Abstract
A major innovation in psychometric science, termed automated item generation (AIG), holds the potential to revolutionise assessment in medical education. In short, AIG involves leveraging the expertise of content specialists, item templates, and computer algorithms to create a variety of item permutations, often resulting in hundreds or thousands of new items based on a single item model. AIG may significantly improve item writing capabilities, reduce human error, streamline efficiencies, and reduce costs for individuals in the medical and health professions. Thus, the purpose of this work is to provide readers with a current overview of AIG and discuss its potential advantages, future possibilities, and current limitations.
AUTOMATED ITEM GENERATION: THE FUTURE OF MEDICAL EDUCATION ASSESSMENT?
Despite many decades of use, multiple-choice questions (MCQ) remain the most commonly used assessment method in medical education. At the undergraduate level, MCQ are routinely administered in classroom assessments due in part to the enormous amount of information students are responsible for learning and the large class sizes that make other assessment methods implausible. At the graduate and postgraduate levels (e.g., medical residency, licensure, and certification), MCQ are regularly used to assess both breadth and depth of ability within a particular medical specialty. General advantages of MCQ are well- documented and include factors such as greater objectivity (scoring is free of judge inconsistencies and bias); greater efficiency (examinee responses can be captured quickly); increased quantity of items (more questions result in smaller error estimates and more reliable scores); increased range of content (broad representation of content provides a more accurate estimate of ability); and a variety of item statistics that help discern the psychometric quality of the items.
Despite these important advantages, MCQ often present several major implementation challenges, namely time, difficulty, expense, and security. Constructing MCQ is time-consuming; the process typically involves constructing each item by hand, reviewing the item, editing the item, and entering the item into a computer.1 Constructing MCQ is also difficult and research has noted that item writers often have difficulty generating plausible distractors,2 writing items to a specified difficulty level,1 and are subject to committing item writing flaws.3 In fact, one study investigating the quality of items administered at a major medical school in the USA found as many as one in five items contained an item construction flaw.4 Costs associated with constructing MCQ are also quite severe. In addition to time and opportunity costs, Rudner5 estimates the monetary cost associated with a single item approximates from $1,500–2,500. Finally, given the high stakes associated with medical education assessments and the pressures to perform well, some unscrupulous individuals will attempt to harvest items, thus rendering them ineffective for all future examinations.6,7
Each of the aforementioned limitations, coupled with increased demands for new and more numerous items for both high-stakes examinations and practice tests, have made it difficult for the field of medical education to keep pace with current demands. Fortunately, a major breakthrough in psychometric science, called automated item generation (AIG), holds the potential to overcome many of the weaknesses and challenges associated with MCQ. Thus, the purpose of this work is to provide an overview of AIG and discuss its potential implications for the field of medical education.
OVERVIEW OF AUTOMATED ITEM GENERATION
Broadly defined, AIG is the process of using item models to create examination items with the assistance of computer technology.8 Unlike the typical item generation process in which a content specialist constructs each item individually, AIG involves leveraging the expertise of content specialists, item templates, and computer algorithms to create a variety of item permutations, often resulting in hundreds or thousands of new items based on a single item model.9 AIG is considered both an art and a science, as developing an examination requires human judgment and expertise (art) and computing technology systematically combines large amounts of information to generate new items (science).
Automated Item Generation Process
The AIG process involves three steps: first, content experts create a cognitive map by identifying the content necessary for inclusion in an examination item; second, content experts develop an item model (or template) for the content; finally, a computer algorithm combines various elements of content to generate items. New items can be classified as either a ‘clone’ or a ‘variant’, where cloned items appear very similar and will possess only subtle differences with comparable psychometric properties, whereas a variant will vary in some more discernible way and likely possess different psychometric characteristics (Figure 1).
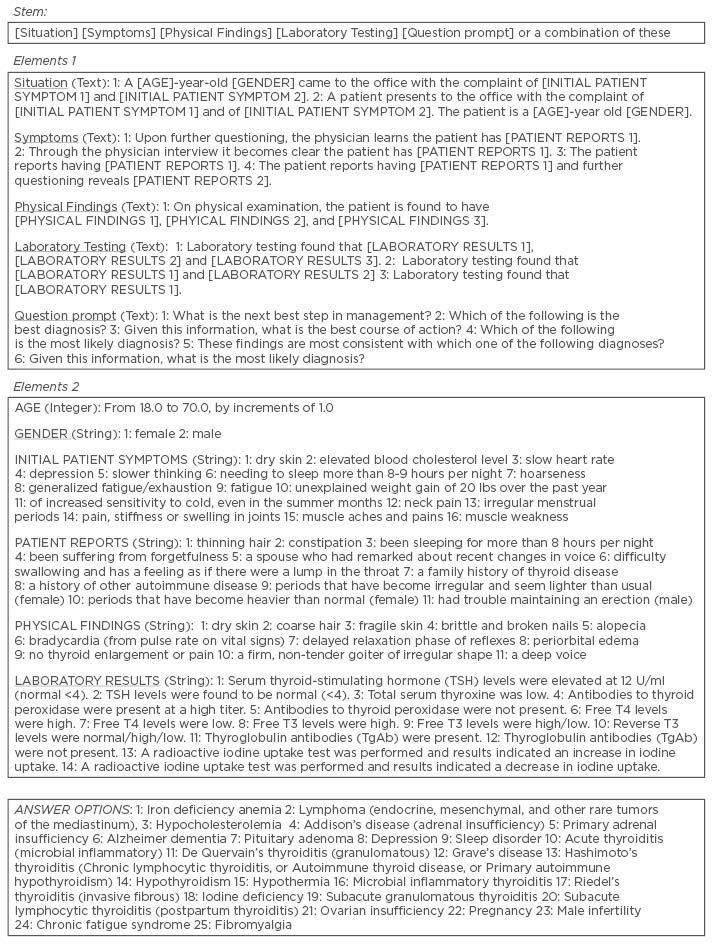
Figure 1: Sample automated item generation criteria.
Sample Items Generated to Assess One’s Ability to Diagnose Hypothyroidism
Using the criteria in Figure 1, we have used AIG to generate four sample items to assess one’s ability to diagnose hypothyroidism.
#1: A 30-year-old female came to the office with the complaint of unexplained weight gain of 20 lbs over the past year and of increased sensitivity to cold, even in the summer months. Upon further questioning, the physician learns the patient has periods that have become irregular and seem lighter than usual. On physical examination, the patient is found to have dry skin, delayed relaxation phase of reflexes, and a firm, non-tender goiter of irregular shape. Given this information, what is the most likely diagnosis?
A. Adrenal insufficiency
B. Depression
C. Hypothyroidism*
D. Iron deficiency anemia
*Correct answer option
#2: A 36-year-old female came to the office with the complaint of dry skin and fatigue. The patient reports that her spouse had remarked about recent changes in her voice and further questioning reveals a family history of thyroid disease. On physical examination, the patient is found to have coarse hair, bradycardia, and delayed relaxation phase of reflexes. Laboratory testing found that TSH levels were elevated at 12 U/ml (normal < 4), total serum thyroxine was low, and antibodies to thyroid peroxidase were present at a high titer.
Which of the following is the most likely diagnosis?
A. Acute thyroiditis
B. Hashimoto’s thyroiditis*
C. Iodine deficiency
D. Pituitary adenoma
*Correct answer option
#3: A 28-year-old female came to the office with the complaint of generalised fatigue/exhaustion and irregular menstrual periods. The patient reports having difficulty swallowing and has a feeling as if there were a lump in the throat. On physical examination, the patient is found to have fragile skin, brittle and broken nails, and no thyroid enlargement or pain. Given this information, what is the most likely diagnosis?
A. Adrenal insufficiency
B. Fibromyalgia
C. Hypothyroidism*
D. Ovarian insufficiency
*Correct answer option
#4: A patient presents to the office with the complaint of needing to sleep more than 8-9 hours per night and fatigue. The patient is a 35-year-old female. The patient reports having constipation. On physical examination, the patient is found to have bradycardia, delayed relaxation phase of reflexes, and periorbital oedema. Given this information, what is the most likely diagnosis?
A. Adrenal insufficiency
B. Depression
C. Hypothyroidism*
D. Sleep disorder
*Correct answer option
ADVANTAGES OF AUTOMATED ITEM GENERATION
Perhaps the most obvious advantage of AIG is its ability to quickly produce thousands of new items. This strength is particularly advantageous in scenarios such as medical licensure and certification, in which the organisation must maintain thousands of updated, high-quality items at all times. A common problem for most organisations, including medical schools, is that item banks often possess shallow pools in certain content areas. AIG can be particularly helpful in this situation by populating notoriously sparse content areas.
Another major advantage of AIG is that items can be targeted based on known difficulty estimates and reproduced with ‘clones’ to generate new, yet different, items.10 Similarly, if an examination developer discovers an examination contains too many easy or difficult items, AIG can help populate the item bank with ‘variants’ to improve item targeting (e.g., ensuring items are appropriately easy or difficult relative to the ability of the collective sample frame).
In the context of medical licensure and certification, it is common practice for examination committees to construct new items, review others’ items, and enter items into the item bank for operational use. The use of AIG can help examination committees shift their focus from creating new items to evaluating new items and providing quality assurance efforts. This change in functional duties could result in the exponential increase of high-quality items produced in the same amount of time.
In the context of medical schools, most faculty members have little to no formal training in item construction, yet are expected to produce their own high-quality items. AIG could also prove invaluable for these individuals. Research4 has also noted that the most common item construction flaws involve poor item formatting and structures (e.g., unequal distractor length, unfocussed stem, use of negative statements, etc.). Because AIG use standardised templates for constructing each item, it could help the faculty avoid these common mistakes and result in more standardised and robust items for students.
FUTURE POSSIBILITIES
The medical and health professions offer numerous opportunities for AIG to be applied and evaluated. For example, there are numerous types of assessment beyond MCQ, such as objectively structured clinical examinations, simulations, live-patient examinations, oral examinations, mannequin examinations, and more. Furthermore, there are multiple levels of medical education, including undergraduate, graduate, postgraduate, and continuing medical education. At present, we are unaware of anyone who has used AIG in any assessment context beyond MCQ, but such use is certainly possible. We are also only aware of <5 organisations in medical licensure and certification that have trialled AIG, thus further evidencing the room for growth and development of AIG.
While none of the authors of this paper claim to have any prognosticating abilities, we can envision several ways in which AIG may be used in both medical education and clinical medicine. First, licensing and certification boards spend a large sum of money training physicians to write high quality MCQ. Even after physicians are trained and items developed, professional editors must still review the newly generated items to identify any flaws and ensure standardisation in format. It is possible that AIG can mitigate many editorial duties for both physicians and professional editors and significantly improve efficiencies by having content experts focus almost entirely on content creation and review.
Secondly, there is currently a significant focus on maintenance of certification (MOC) and medical recertification. Most MOC efforts require physicians to complete a battery of practise cases for ongoing professional development purposes. Given MOC cases and practise items are similarly expensive to produce, AIG could significantly reduce costs and promote efficiencies. In the context of medical school training, perhaps even greater possibilities exist.
For academic staff, item writing flaws could significantly reduce resulting in items that are more likely to yield accurate estimates of what students know or can do. Furthermore, if the staff desire to have more clinically based items in their item bank, AIG can help with its use of a standardised template. Faculty members could then spend more time reviewing and editing items, as opposed to generating new items from scratch. With respect to classifying items into content domains, AIG can likely also improve this process.
Through the use of an established model, such as Bloom’s taxonomy, the faculty could create a variety of templates to assess learning from a variety of cognitive approaches. For example, Bloom’s taxonomy identifies the following domains: Know, Comprehend, Apply, Analyze, Synthesize, and Evaluate. Staff could structure item templates to address each of these domains and provide greater balance in domain assessment should they choose.
Students also have much to gain from AIG, as a virtually unlimited item bank could provide students with endless opportunities to learn and self-assess. Automated items could also be provided to students as part of virtually any course for continuous and long-term study extending months or even years later. Additionally, AIG has the potential to revolutionise the post-examination review process. Several assessment experts have noted that reviewing secured examination items during post-examination review sessions could increase the odds of items being leaked, thus affecting the validity of future scores. If students were presented unsecured items on related content, it could achieve the goal of reviewing substantive content without sacrificing potential item loss.
CURRENT LIMITATIONS
Perhaps the greatest limitation of AIG today is that it remains a budding, albeit potentially revolutionary, science. Before a paradigm can become an established science, it must be scrutinised, thoroughly tested, and become well understood. Although scholars have worked on the foundations of AIG for decades, the AIG paradigm has yet to take hold in most areas of research and practice. While there are many potential reasons for this, perhaps the greatest is the limited availability of software and a reluctance to share it from those who do have access. Clearly, AIG science cannot grow and be tested if others cannot test AIG for themselves and contribute toward new discoveries. Assuming the scientific community fully embraces AIG, the next challenge will be to extend AIG into everyday practice in a variety of settings. It is likely, however, that the field of medicine will be among the many potential first adopters of AIG, given the need for continuous, rigorous assessment of students and practicing healthcare professionals alike.
One major limitation of AIG involves ill-structured problems.11 AIG appears to work effectively with well-structured problems, such as clinical vignettes in which there are multiple replacement characteristics to generate a variety of vignettes and a single correct answer. However, an instance in which a problem is ill-structured becomes much more problematic. For example, a problem may be inadequately defined, have many correct answers, lack background information, or its scope may be broader than a single item can capably assess.8 In these instances, AIG will suffer from the same limitations as ill-structured items prepared by traditional means.
Another potential limitation pertains to distractor quality. AIG selects potential distractors based upon information entered into an algorithm, thus there is a strong possibility that many of the newly generated distractors may be problematic. For example, many distractors may be implausible, irrelevant, and/or factually incorrect. Depending upon the information used to generate distractors, some output may be entirely unintelligible. Furthermore, because of the manner in which items are generated, there is a risk that item quality may be highly variable. While it is true that AIG may produce hundreds of items based on a single case or scenario, it remains unclear what proportion of newly generated items and distractors are typically of sufficient quality or worthy of use. As noted previously, the need for human discernment is an inescapable element of AIG, thus any benefit gained from producing additional items will be at the costs of additional time spent conducting quality assurance activities. Naturally, all automated items will still need to undergo review for substance, clarity, and appropriateness to minimise item flaws.
Finally, the cost-benefit economics of AIG technology have not yet been thoroughly evaluated or reported. In theory, AIG has the potential to save exorbitant amounts of time for item writers, which may include subject matter experts who provide items for board examinations and medical school departments charged with the task of teaching and assessing students’ learning. Increased time savings could allow item writers to focus their energies in other areas and potentially result in greater achievement of outcomes, such as student learning.
It remains unclear how steep any learning curves may be for item writers to use AIG technology. It is unknown how difficult the typical subject matter expert will find the process of writing cases and preparing content to fit a structured item model. It is also unknown how subject matter experts will respond to AIG software, particularly if it is something they can do themselves or if it will require the assistance of an information technology specialist.
CONCLUSION
The purpose of this review aimed to familiarise readers with AIG and promote interest in this exciting, and potentially revolutionary, innovation in medical education. Although much is currently unknown about AIG in practice, extant research from those who have used it is assuring. While the future of AIG is sure to encounter some turbulence as early adopters become acquainted with this new science and explore its possibilities, its long-term prospects for improving the way in which students are assessed is very bright.