AIMS
Rheumatic and musculoskeletal diseases (RMD) affect up to one-third of the UK population, are the number one cause of disability, and are one of the most common reasons for people of working age having an increased number of sick days or unemployment.1 Patients with RMD experience periods of exacerbation of disease, known as flares, which are associated with pain and function limitations for patients.2 If the disease flare is detected and treated late, this can lead to joint damage and reduced function.
RMD flares are unpredictable, and there is no marker identified for an impending flare. Sudden flares may lead to additional hospitalisations and follow-up visits,and decreased quality of life in general.3 However, there is currently no risk stratification model that can predict patient flare early and accurately in practice.
The authors aimed to develop and evaluate a machine learning-based risk stratification model that can predict the risk of future disease flares and guide early and accurate intervention to prevent flares in patients with RMD.
METHODS
In this study, 142,067 patients who had been diagnosed with RMD in the rheumatology department of Royal Berkshire NHS Foundation Trust (UK) from January 1, 2016–May 7, 2024 were included. This included 58,727 patients who had flares and 83,340 patients who did not have flares. The authors utilised patient blood test results, demographic information, electronic patient-reported outcomes scores, comorbidity, weight, and height. They developed a time series dataset and applied Long Short-Term Memory networks and neural networks to forecast the risk of flare before their future clinics.
RESULTS
The authors conducted predictive modelling to forecast flare and non-flare events using data collected prior to patients’ upcoming clinic appointments. The dataset was split into training and testing sets based on observations taken 3 months and 6 months before the clinic visit. Their applied Long Short-Term Memory-based model achieved 89.32% accuracy and 0.76 Area Under the Receiver Operating Characteristic Curve (AUROC), predicting flare and non-flare events 6 months before the clinics, and 81.93% accuracy and 0.68 AUROC 3 months before. The results are summarised in Table 1.
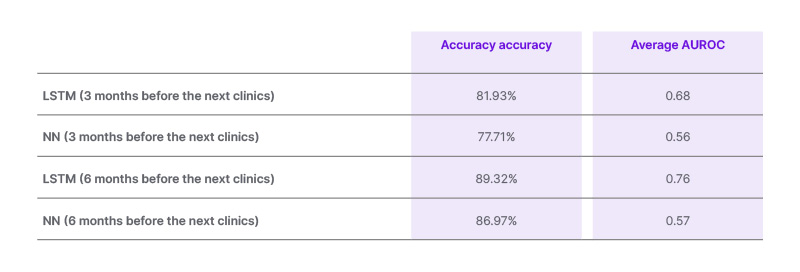
Table 1: Performance of Long Short-Term Memory and neural networks on data from 3 months and 6 months before the clinic visit.
Prediction 6 months in advance is more accurate than 3 months in advance. LSTM exhibited superior performance in average accuracy and AUROC compared to NN.
AUROC: Area Under the Receiver Operating Characteristic Curve; LSTM: Long Short-Term Memory; NN: neural networks.
CONCLUSION
The authors’ model can predict future flare and non-flare events with 89.93% accuracy 6 months in advance. Further work includes using advanced data processing methods to handle missing values to improve accuracy, especially for predictions 3 months in advance. If implemented in practice, the flare-risk stratification model can be used to aid patients and clinicians in prognosticating the risk of flares quickly. This flare-risk prediction can aid the patients and clinicians in recognising if they are at a trend of flares or reduction in the efficacy of their treatment. With the informed risk prediction presented early and more accurately,