BACKGROUND AND AIMS
Seizure unpredictability is consistently reported as one of the most disabling aspects of living with chronic epilepsy. Reliable forecasting systems could have far-reaching consequences for patients, from allowing protection at times of high seizure risk to improving confidence during everyday life at times of low seizure risk.1,2 The feasibility of forecasting has been demonstrated with chronic intracerebral electroencephalographic (EEG) recordings;3 however, invasive devices carry significant risks and are not appropriate for all patients.
The Epilepsy Foundation’s My Seizure Gauge Project is an ongoing multicentre study aiming to determine the feasibility of seizure forecasting with non-invasive and minimally invasive mobile devices (Figure 1).
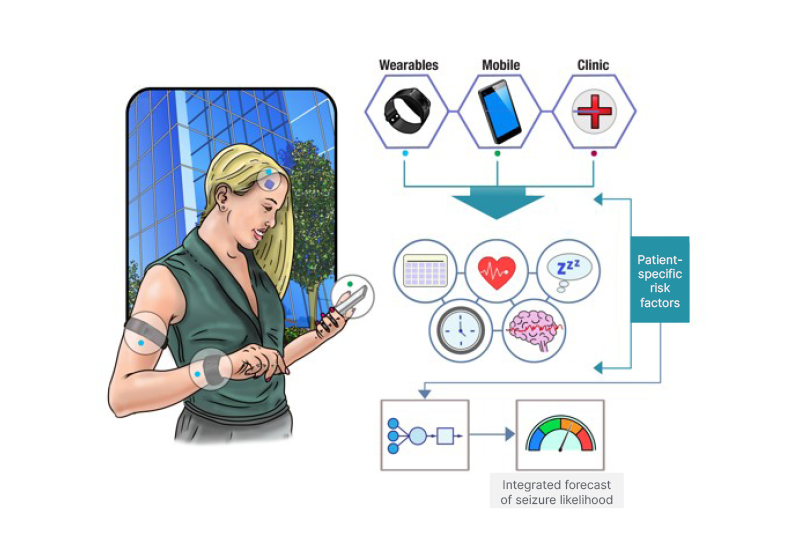
Figure 1: The overarching goal of the Epilepsy Foundation’s My Seizure Gauge project is to establish the feasibility of seizure forecasting using non-invasive and minimally invasive mobile devices.
Multimodal data can be incorporated into patient-specific forecasts of seizure risk.
MATERIALS AND METHODS
Adult patients with active (>10 seizures/6 months) drug-resistant epilepsy were enrolled for ultra-long-term (>8 months) monitoring. Patients were asked to self-report their seizures on an electronic diary (Seer app [Seer, Melbourne, Australia]) while using a wearable device (Empatica E4 [Empatica, Boston, Massachusetts, USA], Fitbit Charge 4/HR [Fitbit, San Francisco, Calirfornia, USA], or Fitbit Inspire) and simultaneous chronic ambulatory EEG monitoring (RNS [NeuroPace, Mountain View, California, USA], 24/7 EEG™ SubQ [UNEEG, Allerød, Denmark] or Epi-Minder sub-scalp [Bionics Institute, Melbourne, Australia] systems). Recorded data from these multiple modalities was analysed via traditional machine learning or deep learning methods, together with the identification of patient-specific circadian and multiday seizure risk cycles to forecast seizures.
RESULTS
To date, 40 enrolled subjects have recorded over 11,400 days (>31 years) of ambulatory data, and over 1,700 seizures have been annotated. Nine patients left the study prematurely due to device malfunctions, complications, poor adherence, poor data quality, or unanticipated seizure freedom. However, 20 patients are continuing to record data and 11 have completed the study.
Selected results from analysis of this cohort have been presented. Circadian and multiday seizure cycles were detected with ultra-long-term subcutaneous EEG.4,5 Heart rate circadian and multiday cycles were also detected in a high proportion of patients using a commercial fitness tracker (Fitbit), and were found to correlate with self-reported seizure likelihood.6 Using data from a multimodal wearable device (Empatica E4), electrodermal activity, heart-rate, and accelerometery data were significantly correlated with electrographic seizures detected by the RNS device in 11 patients. In another group of 11 subjects, patient-specific seizure forecasts trained on Fitibit data were found to be significantly better than chance in 91–100% of subjects.7 Seizure forecasting was also better than chance in five out of six patients using the Empatica E4 data, validated on RNS-detected seizures.8 Finally, seizure forecasting using ultra-long-term subcutaneous EEG data was significantly better than chance in at least 50% of patients in a six-patient cohort.9,10
CONCLUSION
This project has established the feasibility of forecasting seizures using seizure cycles, wearable devices, and subcutaneous EEG. Seizure cycles are common in patients with epilepsy. They are measurable across a range of non-invasive wearables, minimally invasive EEG, and intracranial EEG devices, and they are strong forecasts of seizure likelihood. The next steps in this project are to establish a freely available data science competition for forecasting using wearable data, and to trial a prospective seizure forecasting smartphone app (Seer), which is now available to the public.