INTRODUCTION
AI is the process of simulation of human intelligence by computer systems. These processes encompass learning, reasoning, and self-correction by machines. Just like the way the human mind learns, in AI, the machine learns through training using algorithms. After the increased use of AI in various fields such as commerce, finance, and hospitality, it has now made its entry into healthcare in a rapid way. AI has emerged as both a current and future tool that brings the promise of finding solutions to the challenges faced in healthcare.1
AI Technologies
AI technologies include machine learning (ML), deep learning, natural language processing (NLP), image recognition, and large language models (LLM). ML algorithms can analyze vast datasets to identify patterns and predict outcomes. NLP facilitates the extraction from unstructured clinical notes and turns it into meaningful information. LLMs are AI systems capable of understanding and analyzing large amounts of texts by mimicking human intelligence. LLMs have been increasingly used more recently with access to Generative Pre-trained Transformer (Chat-GPT, San Francisco, California, USA). The terms and domains used in AI systems are shown in Table 1.
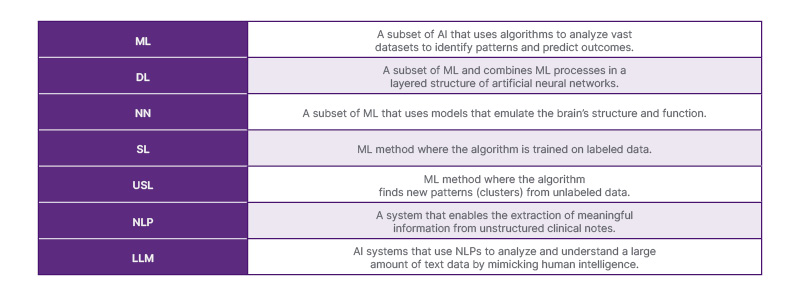
Table 1: Terms and domains in AI.
DL: deep learning; LLM: large language models; ML: machine learning; NLP: natural language processing; NN: neural networks; SL: supervised learning; USL: unsupervised learning.
AI in Rheumatology
AI has been used in various conditions in rheumatology. This includes rheumatoid arthritis (RA), axial spondyloarthritis (axSpA), osteoarthritis, systemic lupus erythematosus, systemic sclerosis, and many others. These conditions often present complex diagnostic and treatment challenges. AI has a role in enhancing the understanding of disease, improving accuracy of diagnosis and treatment of rheumatic conditions, as well as enhancing research. The evaluation of AI includes the assessment of its strengths, weaknesses, challenges, and opportunities.
STRENGTHS OF AI
With the increasing amount of data being recorded on electronic health records (EHR) and health apps in hospitals and primary care settings, there is a need to harness this information to improve the care of patients. ML algorithms can analyze large datasets from EHRs and health registries.1 This allows it to identify patterns and predict outcomes in patients. ML models, for instance, have been used to predict disease activity in RA by analyzing clinical data and biomarkers.2 The predictive capability of ML allows for personalized treatment plans and, in essence, should enhance shared decision-making between patients and healthcare providers as more options are discussed. The use of AI goes beyond objective data as LLMs can also analyze subjective datasets. For example, LLM-driven sentiment analysis has been used for more subjective patient reported outcomes in fibromyalgia.3 This can facilitate diagnosis of fibromyalgia that can be challenging, such as in primary care with shorter appointment times. This LLM-driven approach can detect subtle differences in pain and nuances in patient reported symptoms, aiding the diagnosis of fibromyalgia. AI algorithms can also process and analyze data faster and possibly more accurately than human clinicians. This in the long term will improve patient outcomes.
NLP facilitates the extraction of meaningful information from unstructured clinical notes. It streamlines the information from the patient’s history and referral, which aids in more accurate diagnoses and treatment plans. By analyzing EHRs, NLP can enhance the understanding of disease patterns and treatment responses. Early detection of rheumatic diseases is critical for effective management.
Convoluted neural networks are used for image recognition, such as for radiological images. With the increased demand for imaging in radiology, scans may take much longer for reporting by the radiologist. AI-powered image recognition systems can assist in the interpretation of X-ray and MRI images, identifying joint damage or inflammation with high accuracy. Deep learning has been used to detect inflammatory or structural changes in the sacroiliac joints indicative of axSpA.4 Radiomics is another expanding field that uses AI in imaging. In radiomics, AI systems are used to convert images into high-dimensional, quantifiable data. This is being used, for example, to study prognostic and molecular differences in interstitial lung disease in systemic sclerosis.5 AI systems can reduce the burden on radiologists and improve diagnostic efficiency. AI can also reduce the administrative burden on healthcare providers, allowing them to focus more on patient care. AI can facilitate large-scale analyses of clinical data, enabling researchers to uncover new insights in the pathophysiology of rheumatic diseases and identify novel therapeutic targets. The strengths of AI can be leveraged in the patient pathway at the diagnosis, disease classification, treatment, and monitoring phases. The usage of AI in rheumatology is shown in Table 2.
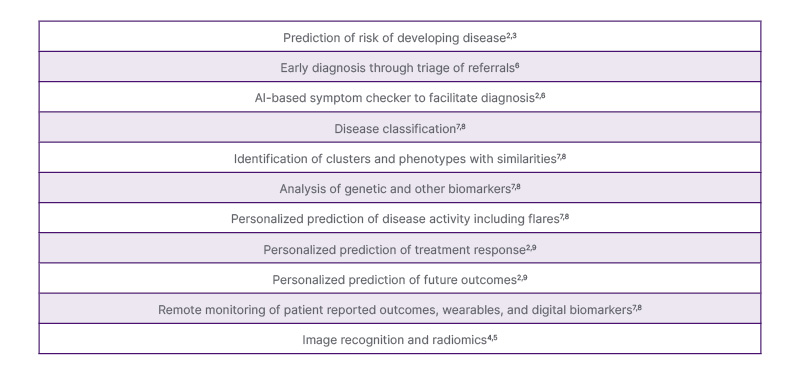
Table 2: The use of AI in rheumatology.
Diagnosis
Early diagnosis can improve long-term outcomes in rheumatic diseases. AI algorithms can analyze data from various sources, including genetic, clinical, and imaging data. The use of EHRs allows the collection of real-world data, including referral information, which can be used to train and validate AI algorithms to predict the probability of inflammatory arthritis such as RA.6
Disease Classification
AI can perform both supervised and unsupervised learning. In supervised learning, the machine learning model is trained based on labeled data. In unsupervised learning, the reverse is done where the machine learning model is trained on unlabeled data. The latter is used to process large databases such as the EHR. This can result in the identification of clusters of patients with similar characteristics or phenotypes. This can improve disease classification.
Treatment Optimization
AI can help tailor treatments to individual patients based on their unique, personalized characteristics. For example, AI has been used to predict giant cell arteritis (GCA) relapse after glucocorticoid (GC) tapering.9 This can aid the clinician in making decisions to taper the GCs slower or increase monitoring in this group of patients with GCA. In RA, deep learning can identify patient clusters that may have different responses to biologic and targeted synthetic disease-modifying antirheumatic drugs.7 By analyzing treatment responses from large patient cohorts, AI can identify which therapies are more effective for specific patient profiles, thereby improving treatment outcomes and minimizing adverse effects.
Patient Monitoring
AI has been used to enhance remote monitoring. As patients are increasingly seen at different time points in clinics based on their disease activity, the data that is provided in between clinic visits can help monitor and predict outcomes. In RA, an adaptive recurrent neural network has been used to predict active disease using the disease activity 28 joint score and blood sedimentation rate (DAS28-BSR) >2.6 in future appointments.8 Post-COVID-19, with the rise in telemedicine, AI has been used in areas such as wearables. These devices equipped with AI can track symptoms and disease activity in real-time, providing healthcare professionals with valuable data to adjust treatment plans promptly.
WEAKNESSES OF AI
Despite its potential to provide rapid insight, the use of AI has its flaws, weaknesses, and shortfalls. AI systems require high-quality, diverse datasets for effective training. The heterogeneity of data sources and patient populations in rheumatology can hinder the development of robustness. Many AI algorithms, such as deep learning models, operate like black boxes, which makes it difficult for clinicians to understand how decisions are made. The AI systems can produce inaccurate and unreal contents, which is known as hallucinations. Clinicians work through problem-solving and refinement of decision-making. The lack of transparency in AI can pose a challenge in clinics where trust and accountability are crucial pillars of good medical practice. The use of AI also raises ethical questions regarding patient data security, privacy, and potential biases in algorithm training. As the system uses large and very diverse content for training, it can be prone to creating biased content. Protecting patient information is key for its successful use. AI systems require large datasets that may include sensitive health information. There is a need to ensure equitable access to AI technologies and address biases in algorithms to avoid exacerbating health disparities. A balance between preciseness and explainability is fundamental for AI. Strategies should be developed to ensure equitable access to AI-driven healthcare solutions, especially in areas of high deprivation. There is also a need to ensure robust data encryption, secure storage, and compliance with regulations that safeguard patient privacy.
CHALLENGES FROM AI
Implementing AI in healthcare, including rheumatology, has ethical and safety challenges. Patients should be made aware of how their data is used, and informed consent must be obtained, particularly if it is being utilized for training AI algorithms. Informed consent must clearly communicate the purpose, potential risks, and benefits of data usage. Determining who is responsible for the decisions made by AI systems is a complex issue of accountability and liability. Clear guidelines are needed to establish accountability in cases of clinical errors, misdiagnosis, or adverse outcomes resulting from AI recommendations. AI may change the dynamics of the healthcare provider and patient relationship. The interaction, communication, and personal connection between both patient and provider need to be enhanced and not diminished with AI.
An ethical dilemma AI poses is the dehumanization of medical actions. Empathy, compassion, and moral subjectivity can only be given by humans to patients. These qualities are essential for clinical care, prevention, and the interaction between a doctor and patient. The use of AI should support rather than replace clinical judgment. There is a risk of a reduction in human critical thinking and reasoning with over-reliance on AI. There are ethical concerns such as AI hallucination and algorithmic bias, which can lead to errors in task management and clinical decision-making.10 On the other hand, AI can enrich various facets of critical thinking when users do not accept AI recommendations without question, analysis, and evaluation of the evidence presented. Healthcare providers should retain the authority to make final decisions regarding patient care. Any output from AI will need human oversight of its use. For this, there must be regulatory frameworks for the use of AI in healthcare. This needs to be continuously monitored and evaluated. Adjustments to AI technologies must meet safety and quality standards. Transparency about how AI systems work and their potential impact on healthcare can improve acceptance by patients and the public. Humans must decide how we use AI ethically, responsibly, and safely.
OPPORTUNITIES FROM AI
Continued efforts are needed to integrate AI into routine clinical workflows. This includes integration of AI within the EHR, interoperability across different software platforms, and interaction with patients through patient portals. Developing user-friendly interfaces that ensure healthcare providers are adequately trained and equipped to use these technologies effectively. Collaborative efforts among rheumatologists, data scientists, and ethicists are essential to address the challenges of AI implementation. Interdisciplinary teamwork can foster innovation and ensure that ethical considerations are prioritized. There will be a need to include informatics and data management as part of the multidisciplinary team. This collaboration and engagement is necessary to provide oversight of AI systems.
FUTURE PERSPECTIVES
There is a bright future for AI in rheumatology, and this will likely focus on a few key areas. AI-driven tools can be used in personalized treatment strategies by analyzing genomic, imaging, and clinical data. AI can also improve efficiency by automating routine tasks such as monitoring disease activity, interpreting blood and imaging studies, and electronic communication. Virtual care and telemedicine platforms powered by AI can expand access to care. With advances in research, AI technologies are poised to evolve alongside. Future research will emphasize longitudinal studies that assess the long-term safety and impact of AI on patient outcomes in rheumatology. Understanding how AI influences disease progression and treatment response over time will be important to optimize its use.
CONCLUSION
AI presents a transformative opportunity in rheumatology. It offers improved diagnosis and personalized treatment and enhances remote monitoring of patients. While the benefits are substantial, addressing challenges related to data quality, interpretability, and ethical considerations is vital for its successful implementation. AI has the potential to revolutionize the management of rheumatic diseases and pave the way for improved patient care and outcomes. The integration of AI in rheumatology is more than a technological advancement. It represents a paradigm shift in the understanding and treatment of rheumatic conditions. Collaboration and innovation will be essential to harness the full potential of AI in rheumatology.