Abstract
Background: Severe COVID-19 has been associated with certain pre-existing health conditions and can cause respiratory failure along with other multi-organ injuries. However, the mechanism of these relationships is unclear and prognostic biomarkers for the disease and its systemic complications are lacking. This study aimed to examine the plasma protein profile of patients with COVID-19 and evaluate overlapping protein modules with biomarkers of common comorbidities.
Method: Blood samples were collected from COVID-19 cases (n=306) and negative controls (n=78) among patients with acute respiratory distress. Proteins were measured by proximity extension assay utilising next-generation sequencing technology. Its associations to COVID-19 disease characteristics were compared to that of pre-existing conditions and established biomarkers for myocardial infarction, stroke, hypertension, diabetes, smoking, and chronic kidney disease.
Results: Several proteins were differentially expressed in COVID-19, including multiple pro-inflammatory cytokines such as interferon-γ, CXCL10, and CCL7/MCP-3. Elevated IL-6 was associated with increased severity, while baseline IL1RL1/ST2 levels were likely associated with a worse prognosis (p<5×10-5). Network analysis identified several protein modules associated with COVID-19 disease characteristics overlapping with processes of pre-existing hypertension and impaired kidney function. B-type natriuretic peptide and N-terminal pro-B-type natriuretic peptide, markers for myocardial infarction and stroke, increased with disease progression and were positively associated with severity. MMP12 was similarly elevated and has been previously linked to smoking and inflammation in emphysema, along with increased cardiovascular disease risk.
Conclusion: This study provides an overview of the systemic effects of COVID-19 and candidate biomarkers for clinical assessment of disease progression and the risk of systemic complications.
INTRODUCTION
The recent COVID-19 pandemic is caused by the severe acute respiratory syndrome coronavirus 2 (SARS-CoV-2) and, since its identification in late 2019, the virus has spread worldwide with >30 million reported cases.1 Clinical presentation can range from mild flu-like symptoms, including fever, cough, and shortness of breath, to acute respiratory distress syndrome (ARDS) requiring mechanical ventilation.2-4 Disease severity and mortality rate have been associated with certain pre-existing conditions such as a history of cardiovascular disease, hypertension, diabetes, and obesity, which correspond with a worse prognosis.4-7 These conditions often accompany older age and likely explain the higher mortality rate observed among the elderly population.4 Recent genetic studies indicate the potential protective effect of specific blood antigens and possibly polymorphisms within the angiotensin-converting enzyme 2 (ACE2) gene,8,9 the primary cell entry receptor for SARS-CoV-2.10
Although clinical manifestations are mainly respiratory, early clinical reports and extrapolation from similar coronaviruses11 (e.g., SARS-CoV-1 and Middle East respiratory syndrome coronavirus) have detailed the systemic effects of COVID-19, including acute cardiac injury, heart failure, arrhythmia, gastrointestinal distress, impaired liver function, and acute kidney injury.4,7,11-13 Thromboembolic complications are common among patients with pre-existing cardiac and cerebrovascular diseases, which is likely related to the systemic inflammation and pro-coagulatory conditions from COVID-19 infection.14,15 Early surveillance studies have also reported neurological manifestations, including altered mental status and impaired consciousness along with fatigue, pain, and sensory disturbances (e.g., anosmia, dysgeusia) post-recovery;16,17 however, the long-term complications of COVID-19 remain unclear.
While the clinical characteristics of COVID-19 are continually refined in real-time, more efficient tools, particularly prognostic markers, are needed to evaluate disease progression for targeted intervention strategies and to better understand the overlapping systemic pathology between SARS-CoV-2 infection and comorbidities. Using high-sensitivity proximity extension technology, this study examined the blood proteome of patients with COVID-19 for protein markers associated with early infection and disease prognosis, and compared them with known biomarkers of common pre-existing conditions and related complications.
METHODS
Adult patients (n=384) presenting with acute respiratory distress were investigated at Massachusetts General Hospital, Boston, USA, of which 306 patients tested positive for COVID-19 while 78 remained as negative controls.18 The descriptive statistics of the cohort are provided in Table 1. Longitudinal blood sampling was conducted for cases at 3, 7, and 28 days from baseline, if possible. Pre-existing conditions, including heart (e.g., coronary artery disease, congestive heart failure, valvular disease), lung (e.g., asthma, chronic obstructive pulmonary disease, regular oxygen use), and kidney (e.g., chronic kidney disease, baseline creatinine >1.5 mg/dL) diseases, were recorded along with any history of diabetes, hypertension, and any immunocompromising conditions. Characterisation of obesity was defined as a BMI of ≥30 kg/m2. Each patient’s condition was assessed using a 6-point ordinal scale (1: death; 2: intubation and mechanical ventilation; 3: non-invasive ventilation or high-flow oxygen; 4: hospitalised with supplementary oxygen; 5: hospitalised without supplementary oxygen; and 6: not hospitalised) based on World Health Organization (WHO) guidelines.19
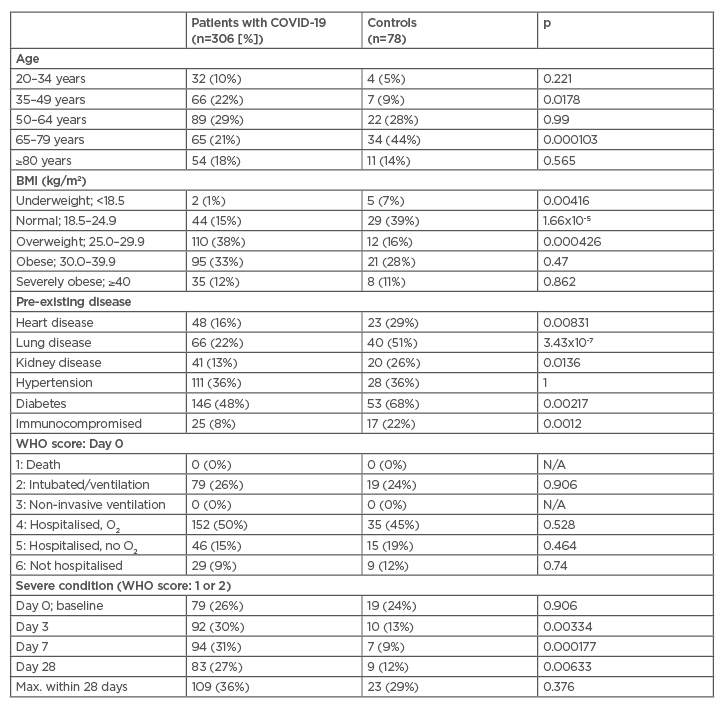
Table 1: Descriptive statistics of the cohort.
Frequency and proportion are reported. Differences between cases and controls were assessed using a chi-square test. WHO-based 6-point ordinal scale was used to categorise severity of patient condition: 1=death, 2=intubation and mechanical ventilation, 3=non-invasive ventilation or high-flow oxygen, 4=hospitalised with supplementary oxygen, 5=hospitalized without supplementary oxygen, and 6=not hospitalised. Severe condition was categorised as WHO score of ≥2.
O2: oxygen; Max.: maximum; N/A: not applicable; WHO: World Health Organization.
Proteins were measured in plasma using a proximity extension assay, a high-sensitivity multiplex immunoassay that utilises paired oligonucleotide antibody probes for protein identification, followed by quantification using quantitative polymerase chain reaction or next-generation sequencing (NGS) technology.20 In this study, samples were analysed with the NGS-based Olink® Explore (Olink, Uppsala, Sweden) product, consisting of four 384-plex panels of 1,536 assay probes, including 48 controls and three inter-panel quality control markers (IL-6, IL-8, and TNF).21 The relative concentration for each protein was quantified as log base-two normalised protein expression levels. Internal assay controls were used to quality check each step of the assay (i.e., incubation, extension, and amplification), and sample measures with high variability were excluded. Additional details regarding the method have been described elsewhere.20,21
In summary, 1,420 unique proteins were analysed, with the majority having a call rate (i.e., measurable levels above the limit of detection) >80%. Measures <25% (x=160) were excluded, while those between 25% and 80% (x=229) remained in the analysis but were interpreted with precaution. In total, 1,260 proteins passed quality control for the analysis.
Differences in protein levels between patients who tested positive for COVID-19 and negative controls were analysed using a multivariable linear regression model, adjusting for age and pre-existing conditions (Table 1). Longitudinal changes in protein concentrations were analysed using a paired Student t-test. Pre-analytical variation associated with sample quality was assessed and corrected using previously defined markers of sample handling (e.g., CD40L).22 Association with severity and prognosis was assessed using the baseline severity score or maximum-reached severity score within the 28-day period, respectively. Scores for severity and prognosis were dichotomised based on the usage of mechanical ventilation or death (severe: 1-2, non-severe: 3-6; WHO score). Significance after Bonferroni correction for multiple testing was set at conservative cut-off of p<10-5, although suggestive associations of p<5×10-4 were considered. All statistical analyses were conducted using R v.4.0.2 (R Core Team, Vienna, Austria).
Modules or clusters of proteins were identified by weighted correlation network analysis using a Pearson-based weighted adjacency matrix (signed, β=14) and average linkage hierarchical clustering.23 Modules were evaluated for enriched biological processes24 and used to compare study associations with the differential protein profiles of other diseases including myocardial infarction,25-27 cardiovascular-related death/heart failure,27 stroke,27,28 hypertension,29 atherosclerosis,30 diabetes,31 smoking,32 kidney function,33 and chronic kidney disease.33
RESULTS
Many proteins were differentially expressed among patients with COVID-19 compared to negative controls, as shown in Figure 1. Inflammatory cytokines such as CXCL10, CXCL11, and interferon-γ increased four-fold at initial sampling but then decreased during the follow-up period. In contrast, lower levels were detected for CDON, ROR1, and BOC but likewise stabilised and regressed within the first week. However, several proteins, including ITGA11, continued to decrease over time. A delayed effect was observed with SDC1, PTN, and SFRP1, which were not initially associated with the disease but increased gradually as the disease progressed. Similarly, levels of ACE2 increased over two-fold within the first week but were not elevated at baseline (β=-0.06, p=0.65).
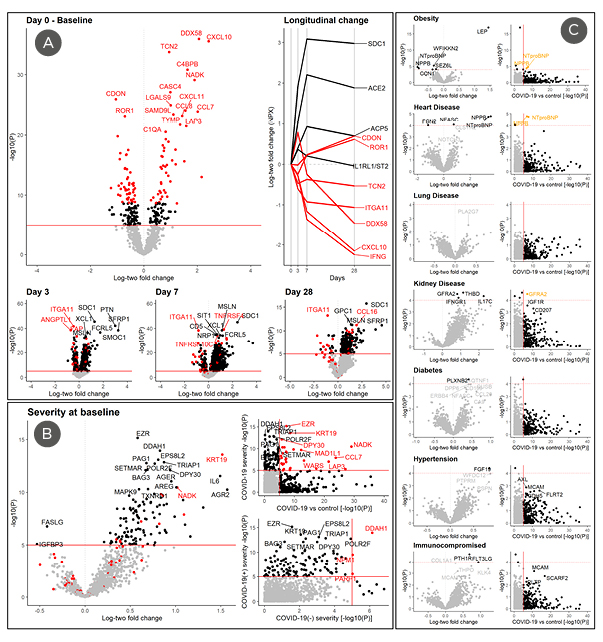
Figure 1: Proteins associated with COVID-19 disease onset, severity, and pre-existing conditions at baseline.
A) Differences in log base-two protein levels (NPX) between COVID-19-positive cases (n=305) and -negative controls (n=78) were analysed using a multivariable linear regression model adjusting for age, pre-existing conditions (Table 1), and sample handling. The top 100 COVID-19-associated markers are labelled in red for all plots. Changes in protein levels at 3, 7, and 28 days compared to baseline among cases were analysed using a paired Student t-test (n=211, 131, and 40, respectively). Levels relative to baseline are also plotted longitudinally for those followed till Day 28 (n=40) to limit potential survivorship bias. B) Association between protein levels and severity at baseline was determined using a multivariable linear regression model adjusting for age, pre-existing conditions, and sample handling. Only DCN, S100P, and IL1RL1 was suggestively associated with prognosis (p<5×10-5) after adjusting for baseline severity (not shown). Both severity and prognosis were defined as a WHO score of ≥2 (i.e., death or use of mechanical ventilation) at baseline or within 28 days, respectively. C) Association to pre-existing conditions including obesity (≥30kg/m2), heart/lung/kidney disease, diabetes, hypertension, and any immunocompromising conditions are illustrated above (left) in comparison to their association with COVID-19 (right). Cases with pre-existing conditions were compared to those with no pre-existing condition (n=89) using a multivariable linear regression model, adjusting for age and other pre-existing conditions.
NPX: normalised protein expression; vs: versus; WHO: World Health Organization.
Findings were not significantly affected after correcting for sample handling, although there was a minor improvement in the variability. The majority remained significant after correcting for pre-existing conditions and obesity. However, a few proteins, including IL1R2, a protein upregulated in the adipose tissue of patients with obesity,34 were only associated after stratification by BMI (normal: β<0.001; p=0.99; obese: β=-0.54, p=3.3×10-7).
Biomarkers associated with disease severity, as defined by mechanical ventilator use, are illustrated in Figure 1B and compared to the disease-associated markers identified in Figure 1A. Overlapping markers, including EZR, nicotinamide adenine dinucleotide kinase, and KRT19, may be useful biomarkers for diagnosing infection and monitoring disease severity. IL-6, which was only slightly elevated among cases (β=0.60; p=0.02), was significantly correlated with severity. However, the majority of proteins associated with severity were different from those associated with disease development. Measures such as DDAH1 and NPM1 were also associated with severity among COVID-19-negative controls, indicating a lack of specificity for certain proteins.
Baseline levels of DCN, S100P, and the cardiac biomarker IL1RL1/ST2 were suggestively associated with maximum-observed severity (i.e., death or requiring ventilation) within the 28 days (p=1.2×10-6, 1.8×10-4, 1.3×10-12, respectively), even after correcting for baseline severity (p<5×10-5). Plasma levels of S100P were lower among cases compared to controls (β=-0.48; p=0.0004), but levels of DCN and IL1RL1 were not affected at baseline. However, IL1RL1 was associated with baseline severity (β=0.83; p=1.2×10-8). Chemokine CXCL10 was also correlated with baseline severity and prognosis (β=0.63; p=0.0003; β=0.89; p=0.0005).
As most cases were >50 years old, many had pre-existing conditions such as diabetes and hypertension (Table 1). Patients with COVID-19 were more likely to be obese but had unexpectedly lower rates of lung disease and diabetes, although this may be due to selection bias among controls. Association between baseline protein levels and pre-existing conditions among cases was examined in Figure 1C. Leptin, a metabolism-regulating hormone primarily secreted by adipose tissue,34 was the primary protein associated with obesity but was only slightly increased among cases (β=0.681; p=0.0007). Cardiac biomarkers B-type natriuretic peptide (BNP) and its N-terminal pro-hormone (NT-proBNP) were negatively associated with obesity but positively associated with pre-existing heart disease. Both were under-expressed in COVID-19 at baseline (β<-1.19, p<2×10-7) but increased significantly during follow-up (β>1.1; p<3×10-5). BMI significantly modified baseline association for both cardiac biomarkers (normal: β>-0.71, p>0.20; obese: β<-1.82, p<2×10-8). Fibroblast growth factor 19 was elevated in patients with hypertension and possibly those with pre-existing kidney disease (unadjusted: β=0.73, p=2×10-5; adjusted: β=0.77, p=0.08).
To determine if specific biological processes overlap between COVID-19 disease and related comorbidities, modules of intercorrelated proteins were identified and used to cross-examine associations between disease characteristics, pre-existing conditions, and relevant biomarkers established in previous studies. As illustrated in Figure 2, several identified modules correspond to over- (green) and under- (turquoise/black) expressed proteins among patients with COVID-19. As previously illustrated, these tend to differ from proteins associated with disease progression (red/turquoise) and severity (yellow).
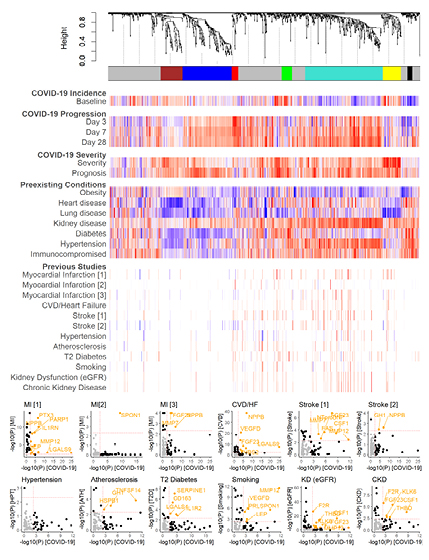
Figure 2: Comparison of protein modules associated with COVID-19 and disease-related pre-existing conditions and complications.
Dendrogram and coloured bars illustrate intercorrelated protein modules among patients who tested positive for COVID-19, determined by weighted correlation network analysis. Colours indicate a normalised score (-1: blue; +1: red) of the effect estimate divided by standard error, with colour and intensity corresponding to the direction and strength of the association, respectively. Scores were normalised within each study by the 95th percentile of the absolute scores to allow inter-study comparability and minimise outlier effects. Studies are referenced in the methods by order.
CKD: chronic kidney disease; CVD: cardiovascular disease; eGFR: estimated glomerular filtration rate; HF: heart failure; HPT: hypertension; KD: kidney dysfunction; MI: myocardial infarction; log10: logarithm with base 10; T2: Type 2.
There was a significant overlap in markers associated with pre-existing kidney disease and hypertension (turquoise), which were responsive to COVID-19 infection and increased with disease progression. Many have been previously established as markers of reduced kidney function, based on estimated glomerular filtration rate, and increased risk of developing chronic kidney disease.
BNP and NT-proBNP (left-end turquoise) were associated with myocardial infarction, heart failure, and ischaemic stroke. Its moderately correlated protein matrix metallopeptidase 12 (MMP12) was similarly elevated in both myocardial infarction and stroke. MMP12 was also higher among smokers, being associated with inflammation in emphysema; therefore, it may be a shared pro-inflammatory mediator between COVID-19 and severity-related comorbidities. The protein module associated with disease severity (yellow) overlapped with biomarkerAs for increased myocardial infarction risk such as AGER, CTSL, PARP1, and SOD2. Many severity-related measures were also associated with pre-existing lung disease, although in the opposite direction. Proteins of the last module (black), which include ITGA11, continued to decrease throughout the 28-day observation period. Considering its moderate overlap with obesity and diabetes, the module may be related to long-term metabolic dysfunctions.
DISCUSSION
This study provides a broad overview of the systemic effects of COVID-19 measured through blood, a natural sink for multiple organ systems and an easily accessible medium for clinical investigation. Findings indicate a significant disruption in the circulating proteome of infected patients, impacting multiple biological processes relating to pulmonary inflammation along with cardiac injury and renal dysfunction. These findings support the observation of multi-organ complications reported in previous clinical studies.4,7,35
As expected, many pro–inflammatory cytokines were elevated during the early stages of the disease and may influence overall severity, as shown in the previous studies.3,4 The hyperactive immune response against SARS-CoV-2 infection, often referred to as a ‘cytokine storm’, has been hypothesised to be a cause of disease mortality.36,37 Interferon-γ-induced chemoattractant CXCL10 was one of the primary cytokines elevated in cases and was a suggestive marker for disease severity. Previous studies have also shown increased levels of CXCL10 along with CCL7 (MCP-3) in COVID-19, and CXCL10 was a suggested biomarker for ARDS with protein DDX58.38,39 As IL-6 blockade has been effective for managing cytokine release syndrome, IL-6 has also been investigated as a therapeutic target for COVID-19.36 Although not notably elevated among cases in this study, higher levels of IL-6 were associated with increased severity. Findings further support the potential benefits of such treatment. As IL-6 is also a marker with myocardial infarction and smoking, treatment efficacy may be modulated by active cigarette smoking or pre-existing cardiovascular disease targeting related disease complications.25,27
Several cardiac biomarkers were influenced by COVID-19, including the hormone BNP and its inactivated-form, NT-proBNP. Both are known predictors of acute cardiac injury and heart failure27,40 and have been proposed as prognostic measures for increased mortality among patients with COVID-19.41 A retrospective examination of deceased patients with COVID-19 has also shown elevated levels of circulating NT-proBNP during hospitalisation.4 Therefore, BNP-related measures may be useful for monitoring cardiac stress and the risk of thromboembolic complications, particularly among those who are obese or with pre-existing heart conditions. Another biomarker, IL1RL1, also known as ST2, is associated with cardiac remodelling, and soluble ST2 has been a marker of acute myocardial infarction.42-44 Elevated levels of ST2 were associated with increased mortality rate and may be an additional complementing marker of cardiac complications for COVID-19.45
Other proteins may also indicate cardiac-related injury, including CDON, which, along with its co-expressed partner BOC, were lower among cases. CDON deficiency in mice has been associated with cardiac remodelling and fibrosis through hyperactivation of the Wnt/β-catenin pathway and may indicate an increased risk of cardiac injury and heart failure in patients.46 CDON levels were also negatively correlated with severity and, to a lesser extent, disease prognosis. On the other hand, MMP12, an increased measure associated with cigarette smoking, may be a mediator of pulmonary inflammation32,47 in COVID-19 and pre-existing lung conditions and cardiovascular-related comorbidities.
As the primary entry receptor for SARS-CoV-2, ACE2 is an essential moderator of blood pressure and has often been examined in the relationship between COVID-19 and cardiovascular complications. Studies have suggested that SARS-CoV-2 infection can affect the expression of ACE2 pathways in the heart and increase cardiac complication risk associated with localised inflammation.48 ACE inhibitors frequently used to manage hypertension have been associated with increased risk of kidney injury among patients with COVID-19.49 Furthermore, increased levels of soluble ACE2, as seen among cases in this study, may offer some protection against SARS-CoV-2 infection by inhibiting receptor-binding activity, although this requires further clinical investigation.50
Studies have also shown ACE2 expression in kidneys, which may indicate a direct relationship between SARS-CoV-2 infection and renal complications.51 Systematic release of pro-inflammatory cytokines in ARDS may increase the risk of acute kidney injury, while its resulting accumulation from reduced renal function may, in turn, exacerbate ARDS.52 In this study, several proteins were associated with both COVID-19 and kidney dysfunction, and may be possible biomarkers for monitoring acute injury, particularly among those with hypertension.
The long-term complications of COVID-19 could not be directly assessed in this study due to the limited follow-up time. However, previous studies have reported lasting changes in metabolic and sensory functions, particularly among severe cases.17,53 Although levels of proteins like ILTGA11 seemed to show long-term disruptions from disease, proper longitudinal studies will be required to investigate its trajectory. Although this study focused on circulating proteins, other media such as sputum may provide more localised measures for COVID-19.47 Similarly, urine and cerebrospinal fluid may be better for assessing renal and neurological complications. However, blood remains the single most comprehensive source for assessing systemic effects, and the sensitivity of proximity extension technology allows the detection of trace proteins from multiple organ systems. Unfortunately, previous studies have been limited to targeting specific aspects of the blood proteome, which significantly limits the resolution for comparing overlapping biological processes. However, the recent incorporation of NGS readout in a proximity extension assay will likely provide a more comprehensive disease proteomic profile in future studies.
In summary, this study provides an initial assessment of the overlapping biological processes associated with COVID-19 and related comorbidities. Cardiac biomarkers NT-proBNP, BNP, and ST2 may be useful for monitoring and assessing the risk of cardiac and cerebrovascular complications, while specific measures of inflammation such as CXCL10, IL-6, and MMP12 may be useful for identifying patient groups responsive to immunosuppressive treatments. However, further investigations are required to validate the efficacy of these potential biomarkers in clinical settings.