Meeting Summary
This symposium was held on 20th October 2019 during the United European Gastroenterology (UEG) Week in Barcelona, Spain, where a gathering of global opinion leaders in gastroenterology took place to discuss advances in the field of early detection of colorectal lesions with artificial intelligence (AI) technology. Updated information was presented on the role of AI in identifying and recognising polyps during colonoscopy. A summary of the symposium is presented below.
Early detection of colorectal cancer (CRC) in gastroenterology through national screening offers patients the best opportunity for disease-free and overall survival,1 but there is still a large variation in lesion detection via colonoscopy.2,3 The adenoma detection rate (ADR) is the gold standard when it comes to evaluating the quality of the endoscopist in screening for CRC.4 Improved ADR is driven by imaging technology, withdrawal technique, endoscopist knowledge and motivation, as well as environmental and social factors.5,6
Using computer vision for lesion detection will be the first application of AI in gastroenterology and will help to improve the quality of endoscopy practice in finding and recognising polyps on colonoscopy. The GI Genius™ intelligent endoscopy system (Medtronic Inc., Minneapolis, Minnesota, USA) is an AI-enabled endoscopy software that detects mucosal abnormalities during colonoscopy in real-time. It is the first global application of AI for endoscopists, alerting their practice via a visual marker and an optional acoustic alert.
GI Genius shows impressive detection when used to view videos of both good and bad bowel preparation, aligning with the observation patterns of the endoscopy specialist using the system. AI applications such as the GI Genius can lead to improved ADR, providing earlier detection of CRC and better clinical management of lesion and tissue characterisation, making the diagnosis and management process easier for the clinician.
Introduction
CRC is the third most common form of cancer globally, with 1.8 million new cases diagnosed every year. It is the second highest cause of cancer mortality worldwide.7 More than 90% of colorectal carcinomas are adenocarcinomas, which can be detected using colonoscopy screening procedures.8
Although colonoscopy is the established method for CRC screening, it is subject to large variations in quality.9,10 The performance benchmark for ADR is currently ≥25% for all patients.11 Improved ADR are directly correlated with CRC detection; each 1% increase in the ADR is associated with a 3% decrease in CRC risk.4,12 However, the ADR can vary greatly between endoscopists, and a second observer may also help to increase polyp detection rates.9,10
Each 1% increase in the ADR is associated with a 3% decrease in the risk of cancer.
Artificial Intelligence for Colonoscopy
AI in the field of endoscopy largely relates to computer vision technology, which allows computers to ‘see’ and interpret visual content. Through processes of machine learning, and more recently deep learning, AI systems can be trained to recognise ‘normal’ characteristics by linking a gold standard to suitable images.
Initial AI development relies on creating an algorithm that uses highly accurate datasets, coded in an independently staged manner by a group of specialists. This process is called the ‘ground-truth’ setting, where the computer is trained to distinguish between ‘abnormal’ and ‘normal’ tissue. Once an algorithm is created, deep convoluted neural networks use vast datasets to enable the algorithm to become more intelligent. This intelligence can extend to the algorithm becoming agnostic to manual data input and it can learn unsupervised by developing its own rules and classifiers.13
The intelligent GI Genius system uses the principles of computer vision and deep learning. GI Genius is the world’s first application of AI during endoscopy, using advanced AI to highlight potential precancerous lesions. It analyses the video stream in real time and superimposes a frame over any potential lesions, including those with flat (nonpolypoid) morphology. GI Genius therefore acts as a virtual second observer to assist the endoscopist in detecting lesions and abnormalities during endoscopic screening. The GI Genius module consists of two parts; the module itself is a small box that seamlessly integrates with existing colonoscopy equipment and therefore the physician does not have to change standard colonoscopy procedures, while the polyp detection software uses a validated AI algorithm. The endoscopic exam also remains the same for the patient. Since the first use-case in June 2019, where Prof Alessandro Repici successfully demonstrated the GI Genius technology to detect sessile serrated adenoma/polyps during colonoscopy, the validation of GI Genius has continued to grow in Europe. GI Genius was Conformité Européenne (CE)-marked in Europe in 2018.14
The GI Genius module works in real time, automatically identifying and marking abnormalities consistent with colorectal polyps, including those with flat (nonpolypoid) morphology.
Validation of the GI Genius System
In 2013, a study by Repici et al.15 investigated the efficacy of per-oral methylene blue formulation in colonoscopy.15 During the study, all colonoscopies were digitally recorded in high definition without any loss in quality. All data were stored on local drives and backed up on cloud storage. All polypoid and nonpolypoid lesions were digitally annotated with a high degree of accuracy.
The next step was to train the AI deep learning system. Every frame of each polyp was annotated, leading to the creation of a mathematical matrix for polyp identification. The first image dataset used for AI algorithm development (Quarter 1, 2017) consisted of 500 ‘easy to detect’ polyps. These represented optimal AI learning conditions with no endoscopist bias, consecutive patient enrolment (unbiased distribution), and endoscopists with a high ADR (47%). The dataset was then expanded in Quarter 2 of 2018 with an additional 5,000 images added from the per-oral methylene blue formulation clinical study. An improved annotation procedure was created during this second addition, which improved the quality of the dataset. By the time the dataset size was frozen in September 2018 to secure regulatory approval, it consisted of more than 1.5 million images.
On database validation, GI Genius was found to be robust and unaffected by size, morphology, location, and histology of lesions (Figure 1).16 The false positive rate was also assessed in a per-frame analysis. Activation noise (false positive frames in a full procedure) was 0.9%, with no detrimental effect on withdrawal time or standard clinical practice. To minimise the impact of bias in this study, the team assessed whether the computer was faster versus a panel of five expert endoscopists.
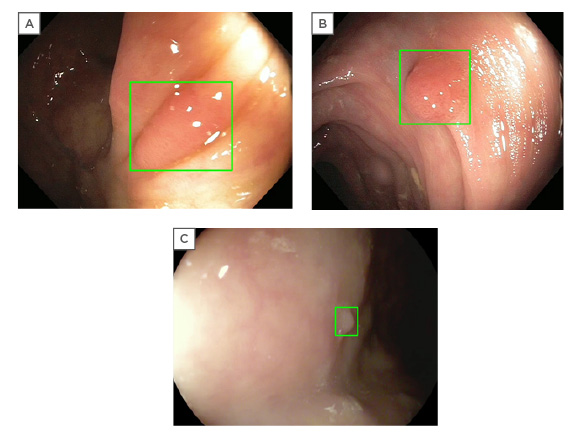
Figure 1: Examples of GI Genius™ intelligent endoscopy system (Medtronic Inc., Minneapolis, Minnesota, USA) imaging detection (GI Genius abnormality notification is displayed as a green box around the suspect area).16
(A) II-a adenoma. (B) I-s adenoma. (C) II-a hyperplastic polyp.
The GI Genius detected polyps 1.27 seconds earlier versus than the endoscopists 82% of the time.16
Polyp recognition does not always equate to polyp detection. Effective polyp detection is associated with a multitude of other factors including withdrawal time, degree of mucosal exposure, level of bowel cleansing, disease prevalence, auxiliary manoeuvres, angle of view, and the skill of the endoscopist. Polyp detection should be focussed on the accurate detection of lesions for cancer prevention.
A key strength of the GI Genius during diagnostic colonoscopy is that it brings the endoscopist into a learning community and helps to improve their diagnostic accuracy. The GI Genius system is accurate because it is objective; it can detect more than one polyp, is not distracted or side-tracked, and also tends to be faster than manual human identification. Routine use of GI Genius can make life much easier for the endoscopist, allowing faster and more accurate lesion detection.
With endoscopist competence, passion, and dedication, GI Genius can be exploited at its maximum level, making it a great device.
Artificial Intelligence and Quality Issues for Colonoscopy
AI will change the way that performance and quality improvement in colonoscopy is managed, with the potential to improve a number of key performance measures (KPM) (Figure 2).17 One of the first applications of AI in KPM improvement would be to qualify the adequacy of bowel preparation. There are currently no consistent methods to capture this, but an AI system could provide endoscopists with the facility to automatically grade bowel preparation against a scale measure, and identify those of poor quality. Another application would be to capture the completeness of colonoscopy; an AI system could recognise caecal intubation and immediately capture this into datasets.
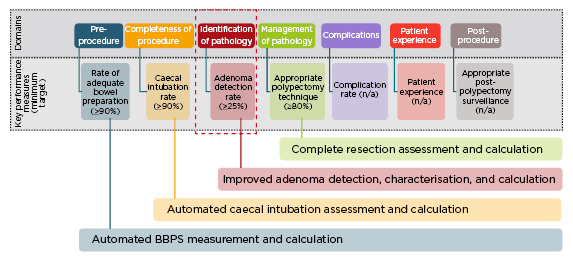
Figure 2: Key performance measures for colonoscopy: potential role of artificial intelligence.17
BBPS: Boston Bowel Preparation Scale.
This is in contrast with the current manual recording method of KPM which is cumbersome and sometimes inaccurate.
The success of a colonoscopy depends on many variables such as the ADR of the endoscopist, the time of day, fatigue of the physician, the equipment used, or the patient population.18 The endoscopist’s ADR is inversely associated with the risk of interval CRC (development of CRC following colonoscopy) and CRC death;3,12 improving ADR can therefore reduce both CRC and mortality risk.19,20 ADR is driven by a number of factors including imaging technology, withdrawal technique, knowledge and motivation, as well as environmental and social factors.5,6 In addition to improving image recognition and supporting endoscopists in real time to improve their technique, AI can help to understand or improve the quality of withdrawal technique by providing real-time feedback to the endoscopist on how they are performing.19,21-29 AI can also improve ADR by supporting assessment of environmental and social factors and providing feedback for improvements.
For AI to be of value to endoscopists, it should seamlessly integrate into any existing endoscopy stack, be accurate in lesion detection and tissue characterisation, increase ADR, not distract the endoscopist whilst it deploys its technology, and create limited false positives. The first iteration of GI Genius successfully meets these criteria.
When AI systems for polyp detection are evaluated, the examiner must ensure that endoscopy times are equal to standard colonoscopy; the temptation in AI-based systems is to increase endoscopy time by spending more time looking for polyps. This can create bias favouring AI-based endoscopy. Wang et al.30 randomised 536 patients to standard colonoscopy and 536 to colonoscopy with computer-aided diagnosis (not the GI Genius). ADR increased significantly with computer-aided diagnosis (29.1% versus 20.3%; p<0.001) and adenoma rate per colonoscopy was also significantly higher with AI (0.53 versus 0.31; p<0.001). However, this was found to be attributed to the higher numbers of diminutive adenomas being found (≤5 mm), with no statistical difference in larger adenoma detection. A concomitant increase in the rate of hyperplastic polyps was also seen in the study, which should be taken into consideration.
Technologies that increase ADR (EndoCap, chromoendoscopy, and AI) tend to create higher false positives. The ratio between ADR and histological confirmation of adenoma should therefore be greater than one. AI-identified lesions should be validated by histopathology during training, though the level of validation can vary. This approach can help to recognise subtle differences between lesions, and although challenging, can considerably improve AI efficiency. To conclude, AI can support improvements in colonoscopy quality and ADR, and has enormous potential in facilitating ADR increase and/or general quality improvement in endoscopy.
EARLY EXPERIENCE WITH GI GENIUS IN CLINICAL PRACTICE
When a pedunculated or sessile polyp lesion is detected, GI Genius alerts the physician with an on-screen marker. This allows them to focus on this region of the intestine, set markers in the mucosa, take a tissue sample, or remove an abnormal section completely.
Prof Bhandari has used and assessed the GI Genius stringently and critically. His real-world experience of GI Genius in clinical practice demonstrated that the system had a comparable speed to his manual technique as the testing endoscopy specialist. Notably, GI Genius also demonstrated similar recognition patterns, spotting the same areas of potential abnormality on colonoscopy then reassessing the potential abnormality on closer viewing. GI Genius was able to highlight any unusual area in the bowel that may have warranted further investigation, which is valuable to the endoscopist as any uncertain areas detected should still be biopsied.
“…it (GI Genius) is thinking like us (the endoscopist),” commented Prof Bhandari.
He also reported that the GI Genius was able to detect abnormalities even in bowel that was poorly prepared and covered in stool, showing good consistency of detection, which is extremely valuable in real-world practice. Poorly prepared bowel can make lesion detection more challenging. Prof Bhandari discussed the nuisance of air bubbles in the colon during colonoscopy, which can sometimes interfere with detection, creating false positives. However, the GI Genius did not appear to be affected in the case presented, where many bubbles were present. Even with water flushing, presenting the challenge of water submerged views (which include complicating factors such as bubbles, quick withdrawal, and sudden movements), GI Genius still identified abnormalities accurately. These cases demonstrate the value of GI Genius in identifying unusual areas even when factoring in real-world practice and difficult manoeuvres during endoscopy.
The GI Genius module uses advanced AI to highlight the presence of precancerous lesions with a visual marker in real time, providing a reliable second observer for the endoscopist.
GI Genius therefore shows impressive detection abilities when used to view videos of both good and badly prepared bowel. This has the potential to change clinical practice and could influence how bad bowel preparation is managed, as GI Genius can highlight abnormalities even with poor preparation. Even a false positive result calls attention to the mucosa and helps the endoscopist to hone their detection technique. AI can be used to recognise key landmarks which can provide endoscopists with better navigation tools during challenging cases. Prof Bhandari concluded that the GI Genius technology is promising, and able to find polyps at the same time as, or faster than, the human eye. There is continuing scope for improvement, but it is ready for use in clinical practice.
Conclusion
The GI Genius intelligent endoscopy module is the first system worldwide to utilise AI in the field of gastroenterology. It has supported the physician during the endoscopic exam and has demonstrated detection sensitivity, both in a retrospective clinical study16,29 and in real-world clinical practice. There is no substitute for good endoscopy practice and ADR, but GI Genius can act as a virtual second observer to improve ADR and reduce a patient’s risk of CRC developing undetected.
It is important to consider whether advances in AI will lead to endoscopists becoming complacent and less trained in detecting lesions. The faculty’s view was that AI acts as a valuable supporting tool for gastroenterologists and will not remove the need for the clinician’s input. Gastroenterologists do a great deal in addition to endoscopy; they decide on who needs a colonoscopy, advise on bowel preparation, integrate patient factors, and carry out correct scope insertion, correct detection, and classification. While AI is likely to show advances in classification, detection, and assistance with polyp removal, gastroenterologists will still need to provide therapeutic support. AI has potential to standardise performance across centres and reduce the wide variability in endoscopist’s performance.
AI in colonoscopy is a new field with a new curriculum, but it has the ability to improve the quality of endoscopy and patient care. AI in endoscopy currently provides both effective lesion detection and a valuable learning platform for the endoscopist. The future is finally here.