Abstract
Electronic medical record (EMR)-based precision oncology is a vision that is so far limited to a few pilot and basket studies, with the goal being the design of a proper treatment for cancer patients in real time, based on the panomics knowledge of the patient, and that of similar types of patients. It aims to deliver better treatment outcomes through the design of rational drug combinations, a lower number of futile therapies, reduced patient discomfort, and a healthy human society with a reduced risk of cancer. The concept of precision oncology began with a few cancer awareness programmes and preventative screenings almost a decade ago. However, the technique took an astronomical leap with the start of the Precision Medicine Initiative Cohort Program and Cancer Moonshot programme very recently. Both projects have invested heavily towards several goals, including the merging of cancer registries and EMR to find the best treatment options for a cancer patient, an idea which, if extended globally, will generate unprecedented possibilities for precision oncology. EMR serve as a broad platform merging a variety of patient information and expert advice to facilitate co-ordinated cancer care. In this article, a summary of the recent EMR-based precision oncology practices for prevention, diagnosis, prognosis, prediction, and their associated concerns and limitations is presented. Though the path of precision oncology is unchartered, the usefulness of real-time information derived from EMR or electronic health records will lead to better precision decision-based oncotherapies.
INTRODUCTION
Cancer is a universal phenomenon, yet each cancer type differs from the other variants. In addition, individual human beings differ from one another by a genome difference of approximately 1%. This tiny difference in the genome sequence, in turn, causes a huge discordance in cancer treatment and drug resistance. The dimensionality of cancer networks is much higher compared to other disease systems; cancer is a genomic disease, harbouring a number of mutated oncogenes and tumour suppressor genes that specify the molecular pathways for tumour growth, sustenance, and progression. The network function depends on the individual patient, external factors, and the ability of the network to overcome treatment mechanisms. The treatment of cancer requires a combined effort among a varied team of experts, including counsellors, medical doctors, caregivers, pathologists, radiologists, surgeons, and others. Irrespective of best efforts, a complex disease like cancer is often unmanageable due to the involvement of genetic, lifestyle, and environmental factors. However, with the use of precision medicine, the situation is improving, albeit slowly. Even though a definitive cure for cancer has not been found, the life expectancy of certain cancer patients has certainly increased.
Predictive, preventative, personalised, and participatory (known as P4) treatment of each individual patient, often coupled with population perspective,1-3 is one of the definitions of precision medicine,4 also known as stratified medicine;5 it tries to impart proper treatment to a patient in real time.6,7 Identification, risk assessment, and targeted therapy for cancer patients by considering resources like the genomic sequence, the prevalence of rare mutations, and proteomic profile, among others, is known as precision cancer medicine or precision oncology.8
In simpler words, precision oncology can be defined as diagnosis, prognosis, prevention, treatment, and follow-up that are tailored specifically to an individual patient based on their genetic profile and supported by the genetic profiles of similar types of patients;9,10 it is an emerging approach for disease treatment and prevention that takes into account the genetic variability, environment, and lifestyle-related habits of each person.11
DISCUSSION
Precision oncology is applicable to various aspects of human health, for example, identification of presymptomatic individuals, detection of disease, patient-specific therapy, the study of disease evolution, the design of effective surveillance, and management techniques, among others; altogether, it focusses on providing a uniform platform for human health management.8 Traditional evidence-based medical practice along with precision medicine will pave the way for the achievement of such ambitious goals.
The idea of precision oncology gained momentum after a few ‘fit for all’ solutions, i.e., molecularly driven therapies that have been devised for certain types of cancers. At present, imatinib has dramatically improved the survival of chronic myeloid leukaemia patients12,13 and HER2-targeted therapies have improved survival rates of women with metastatic HER2-positive breast cancer.14,15 More detailed applications of precision oncology have been reported by Hyman et al.16 Precision oncology aims to reduce morbidity and mortality, along with improving clinical outcomes of cancer patients, as each patient and cancer type is unique, and so must be the precision decision for each treatment.
Catalogue maintenance and curation of cancer sequence variants are crucial for precision oncology and have immense clinical relevance.17 Profiling the somatic mutations of genes may predict tumour evolution, prognostics, and treatment. In this context, Tsang et al.18 reviewed various functional annotation resources and tools to interpret variants in precision genomic oncology. OncoPaD,19 a web-based tool, has been developed for the rational design of cancer next-generation sequencing (NGS) panels based on mutational data.20 Based on 7,298 cancer cases from 29 cancer types, it estimates the cost-effectiveness of the designed panel on a cohort of tumours and provides reports on the importance of individual mutations for oncogenesis and therapy. The tool helps in addressing many translational and basic research questions, including those regarding precision oncology. The advent of this NGS technique has a crucial role in precision cancer therapy, a subject that cannot be covered in the present article’s scope, but is well described elsewhere.21
However, the idea of large-scale precision oncology is burdened by many hurdles, for example, acquired resistance of tumours, cost-benefit analysis, clinical evaluation, clinical trial design, drug resistance, genetic and genomic analyses of tumours of patients, informatics methods to evaluate genetic variants, liquid biopsy data-related issues, target prioritisation, molecularly targeted drug effectiveness, and tumour heterogeneity, among others.22,23 Improvement is needed in the areas of genome sequencing of cancer patients, identification of targets, better access to clinical studies, and design of therapies. In addition, involvement of appropriate parties and stakeholders at every step is crucial for progress in precision oncology, starting from the patient, clinician, physician, caregiver, and third-party insurance providers. These issues should be properly addressed before precision oncology becomes a universal phenomenon for cancer treatment.
In this context, electronic medical records (EMR), the digital version of patient health records, play a crucial role in providing a means by which a patient’s previous health history or health history of patients with similar cancer types can be studied and used for real-time treatment. EMR serve as a broad platform that merges a variety of patient information and expert advice to facilitate co-ordinated cancer care.24 They aid in personalised cancer risk management and treatment by taking into account the behavioural and social contexts.5 An EMR-adapted healthcare system efficiently streamlines day-to-day hospital procedures, along with internal quality control checks, and facilitates easy access to patient data from anywhere around the globe following proper authentication from a user. EMR are changing the current practice of medicine, as expected almost a decade ago.11,25,26
Interestingly, the basket studies into the use of patient EMR for precision oncology12-15,21-23,26-35 have provided meaningful observations for better clinical outcomes. New knowledge developed from the large range of EMR data, with help from predictive models, has generated confidence for precision oncology-based decisions. Precision oncology is a nascent area of therapy but, gradually, with help from information technology and more EMR data, it will take shape from the variety of heterogeneous cancer-related patient data. Precision medicine alongside patient EMR will aid prevention, diagnosis, prognosis, and prediction of cancer types, often one form of assistance combined with others, for the improvement of cancer treatment. Here, we discuss the research developments, or rather the lack of them, in these specified areas.
Preventive Approach
There is currently little scope for adding any cancer prevention measures to a patient’s EMR, as hardly any such measures exist. Mostly, the preventive measures are limited to small cohort screenings22 but, as a Pre-Cancer Genome Atlas (PCGA)36 is being developed, it will be important to add information on preventative measures into EMR to help in individualised cancer care. For example, programmes like the Million Veterans Program37 and the Precision Medicine Initiative Cohort Program38 are adding EMR data into their repositories as part of their quest for cancer prevention. Similarly, there are many existing cancer data and knowledge-bases (Table 1) that will be necessary to include for the development of preventative, diagnostic, and prognostic approaches for cancer.
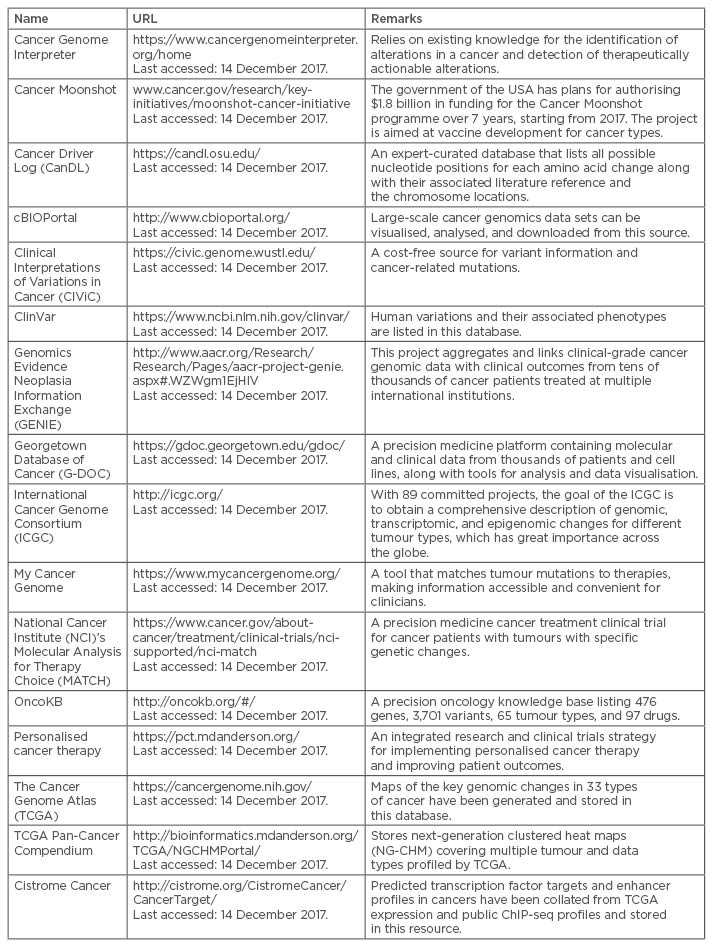
Table 1: Examples of existing cancer data and knowledge-bases.
Cancer Diagnosis
To date, the conventional method for cancer diagnosis is mostly based on histopathological reports and radiographic findings. However, the essential role of genomics in the form of biomarkers, molecular signatures, and the molecular profiling reports to detect cancer types cannot be negated. This information is now being stored in the EMR of cancer patients and, in the future, can be used for fast cancer categorisation. Precision cancer diagnostics extend beyond the conventional methods of gene expression profiling and EMR-based decisions. In this respect, we herein discuss some innovations that generate standardised onco-data for inclusion in EMR or electronic health records (EHR).
Pyradiomics39 is a Python programming language-based open-source radiomic quantification platform to extract radiomic features from computed tomography (CT), magnetic resonance imaging (MRI), and positron emission tomography (PET) images. It establishes a reference standard for radiomic analyses with immense significance that will reflect in cancer diagnosis.40 The Health Level Seven® (HL7) Clinical Genomics group41 is working towards the standardisation of genome data and harmonisation with EMR. LesionTracker42 is a web-based open-source image assessment and tracking platform for oncology clinical trial workflows. Tumor Map43 is an integrated portal for exploration and interrogation of cancer genomics data by using Google’s map technology. It can also facilitate identification of cancer subtypes in silico based on common molecular activities in a set of tumour samples.44 The inclusion of such data with EMR or EHR will be beneficial for further analyses at a later stage for diagnostic purposes.
Prognostic Approach
Nowadays, a few rapid learning health systems45-48 are in practice for sourcing prognostic and associative factors from text-based cancer EMR. Text-based Exploratory Pattern Analyser for Prognosticator and Associator discovery (TEPAPA)45 is a feature learning pipeline for identifying disease-associated factors from unstructured EMR narratives. It has already been applied to a cohort of 82 head and neck squamous cell carcinoma patients’ EMR for phenotyping. CancerLinQTM,46 a cancer decision support system, uses more than a million EMR for any kind of precision decision; it stores data from any format including EMR, provides real-time clinical decision support, measures clinical performance, and generates hypotheses from clinical data to provide lessons learned about technical and logistical challenges. Cancer Commons49 is a non-profit network of patients, physicians, and scientists who help in identifying the best options for treating an individual’s cancer. It facilitates rapid learning for precision oncology by gathering panomic and clinical data about individual patients from the Donate Your Data (DYD) registry.
Furthermore, the Melanoma Rapid Learning Utility (MRLU)47 is an analytical engine and user interface that provides clinical decision support for melanoma treatment by identifying and analysing cohorts from a database of 237 metastatic melanoma patients. Precision Oncology 3.048 advocates the analysis of panomic data to hypothesise the patient-specific cancer molecular pathways and their personalised treatments by combining the targeted therapies, and Watson,50 an IBM developed machine learning system, recommends trials and individualised therapies based on cancer patient data obtained from various sources, including EMR. The Electronic Medical Records and Genomics (eMERGE) Network51 is also helping to develop the EMR-guided cancer genomic studies and associated decisions.
In addition, cancer-associated research often reflects important information for the oncology community based on EMR data, a few of which are discussed. Gregg et al.27 performed a study of 2,352 prostate cancer patients’ EMR to automate the determination of risk strata according to the D’Amico Risk Classification, in which prostate cancer patients can be labelled as low, intermediate, or high-risk. The natural language processing (NLP) algorithms developed by Gregg et al.27 had 91% raw agreement with manual risk categorisation, with a recall of 78% (N=1,833). With proper standardisation, such automating procedures for collecting risk characteristics will reduce the time and effort taken, while integrating the information into the respective EHR or disease registries.
Symmans et al.28 have assessed the long-term prognostic risk of breast cancer patients by analysing 1,158 patients’ EMR belonging to five cohorts after neoadjuvant chemotherapy alone or in conjunction with HER-2-targeted treatment. Patient-specific age, classification of primary cancer (whether multifocal or multicentric), clinical and radiologic characteristics of primary cancer, clinical stage of cancer before treatment, diabetic status (present or absent), follow-up for relapse or death, height, and weight data have been considered from the respective EMR for the prognostic study. The study indicates long-term survival after neoadjuvant chemotherapy in all the considered phenotypic subsets of breast cancer, albeit with a need for further external validation.
Heo et al.30 performed a study of 44 advanced biliary tract cancer patients’ EMR to investigate the significance of the clinical impact of overexpression of the proto-oncogene c-MET. Various patient-specific details have been taken from EMR and expression of the c-MET protein has been generated from tumour samples of metastatic patients for this study. In approximately one-third of the patient population, overexpression of c-MET was found, but it cannot be significantly correlated with the other associated variables, generating a need for further studies.
A research study of 99 smokers’ EHR performed by Begnaud et al.22 reflected a randomised electronic promotion of lung cancer screening and Afghahi et al.47 found the differential use of chemotherapy associated with sociodemographic characteristics by a study of EMR, cancer registry, and genomic data belonging to 293 breast cancer patients. In addition, Rioth et al.52 created an automated, real-time database of annotated tumour variants of cancer patients to gather data generated during cancer care for secondary use during the precision decision. Schwaederle et al.33 generated a study using NGS of 439 diverse cancer patients where they integrated the NGS data with age, cancer histology, sex, stage of metastasis at diagnosis (if any), metastatic disease at the time of the biopsy (if any), and race extracted from electronic medical charts, among others, to find actionable aberrations in patients. Lastly, Servant et al.53 developed a knowledge data interface that facilitates data integration and tracks the processing of individual samples for precision decision in real-time.
As EMR and/or EHR are being considered as a global data source for cancer prognosis, their completeness in accumulating all kinds of patient-related data is necessary. At present, there are many cancer resources (Table 1) and their inclusion or association with the EMR will ensure a more detailed cancer prognosis.
Predicting Cancer Risk
EMR, the data flat files for cancer patients, can be subjected to standard statistical analysis techniques.22,28,30,47 Symmans et al.28 used the Kaplan–Meier estimator with 95% confidence intervals estimated using the Greenwood formula with log-log transformation for determination of survival probability. In addition, Heo et al.30 used the t-test, Fisher’s exact test, or one-way analysis of variance, as appropriate, to analyse correlations between expression of the proto-oncogene c-MET and the associated clinicopathologic variables. They used the Kaplan–Meier estimator for the analysis of all time-to-event variables.30 Statistical analysis by Begnaud et al.22 included the Wilcoxon rank sum test for quantitative characteristics and Fisher’s exact test for categorical items, and the multivariable logistic regression technique was used by Afghahi et al.47
EMR data can also be subjected to advanced machine learning techniques for better retrieval of insightful information.27,52 Gregg et al.27 used natural language processing to achieve >90% accuracy for low, intermediate, and high-risk stratification of localised prostate cancer patients. A naïve Bayes classifier was used by Rioth et al.52 to categorise cancer patient care data into 18 separate clinical groups with 99.37% accuracy, and Schwaederle et al.33 used linear or binary logistic regression analyses and Spearman’s rho coefficients. However, application of these statistical and machine learning techniques in one or more cancer resources (Table 1) is yet to be attempted.
Limitations
Precision oncology has many limitations at the preventive, diagnostic, prognostic, and predictive stages.54 Molecular profiling of tumours is not yet used for all types of cancer and many of the molecular alterations observed in patients do not respond to drugs; therefore, more research is required regarding the complete characterisation of viable target biomolecules. Diversity also exists among the types of clinical molecular tests and opinions vary among clinical oncologists regarding the interpretation of genomic test results. These inconsistencies will be reflected in the EHR and will diminish confidence in precision oncology-associated therapies.
Most often, DNA-based alterations, such as mutations and gene duplications, among others, are found to be responsible for the development of a typical subtype of cancer in a patient. A large proportion of EMR lack DNA alteration data of cancer patients, since third-party payers rarely reimburse for mutational analysis, and so forms a major bottleneck for progress in precision oncology. Even when the mutations are available, a great diversity arises when EMR for all patients of a population are referred to as a background study. How to overcome such diversity and reach a consensus for treating a real-time patient is a dilemma for many oncologists.55
Furthermore, EMR are often erroneous or have incomplete data. Knowledge derived from such error-prone or partial data either misleads the oncologists or prevents the achievement of a consensual opinion regarding cancer treatment in general. Even if the EMR are correct, various protocols and sometimes unbalanced treatments across the globe contribute a lot of variation, and a small sample size and heterogeneous patient population may create more confusion. Automated data collection from EMR cannot guarantee the precision level of manual data extraction by experts and the allowable level of precision in automated data collection is a big debate. However, >90% precision is generally considered high. Overfitting of data must be avoided while aiming for higher levels of precision.27
An EMR is a single record of disease instance and, for better cancer care, a properly monitored EHR should be established for a patient. An EHR is an accumulation of multiple EMR over a time period listing the relapse of cancer and retreatment, where applicable; it should list the patient history, family histories, detailed social histories, the testing and imaging results, treatment details, and genomic information to be considered as an ideal cancer EHR.24 However, there is no universal guideline for criteria of an EHR, except a definition of minimum requirement from the World Health Organization (WHO)56 for structuring EMR or EHR.
Moreover, searching for a common factor across related EHR may be a daunting and large task due to a lack of interconnections, mutual compatibility, and interoperability.5 If similar types of EHR cannot be analysed in real time, finding a common clue for a disease will prove difficult. To date, no uniform approach to address all the above-mentioned issues is in place. Altogether, the lack of completeness, consistency, homogeneity, and accuracy of cancer EMR or EHR will reflect in precision oncology-based decisions. Hence, a robust universal format and clear regulations should exist for patient EMR to avoid these caveats and to ensure reliability and efficiency.57,58
In developed countries, maintenance of the EHR is a must for the free centralised government healthcare; a report regarding this has been penned by Ben-Assuli.59 However, in developing and under-developed countries, the maintenance of EHR is performed by third parties, particularly for insurance purposes of the wealthier population who can afford private healthcare facilities. It is often pursued in a very incomplete and sporadic manner. As precision oncotherapies are heavily dependent on population or cohort-based details, EHR data of developed countries may not help for precision oncology-based treatments in other geographic populations. A lack of proper EHR data will delay and hamper the scope of precision oncology in these countries.
EMR-based precision oncology has provided a large amount of introspection and garnered a lot of focus; however, whether it has generated enough confidence for the decisions to be adopted for clinical and therapeutic purposes is doubtful. A selection of scientists and researchers have already expressed their concerns regarding the performance of precision oncology60,61 and there are doubts regarding clinical usefulness of targeted therapies derived from EMR.62 According to these professionals, current precision oncology practices are too simple an approach for a complex disease like cancer. However, there is also another cohort of professionals who strongly believe in it due to a few clinically useful, although unexpected, advances.63,64 Often, the same researchers are found to sway between feeling hope, optimism, and/or concern regarding precision oncology. Whether the concept of precision oncology will be the next groundbreaking landmark innovation of science, or result in a pseudo-innovation, is not clear.65-67 However, the authors’ opinions favour pragmatic optimism with the advent of the new cloud-based software platforms,68,69 novel ways for phenotypic representation70 and stratification,71 multiomics data analytics platforms,72 and big data infrastructures73 for advancement of precision oncology.
CONCLUSION
In this paper, the authors have introduced the emerging area of electronic record (EMR/EHR)-based advances for the improvement of cancer treatment. Moreover, this paper has summarised a few of the recent EMR-based precision oncology practices for prevention, diagnosis, prognosis, prediction, and their associated concerns and limitations.
A cancer patient experiences many emotional and physical hurdles, starting from the initial confirmation and acceptance of their diagnosis. The associated stress, stigma, and sickness looms like a black cloud over the patient. Irrespective of any kind of curative or palliative care, a cancer patient’s life expectancy certainly decreases following diagnosis; some patients have a few days of life or a few years, while others live longer, but always with a fear of tumour recurrence. In these scenarios, even a few scientific details derived from EMR and some unexpected advances that aid in oncotherapy become a baton of hope and belief.
The idea of EMR data-derived precision oncology is novel and the path for achieving this idea is unchartered, while the limitations are many. With the increase of lifestyle addictions and disorders coupled with the genetic and environmental factors, cancer is becoming the second leading cause of global death,74 with 75% of deaths occurring in low and middle-income countries. However, the unbeatable human desire for cancer-free, longer, and healthier lifespans also exists and EMR/EHR will lead the way towards this through precision oncology-based therapies.