Abstract
Biological disease-modifying anti-arthritis drugs (bDMARD) have transformed rheumatoid arthritis (RA) treatment and allowed many patients to reach clinical remission. With the huge growth in the development of different bDMARDs, there is now a need to decide on which treatment should be prescribed to achieve optimal patient outcomes. Decisions are made by weighing up the comparative efficacy of each agent against risks, namely the risk of bacterial infections. The most powerful tools for investigating the comparative efficacy of bDMARDs are head-to-head trials that directly compare one therapy to another; however, very few trials of this type exist. Furthermore, the heterogeneity of RA calls for consideration of the comparative efficacy of therapies on an individual basis. Many studies have found associations between specific biomarkers and response to different bDMARDs to enable stratification of patient groups, although many results have not been reproducible in different cohorts. Combining predictors to create models of treatment response may be the ultimate key to finding reliable biomarkers with enough predictive power to enable a personalised medicine approach to treating RA in the clinic.
INTRODUCTION
The arrival of biologic disease-modifying anti-arthritis drugs (bDMARD) has vastly improved the outcomes of patients with rheumatoid arthritis (RA) by suppressing inflammation. Conventional synthetic biologic disease-modifying anti-arthritis drugs such as methotrexate (MTX), hydroxychloroquine, and sulfasalazine, which inhibit non-specific inflammatory pathways, are considered for first-line treatment for RA. Following an inadequate response to these agents, bDMARDs that target specific parts of the immune system may be prescribed. These act through various mechanisms such as inhibition of TNFα (adalimumab, etanercept, and infliximab), IL-6 receptor blockage (tocilizumab and sarilumab), T cell inhibition (abatacept), and B cell depletion (rituximab). More recently, the development of JAK inhibitors has marked the first targeted synthetic disease-modifying anti-arthritis drugs to enter the clinic that may be considered alongside bDMARDs.
As per the National Institute for Health and Care Excellence (NICE) guidelines, a treat-to-target strategy is employed for treating active RA to achieve remission or, if that is not possible, low disease activity. Prescribing bDMARDs in combination with MTX is common practice as this has been shown to be more generally effective than monotherapy, likely due to the effect of MTX on autoantibody production. Although bDMARDs have revolutionised RA therapy, this is not the case for all patients, with approximately one-third of patients not responding to bDMARDs.1 However, this comes as no surprise when the vast heterogeneity of RA pathotypes is taken into consideration. When faced with this inconsistent response and the choice between many therapies with diverse mechanisms of action, clinicians can face challenging decisions about which agent to prescribe and when.
This article will outline the current literature to compare different bDMARDs in terms of benefits and risks, and discuss how it may be possible to stratify patients to move towards a personalised medicine approach.
METHODS
The authors conducted database searches for screening studies for inclusion in the review from their inception to 1st January 2021. Data were retrieved from databases including PubMed, Ovid Medline, and Embase. Keywords for searches included: “rheumatoid arthritis”, “clinical trial”, “biological therapy”, “comparison”, “efficacy”, “infection risk”, “treatment”, “response”, “predicts”, “biomarker”, and “personalised medicine.” A total of 11,616 papers were retrieved including duplicates. After review by the authors, articles were excluded if they were out of the remit of the review; e.g., including other agents or diseases, were not published in English, or included animal studies only. A total of 144 abstracts were screened and 70 papers were included in this article. This is not a systematic review or meta-analysis. Where possible, clinical trial data and biomarker results were organised in tabulated form ( Tables 1 and 2 ).
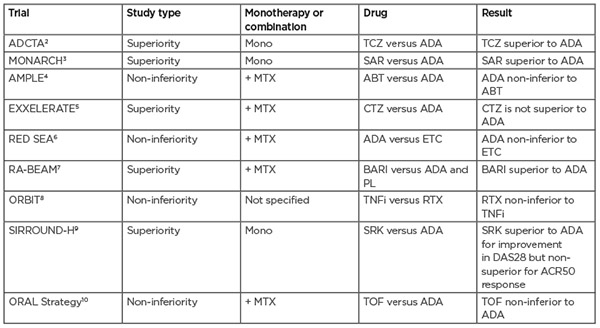
Table 1: Head-to-head trials of biologic agents for the treatment of rheumatoid arthritis. Trials that did not directly compare biological agents or did not measure treatment response were excluded. ABT: abatacept; ACR50: American College of Rheumatology 50; ADA: adalimumab; BARI: baricitinib; CTZ: certolizumab pegol; DAS28: Disease Activity Score 28; ETC: etanercept; MTX: methotrexate; PL: placebo; RTX: rituximab; SAR: sarilumab; SRK: sirukumab; TCZ: tocilizumab; TNFi: TNF inhibitor; TOF: tofacitinib.
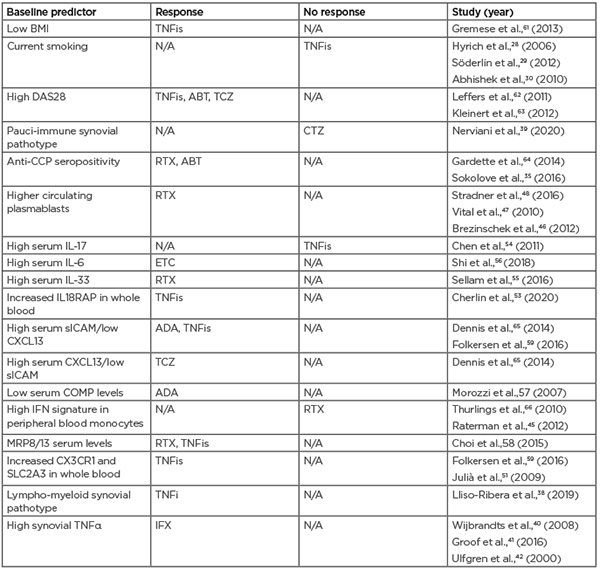
Table 2: Predictors of drug response for biologic agents used to treat rheumatoid arthritis. ABT: abatacept; ADA: adalimumab; CCP: cyclic citrullinated peptide; COMP: cartilage oligomeric matrix protein; CXCL13: chemokine ligand 13; DAS28: Disease Activity Score 28; CTZ: certolizumab pegol; ETC: etanercept; IFX: infliximab; N/A: not applicable; RTX: rituximab; sICAM: soluble intercellular adhesion molecule; TCZ: tocilizumab; TNFis: tumour necrosis factor inhibitors.
COMPARING BIOLOGICAL THERAPIES IN TERMS OF EFFICACY
Being the oldest class of bDMARDs, TNF inhibitors (TNFis) are well-studied, which, along with their low cost, makes them a popular choice for clinicians. Therefore, the contrasting lack of data for newer forms of therapy can pose difficulties for making reliable comparisons. The most useful conclusions can be drawn from head-to-head trials that directly compare one agent to another within the same patient cohort as opposed to using data from placebo-controlled trials or making comparisons between trials on different patient cohorts.
To date, there have only been a handful of head-to-head trials directly comparing different biological therapies. These can either be in the form of superiority trials, which are powered to determine if one therapy is better than the other, and non-inferiority trials, which investigate whether one therapy is at least as good as the other. In two superiority trials, the IL-6 inhibitors tocilizumab and sarilumab were found to be more effective than adalimumab when used as a monotherapy; additionally, in the RA-BEAM trial, baricitinib was found to have significantly greater clinical improvements than adalimumab.2,3 Table 1 summarises findings from hitherto head-to-head trials.
There have been efforts to compare the efficacy of bDMARDs using data from various clinical trials via meta-analysis; however, many of these have not been in agreement or have been criticised in terms of methodology (e.g., combining DMARD-naïve and DMARD-inadequate responder patients or combining monotherapy and combination therapy in one analysis).11 A recent systematic meta-analysis compared eight bDMARDs used as combined therapy with MTX.12 One major strength of this analysis is the inclusion of similar patient populations by using aggregate results from systematic re-analyses of individual patient data performed by the study sponsor. Overall, only a few statistically significant differences were found between the bDMARDs analysed, with anakinra showing the least benefit. This is consistent with two previous meta-analyses and reflected in the NICE guidelines, which do not recommend this therapy on the balance of cost-effectiveness and clinical benefits.13,14 In contrast, a multiple treatment comparison regression modelling approach found, when given as combination therapies, the therapies ranked as follows: certolizumab as the most effective followed by tocilizumab, anakinra, rituximab, golimumab/infliximab/abatacept, and adalimumab/etanercept as the least effective. It is interesting to note that they found a higher dose level had a significant effect on treatment and no effect was found for disease duration.15 In summary, with only a small number of studies looking at direct comparisons in the same cohort of patients, it can be difficult to draw conclusions on the comparative efficacy of biological therapies, therefore more data from head-to-head trials are needed to help to unveil these trends.
QUALITY OF LIFE MEASURES
The most commonly used outcome measures for assessing RA disease activity are the American College of Rheumatology (ACR) and European League Against Rheumatism (EULAR) criteria for remission and low disease activity used by clinicians; ACR20 is commonly used in clinical trials, which is a composite measure involving a set of five criteria. These tend to have a large contribution from measures looking at the reduction in inflammation. However, a common problem seen among patients is the persistence of fatigue and pain, even if inflammation is well controlled. This highlights the importance of considering an alternative perspective: how bDMARDs compare to each other for improving specific quality of life measures. The authors have not been able to identify any published research addressing this question, though there is some suggestion that different bDMARDs may contrast in their ability to treat certain aspects of RA. For instance, in the AMPLE trial adalimumab had a greater reduction in pain assessment score than abatacept.4 A head-to-head trial is currently underway to investigate this finding further (BIORA-PAIN).16
RISK OF INFECTION
In addition to the comparative benefits of biological treatments, another aspect to consider is the comparative risk of infection between therapies. When making judgements about the safety of therapies, it is also important to note that people with RA have a risk of infection that is higher than the general population.17 However, the added risk of infection following administration of biological agents is widely recognised, with many studies reporting this to be higher than conventional synthetic biologic disease-modifying anti-arthritis drugs. This risk has been shown to increase with dosage and is highest within the first 6 months of the first course of TNFi therapy.18 The most common infection caused by biological therapies is pneumonia, primarily caused by the reactivation of a latent Mycobacterium tuberculosis infection. In the case of TNFis, it could be postulated that TNF’s role in stimulating the phagocytosis of M. tuberculosis may be driving this response.
Many studies have suggested a lower risk of infection for abatacept. The ATTEST trial looked at infliximab versus abatacept with MTX and found substantially lower rates of serious infections with abatacept (1.9% versus 8.5%),19 and a meta-analysis by Salliot et al.20 found abatacept did not significantly increase the risk of serious infection in patients with RA. More recent studies have supported these data. A multi-database cohort study found abatacept had a lower composite risk of serious infections when compared to tocilizumab.21 Additionally, a propensity score-matched cohort study with 11,248 patients on abatacept or a TNFi found a lower risk of hospitalised respiratory infection for abatacept versus TNFis (particularly infliximab), and a large retrospective cohort study of 31,801 patients found that exposure to etanercept, infliximab, or rituximab was associated with a greater 1-year risk of hospitalised infection compared with the risk associated with exposure to abatacept.22 This is in line with the AMPLE head-to-head study comparing adalimumab and abatacept, where adalimumab patients experienced more serious infections (3.8% versus 5.8%) and more discontinuations as a result.4 How the risk of infection varies between other biological agents is not yet clear. Some data alludes to a lower risk for etanercept versus adalimumab,14,23 and one head-to-head study found no differences in safety between sarilumab and tocilizumab.24
BIOLOGICAL SWITCHING
After at least 3 months of non-response to a bDMARD, patients are prescribed a different bDMARD; this is known as biological cycling or switching. This particular timeframe is used, as no response after 3 months is a predictor of a lack of responsiveness after 1 year of therapy on the same biological agent.25 Patients who do not respond to therapy fall into two categories: a response that falls over time, and no initial response. Patients in the former group may have developed anti-drug antibodies, in which case cycling to another TNFi inhibitor may still show benefit; however, these antibodies may have cross-reactivity with a biosimilar.26 Patients in the latter group may benefit from switching to a biological therapy with an alternative mechanism of action. This approach has been demonstrated to be beneficial over TNFi cycling for the majority of patients in multiple studies.27 However, this trial-and-error method of choosing therapies is far from ideal and can lead to continued disease progression and low quality of life, while risking exposure to side effects and wasting costly therapeutics. This calls for an urgent need to uncover predictors of patient response to improve patient outcomes.
PREDICTORS OF RESPONSE
Generic Predictors of Response
Patient characteristics and clinical data have been able to draw out overall trends in treatment response. Current smoking has been associated with a lack of response to TNFis in several studies,28-30 but this trend was not seen with a study on tocilizumab.31 Additionally, younger age, male sex, lower BMI, shorter disease duration, no-comorbidities, low Health Assessment Questionnaire (HAQ) score, and high Disease Activity Score 28 (DAS28) predict a better response to bDMARDs.32,33
Current Tools
The only predictive tools currently in clinical use are testing for rheumatoid factor and anti-citrullinated protein antibody positivity. These are shown to correlate with disease severity and are associated with response to rituximab and abatacept; however, this trend is not seen with TNFis, with conflicting reports or no association found.34,35 Blood erythrocyte sedimentation rate and C-reactive protein (CRP) levels are commonly used markers that can indicate inflammation and the requirement of more aggressive therapy, but cannot determine which bDMARD to use. The lack of current distinguishing markers further highlights the pressing need to discover more reliable predictors.
Synovial Biopsy
Histological and gene expression studies on biopsies of pre-treatment synovial tissues have enabled the classification of patients into three distinct pathotypes: lympho-myeloid pathotypes (B cells and myeloid cells); diffuse-myeloid pathotype (macrophage-rich but few B cells); and pauci-immune pathotype (few immune cells and high in fibroblasts). Changes in synovial pathotype towards a less inflammatory pathotype (for instance from lympho-myeloid to diffuse myeloid or pauci-immune) are associated with reductions in DAS28, indicating a response to therapy.36 The lympho-myeloid pathotype has been shown to be associated with more aggressive disease and early radiographic progression, and therefore a higher likelihood of progressing on to bDMARDs.37 Consistent with this, a large study on 200 pre-treatment patients (PEAC) showed a significantly higher number of patients in the lympho-myeloid pathotype required biological therapy.38 Interestingly, patients with this pathotype were predicted to require biologic therapy after 12 months, independent of disease duration, contradicting the ‘early window of treatment opportunity’ dogma and indicating that this stratification method has the potential to fast-track patients who are unlikely to respond to first-line therapy on to bDMARDs at disease onset. As well as predicting requirement for bDMARDs, these pathotypes may also be useful in predicting response to bDMARDs. A recent study with 37 participants showed the pauci-immune pathotype had a significantly lower response to the TNFi certolizumab pegol than the other two pathotypes.39 This may indicate that patients with this lower inflammatory pathotype may require an alternative treatment strategy; e.g., ongoing studies are investigating treatment of pain sensitisation in RA.
The use of genetic markers in the synovium may also provide a useful tool in patient stratification. Using RNA sequencing, 3,000 differentially expressed transcripts were found between the three pathotypes in the PEAC dataset, providing greater evidence for these sub-groups.36 The researchers also found genetic differences between patients requiring biological therapy and those who did not. The non-bDMARDs group had an upregulation of fibroblast proliferation and cartilage turnover genes, and patients requiring bDMARDs had a significantly higher upregulation of genes regulating B and T cell proliferation, differentiation, and activation as well as matrix metallopeptidase and cytokine-mediated cellular activation genes.38 Furthermore, higher baseline levels of TNFα in the synovium has been shown to predict response to infliximab in three studies.40-42
These studies outline an exciting method of predicting treatment response at the site of disease, and ultrasound-guided needle biopsy is reportedly well-tolerated with low complication rates.43 Nevertheless, the use of biomarkers obtained from urine and blood are more appealing for entering into clinical use due to ease of testing and repeatability over time in response to interventions.
Blood Biomarkers
There have been extensive research efforts to find biomarkers present in the blood that correlate with treatment response; however, the advantage of the convenience of obtaining these samples may be offset by biomarkers not being detectable away from the site of pathology. For instance, although TNF levels in the synovium have been shown to correlate with treatment response, this signature does not translate to the blood.44 Likewise, in comparison to the 3,000 differentially expressed transcripts between the three pathotypes in the synovium, Lewis et al.36 found just eight differentially expressed transcripts in matched peripheral blood.
These genes were associated with the more inflammatory lympho-myeloid pathotype, with seven associated with Type I IFN response (IFI27, ISG15, IFI44L, OASL, USP18, RSAD2, and LY6E).36 This genetic signature has had previous interest, with some studies finding high levels of this correlates to a lack of response to rituximab.45
Additional blood biomarkers associated with rituximab response have been found through multiple flow cytometry studies, which have demonstrated that higher numbers of circulating plasmablasts are associated with treatment response,46-48 and sensitive flow cytometry analysis of B-cell depletion can indicate the level of response as well as required dose.49
RNA sequencing of whole blood using microarrays has enabled researchers to identify biomarkers of interest and create multi-gene models of response. Using a cDNA microarray, Lequerré et al.50 used eight transcripts to predict 18/20 patients’ response to infliximab successfully. Another eight-gene model was able to predict infliximab response with an 85.7% prediction accuracy in an independent validation set of patients.51 Finally, Tanino et al.52 used an Agilent whole-genome microarray to propose 10 genes that could predict response to infliximab with a 65.4% accuracy.52 Recently, using PrediXcan software, which is more a cost-effective approach than genome-wide genotyping and RNA sequencing, an association between IL18RAP expression and treatment response to TNFis was found.53
Blood cytokine levels can be used as another predictive tool. Increased T helper 17 frequency and T helper 17-related cytokines (IL-17) in the blood, determined by flow cytometry and ELISA, are associated with lack of response from TNFis, which evidences the theory that TNFi resistance may be due to the prevalence of non-TNF pathways.54 Additional cytokines of interest in the serum include IL-33, which has been linked to rituximab response55 and high levels of IL-6 and survivin found to be associated with response to etanercept.56
Furthermore, low serum levels of cartilage oligomeric matrix protein, a protein related to cartilage turnover, have been shown to be associated with response to adalimumab in one study,57 and significantly higher MRP8/13 serum levels were found in responders to three biological therapies, with high baseline levels increasing the odds of being a responder from 3.3 to 55.58
Although these are intriguing findings, many of these results have not been reproduced in other cohorts and are not able to differentiate treatment response at a level amenable to clinical use. The COMBINE study aimed to overcome this problem by using a multi-omics approach, investigating previously identified genetic and proteomic predictors of TNF response to see if these findings could be validated from a large biobank of 451 blood samples.59 They validated 11 predictors from previous studies, which encompassed eight genes from transcriptomics literature, serum levels of soluble intercellular adhesion molecule 1 (sICAM1)/chemokine ligand 13 (CXCL13), and two single-nucleotide polymorphisms (SNPs) (rs6028945 and rs7305646) from genome-wide associated study literature and used these to explain 51% of the variation in change in DAS28-CRP. Interestingly, they found the sICAM1/CXCL13 levels, reported by Dennis et al.,60 were the greatest predictor of response. Previous evidence has highlighted CXCL13 as a marker of interest; e.g., high CXCL13 levels have been suggested to indicate an inflammatory pathotype that may benefit from more aggressive treatment, and high synovium levels of CXCL13 correlate with high levels in the serum.60 Table 2 summarises the predictors of drug response discussed in this article.
Multiple Predictors
Arguably the best approach is one that uses a combination of predictors. Using the PEAC dataset, a regression model was created to predict whether a patient required bDMARDs at 12 months by combining clinical and gene expression level data. The initial model included four clinical covariates: DAS28, CRP, tender joint count, and synovial pathotype, and resulted in a predictive performance of 78.8%.38 Upon addition of genes that were identified as significantly differentially expressed between the biological and non-biological group, the sensitivity and specificity improved to 89% for penalised predictors and 90% for unpenalised clinical predictors.
The Dialogue on Reverse Engineering Assessment and Methods (DREAM): Rheumatoid Arthritis Responder Challenge took a crowdsourcing approach to encourage the development of models that predict a patient with RA’s response to TNFis by making genome-wide associated study data available. Each team’s model was rigorously tested by the Sage-DREAM team to rank the performance based on predefined metrics in a so-called ‘challenge-assisted peer review.’67
The best performing model was a Gaussian process regression model combining demographic, clinical, and SNP array markers. It correctly classified 78% of patients as responders or non-responders to TNFis (with an area under the curve of approximately 0.66) as well as predicting change in DAS28 after 24 months with a Pearson’s correlation coefficient of 0.405. The model relied on the kernel function, which enabled patients to be weighted based on their similarity to a paired patient and provided information on which predictors had the greatest weights in the prediction. Additionally, this model can generate confidence intervals, which may be beneficial for application as a clinical tool. One downside of this model is that it was built using data from European descendants, so may not be translatable to other populations. It is also worth noting that the SNPs only showed a small contribution to the prediction when added to the clinical model.68
The Maximising Therapeutic Utility in Rheumatoid Arthritis (MATURA) consortium was formed in 2014 in response to stratified medicine being identified as a priority by the Medical Research Council (MRC) in 2011. It comprises 12 academic centres and nine industrial partners, and aims to develop tests to predict patients’ responses to MTX, TNFis, rituximab, and tocilizumab. Two research work-streams are underway, one focused on synovial and blood correlates from PEAC and STRAP. The second will use blood samples and clinical information from some of the largest datasets worldwide. These work-streams will be integrated by statisticians to develop algorithms to help clinicians stratify patients.69 Initial results indicate that biomarkers of DAS28 are detectable in genetic, transcriptomic, and DNA methylation data sets. Consistent with the best-performing DREAM model, these have little predictive power when used alone;70 however, this collaborative effort to combine multiple predictors paves a bright future for creating a model with enough predictive power to be used in clinics.
CONCLUSION
The small number of differences found when comparing bDMARDs in terms of efficiency overall may be explained by different bDMARDs being more suited to particular patients, depending on their unique disease pathways. There is no doubt that better biomarkers will improve patient care; unfortunately, we are not yet at a stage where a model is ready for clinical use, due to low predictive values, practical barriers for obtaining certain biomarkers in clinics, and low reducibility between cohorts. Nevertheless, the flurry of recent research efforts investigating predictive tools in multiple domains, including clinical, imaging, pain and function, and blood and urine markers, may allow patient assessment on a more holistic level, providing promise for more personalised care in people with rheumatoid arthritis.