Abstract
One of the components of a health system as defined by the World Health Organization (WHO) is data that the health system collects, generates, and uses to improve the health of its population. The aim of this paper is to describe key lessons from Denmark in planning, implementing, and using health system-generated data, using Type 1 diabetes as a tracer. A broad literature search was complemented with a review of grey literature, two series of interviews with Danish experts, and feedback from a presentation of these findings to two expert meetings. Denmark, through its unique identifier and data environment, enables the health system to collect a variety of data on Type 1 diabetes. Facilitators and challenges exist for data collection, data aggregation, use of data, communication of data and results, and intangible factors. For each of these, the environment, infrastructure, health system, and Danish society act as facilitators. Barriers relate to data being collected primarily for clinical and administrative purposes, and not necessarily for research, planning, policymaking, or advocacy. Fora are lacking to facilitate the communication and presentation of these results. An intangible element is the trust Danes have in their system, which is hard to replicate. As shown in the Danish setting, contextual factors cannot be negated in developing and implementing data-related solutions at a population level. The lessons from Denmark show that there is the need to conceive and act on all aspects of the data from its collection, aggregation, use, and communication. The last step of interaction between science and policy and practice requires a range of factors, including networks and knowledge brokers.
Key Points
1. Data is an integral part of the health system. For diabetes, this data can be used for a variety of elements, from describing epidemiology, to ensuring appropriate follow-up of an individual. Denmark provides an example of an environment that collects and uses data for a variety of purposes.
2. This paper describes the Danish Type 1 diabetes environment, as well as the facilitators and barriers that exist. Facilitators relate to the environment, infrastructure, health system, and society. Barriers are that the data is primarily collected for clinical and administrative purposes, not for research, planning, policymaking, or advocacy. Another barrier is the means to communicate such results.
3. The lessons from Denmark show that there is the need to conceive and act on all aspects of the data, from its collection, aggregation, use, and communication. The last step of interaction between science and policy and practice requires a range of factors, including networks and knowledge brokers.
INTRODUCTION
The World Health Organization (WHO) defines six key components of a health system.1 This includes data that the health system collects, generates, and uses to improve its management and care of the population. Data in the context of health can include patient records, disease-based or facility-based registers, sub-national and national registers and clinical databases. The aggregation of this data can help the health system in monitoring population health (incidence, prevalence, morbidity, and mortality), planning (medicines, supplies, human resources, etc.), as well as for quality and monitoring and evaluation purposes.2,3 Policies also require data in their formulation, implementation, and monitoring, and, therefore, the health system also needs to generate information that can be used by policymakers.4
In 1989, a meeting of diabetes associations, the WHO, and representatives of Ministries of Health in Europe developed the St. Vincent Declaration on diabetes care and research in Europe.5 Included in this declaration was the need to collect data to ensure improvements in the management of diabetes and the prevention of complications. More recently in Europe, in 2022, the European Union (EU) Parliament adopted a resolution on diabetes which states: “The Commission should follow through with the Healthier Together – EU Non-Communicable Disease Initiative, inter alia by collaborating with Member States in developing and implementing common, standardised criteria, and methods for data collection on diabetes, and in collecting, registering, monitoring, and managing comprehensive epidemiological data on diabetes, as well as economic data on the cost of diabetes prevention and management in the EU, including patient preferences and patient-generated data.”6 Similar messages on the need to strengthen health information systems for diabetes have also been affirmed by the World Health Assembly (WHA) in 2021, with a resolution entitled ‘Reducing the burden of noncommunicable diseases through strengthening prevention and control of diabetes’.7
Bak et al.8 state that diabetes registers allow for a better understanding of diagnosis, complications, and management. However, their impact policy is less clear. Khunti et al.9 propose recommendations for the development of Type 2 diabetes registries in Europe. They highlight the need for buy-in from multiple levels of the health system, including policymakers and frontline health professionals who are responsible for entering the data. Denmark can serve as a model for other countries in its experience of using registers and the collection of diabetes-related information.10 Using Type 1 diabetes as a tracer,11 the aim of this paper is to describe some key lessons from Denmark for other settings in planning, implementing, and using health system-generated data for Type 1 diabetes.
METHODS
Context
Denmark has a population of 5.8 million (2020), with a life expectancy for females of 83.6 years, and 79.5 years for males (2020)12 and a gross domestic product per capita of 60,909 USD (at current conversion rate in 2020.)13 Healthcare is provided for free, with over 10% of gross domestic product being spent on health.14
Approach
The focus of this paper is to document the data environment for Type 1 diabetes in Denmark. This was done by a broad literature search on PubMed using the following search terms: “Denmark,” “diabetes,” “Type 1 diabetes,” “registers,” and “epidemiology.” The search was carried out from January 2011–August 2021, and then updated until March 2022. Reports and publications from the Ministry of Health, Regional Authorities, Danish Diabetes Association (Diabetesforeningen), WHO, and other international organisations were also included as part of this literature review. Bibliographies of articles and documents were used to identify further resources.
This review was complemented by two series of interviews with Danish experts. One set of interviews was with key opinion leaders in the field of diabetes to learn more about clinical practice, the use of data, and gain further insight into the literature from a practical angle. The other was carried out with a purposive sample of experts playing different roles within the Danish Health System, to learn about how data is used to inform policy and practice. These interviews were carried out using a discussion guide that used ‘Grand Tour’ questions15 to gain a ‘verbal tour’ of different areas of the collection, use, and dissemination of data in Denmark. The interviews also used prompts to delve into certain areas mentioned by the experts. Interviews were recorded, anonymised, and analysed thematically.
The final component was to present the results of this work at two meetings held in November 2022. One meeting included Danish diabetes experts, and the other a panel of international diabetes experts. Feedback was provided on the initial results of the lessons learnt from Denmark to further refine these and develop the material presented in this paper.
RESULTS
The Danish Data Environment
The Danish Civil Registration System provides each person residing in Denmark with a unique 10-digit identifier.16 All registers in Denmark use this unique identifier allowing for linkages to be made between different data sources for administrative and research purposes. The Danish Health Data Authority (DHDA) has the responsibility for managing the Danish health registers, and the use of this data for research is regulated by law.17 Data is available to authorised users through a centrally protected environment.18 Individual data can be accessed by researchers, but these need to be published in an aggregate way to ensure that individuals cannot be identified.18
Within the health data environment, there is the Danish National Patient Register, which includes individual data on diagnosis, treatments, etc., for inpatient, outpatient, and emergency visits.19 Reporting to this register is mandated by law, and each outpatient visit or hospital discharge needs to be documented. In Denmark, other registers are also present. The National Health Service Register20 includes information from primary healthcare, and is used for the payment of services. For individual prescriptions, the Danish National Prescription Register21 collects this information. Reporting of all deaths, including information on causes of death, is part of the Danish Register of Causes of Death.
The Danish Diabetes Data Environment
In 1996, the Danish Childhood Diabetes Registry was established. This registry was set up to collect data from paediatric facilities caring for children aged 0–15 with Type 1 diabetes,22 for both epidemiological and monitoring purposes.23 This register collects laboratory, clinical, treatment, and demographics for each individual. The National Diabetes Register, established in 2006 by the Danish National Board of Health (now DHDA), was created as a tool for “monitoring the clinical course in patients with diabetes.”24 This ceased to operate in 2012,25 and was replaced by the Register of Selected Chronic Disease and Severe Mental Disorders (RUKS).26 Specific diabetes research databases have also been established using data from the central Danish health registers mentioned above.27 In addition, a Nationwide Clinical Quality Database for diabetes combines information from different sources, to assess quality for a series of core indicators defined by the health system.28
Lessons from Denmark
In Denmark, there are different facilitators and barriers which are present at national, health system, and population level. These exist for data collection, aggregation, use, and communication. In addition, intangible factors are present.
Data collection
Experts describe a variety of factors that facilitate data collection at a national level. This includes the tradition of the data environment in Denmark, governance of the health system, digital infrastructure present, and a high level of digitalisation in Danish society. Data collection is part and parcel of the daily activity of the health system, with this data being used for improving care and the system. Interviewees also expressed that people entering the data felt a sense of responsibility for doing this. From the population’s perspective, experts stated that there was underlying trust in the government, and that the data would be used properly.
The only barrier mentioned at the level of the government was regarding the General Data and Protection Regulation of the EU, that Denmark, as a member of the EU, had to follow. Barriers at health system and research levels were related to three elements. Firstly, that the data did not currently capture all aspects of laboratory tests, and did not include patient-centred and reported outcomes. Another factor was that the onus of data collection was on clinicians. Finally, the data can have many uses for clinical, administrative, and research purposes, but limitations exist for each of these.
Data aggregation
Data aggregation is a key function of the Danish Diabetes Data environment that allows for individual patient data to be combined at clinic, facility, regional, and national levels. Being able to aggregate this data at these levels allows for comparisons, quality assessments, development of national reports, and research to be carried out. A key component that allows for data aggregation is the standardisation of data collection. The unique identifier was also a tool to aggregate data across different data sources.
The challenges related to data aggregation is that, as with data collection, the primary reason these data are collected is not for research purposes. Therefore, there is the need for validation of the clinical data, and if the codes used are relevant for research purposes. Interviewees also relate that capturing complexity is hard with the existing data. The challenge is that if researchers are interested in looking at multiple factors, e.g., pump use and hypoglycaemia, or mental health and Type 1 diabetes, the aggregation of the different data points can be difficult. Experts also highlighted two other challenges. The first challenge is that the use of the register data requires both clinical and epidemiological knowledge to contextualise and navigate the data. The other is that with the increase of user generated data in Type 1 diabetes with continuous glucose monitors, there is no way to integrate this in the existing data capture.
Use of data
Facilitating the use of diabetes-related data in Denmark is also the unique diabetes environment. This includes academia and the different Steno Diabetes Centers established in each region of the country. These centres of excellence facilitate the interaction between researchers and clinicians for the data to be used in different ways.
The challenges reported for the use of diabetes data was that the data is complex to use, and it requires significant investment to present the data in formats that are relevant for different stakeholders. Key informants described how policy and decision-makers could access data generated by the health system, directly from the system itself, researchers, or advocates, but that often knowledge brokers played a key role as intermediaries in this process. The Danish Diabetes Association was one example of a knowledge broker that was mentioned, in that it had the capacity to use existing data from the system, as well as collecting its own information and use these for advocacy purposes. Other examples were different key opinion leaders who were either clinicians or researchers, who also had administrative roles within the health system, and used their knowledge of the diabetes data in non-scientific settings. Interviewees also state that just because the data is collected and aggregated, this does not mean it will be used, and this can depend on interest of individuals to carry out research in a specific area.
Communication of data and results
Interviewees highlight a variety of factors that facilitate the communication of data and results in Denmark. This includes that Denmark is a small country, the existence of diabetes-related networks, and an active interest in Type 1 diabetes. Beyond this, many experts state that beyond the communication of the results, there is a need to “translate” the data and findings so that these can be useful, and integrated into policy and practice by different stakeholders. In this process, knowledge brokers were mentioned as a key component. Those interviewed highlighted the need for a two-stage process in generating “useable” information, and then being able to provide this in a format that was adequate for the given audience, be it at clinic, facility, regional, or national levels, or for non-expert audiences. Different formats are needed, as well as platforms for communicating these results.
Intangible factors
The interviews also highlighted the importance of intangible factors present in Denmark that facilitated the unique data environment. A recurring theme was the element of trust that Danes have in their government and the health system. The other point was that people were glad to give their data in exchange for the variety of health and social benefits they receive for free. Transparency was another intangible factor, in that the system allows individuals to access their own data. Two elements that could possibly be challenges to the intangible factors in the future were mentioned. These were the fear of health data being shared in a post-COVID-19 society, as well as the increase in ‘big data’ from Google (Mountain View, California, USA), Apple (Cupertino, California, USA), Facebook (Meta, Menlo Park, California, USA), and Amazon (Seattle, Washington, USA).
The barriers and facilitators for each of these components is presented in Table 1.
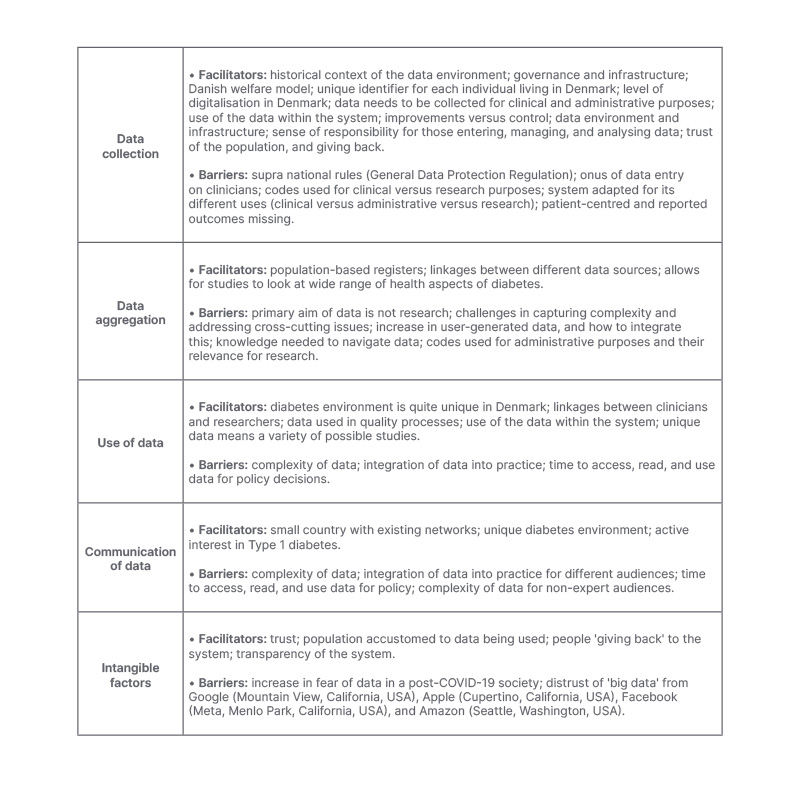
Table 1: Facilitators and barriers for different elements of the Danish Diabetes Data environment.
DISCUSSION
For data collection, aggregation, use, and communication, certain lessons can be learnt from Denmark that might be of use for other contexts, and enable the operationalisation of some of the statements and recommendations being made to improve data on diabetes in Europe and beyond. For data collection, the lesson is that although the data collected allows for a variety of information to be generated, fundamentally the data is collected for clinical and administrative purposes. Therefore, the data system needs to focus on the clinical interaction, and be integrated into clinical practice. The system needs to start simply, and collect data that is useable for the clinician, health system, and decision-maker, in that order of priority. The cost of setting up the system should not be minimised, and, therefore, there is the need to focus on what is essential in addition to having a system that can evolve, as retrofitting is complex and costly. Data aggregation needs to start, with the data being used to improve clinical and administrative outcomes and processes. This focus impacts the use of this data for research purposes, as researchers are then reliant on what and how data has been collected within the registry’s infrastructure. A balance needs to be found between clinical and research results, requiring partnerships to be established between clinicians, administrators, and researchers, to make the most out of aggregating existing data. For the use of the data, those using the data need to be aware of their responsibility in its proper use, which needs to align with Danes willing to share their data as part of their social contribution to maintain their trust. Leadership is needed from the system and user boards, control mechanisms, and who and how research projects are designed should be included as procedures to be put in place. Finally, lessons on the communication of data and results show that there is the need to understand the results that are produced by the data, and then be able to present this in a clear and comprehensive way to different audiences using knowledge brokers. Overall lessons are summarised in Table 2. Beyond these lessons, which can be taken and applied to other settings, the intangible factors present in Denmark cannot be ignored, as the unique historical, political, and social context cannot necessarily be replicated, but must be considered.
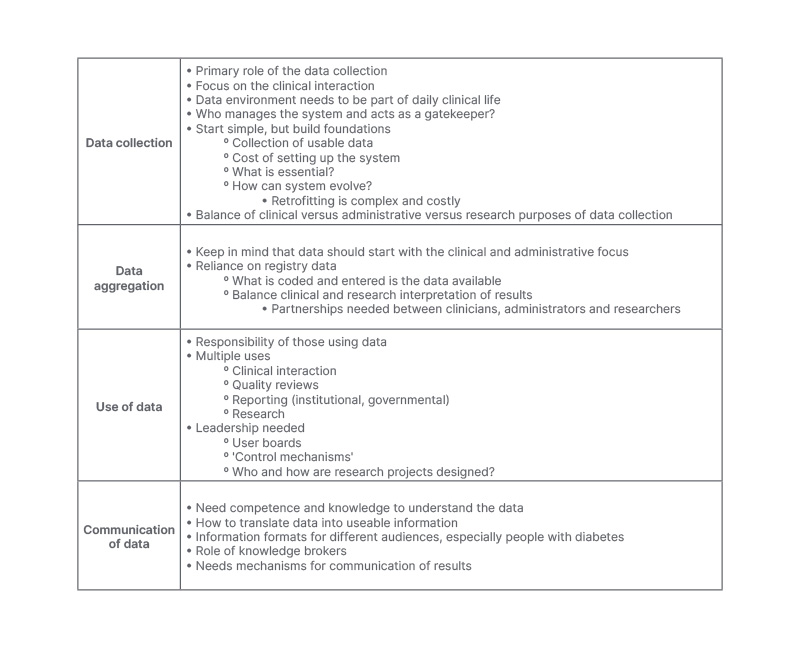
Table 2: Lessons learnt on data collection, data aggregation, data use, and communication of data and results.
Another key element from Denmark is that Danish data informs Danish practice. In many other contexts, countries are reliant on global estimates or modelling. Different estimates exist for Type 1 diabetes,29-33 but these show significant variation in the numbers they present.34 Critiques have been raised about these approaches to defining the burden of disease where there are ethical issues in using such estimates, issues of transparency in how these estimates are developed, their trustworthiness, and who owns them.35 In order to improve the data for diabetes globally, a proposed roadmap might include global, national, and facility-based investments.34 Previously, global and European-based registry studies have existed, namely the WHO Diabetes Mondiale (DIAMOND)36 study, and the EURODIAB register.37 These studies would need to expand, as previously they only collected childhood data, and estimates now show that in many contexts, the largest number of prevalent cases is in the adult population.29 Globally, guidance and protocols, such as those developed by the International Diabetes Federation (IDF),38 could be used to develop tools and targeted studies to fill existing gaps in knowledge. At a national level, clearly the investments and infrastructure needed to develop a system like Denmark’s will be challenging, but new technologies might be an opportunity to build less onerous data collection environments. Beyond ongoing data collection and the establishment of registries, other ways of documenting diabetes could be employed. This could be in the form of a formal research study, or the use of existing data from published and unpublished data, presented as report cards, dashboards, or White Books. Ultimately, diabetes registers, or comprehensive data collection, will be needed to both ensure improved clinical outcomes and overall disease burden, as well as measurement of the attainment of the WHO’s first global coverage targets for diabetes.39 These targets include the aim of “100% of people with Type 1 diabetes having access to affordable insulin and blood glucose self-monitoring.” To achieve these goals, knowledge of Type 1 diabetes needs to be improved.
There are some limitations to this work. Firstly, only PubMed was used in the literature review, and any limitations in the search strategy may have had an impact. This limitation also applies to non-peer reviewed material. As many documents were in Danish, online translation tools were used, resulting in possible misinterpretations.These limitations were addressed by working closely with Danish experts, as well as having all the material reviewed during two expert meetings. The choice and participants at this meeting may have also impacted their feedback, and thus the overall work. Limitations are also present with the interviews of key opinion leaders, as their selection was done purposively. Interviewer and interviewee bias, as well as the analysis being done by only one person, need to be considered.
CONCLUSION
Even in well-resourced health systems, to create a positive data environment for diabetes as it exists in Denmark will require substantial investments. As shown in the Danish setting, contextual factors cannot be negated in developing and implementing data-related solutions at a population level. The lessons from Denmark show that there is the need to conceive and act on all aspects of the data, from its collection, aggregation, use, and communication. The last step of interaction between science and policy and practice requires a range of factors, including networks and knowledge brokers. Margaret Chan, former Director-General of the WHO, is often quoted as having said during her tenure that: “What gets measured, gets done.” With the importance of diabetes being highlighted at national, continental, and global levels, approaches need to be developed to measure different elements of diabetes, to ensure improved health systems and policy responses for the benefit of people with diabetes.