Abstract
Systemic lupus erythematosus (SLE) is a chronic, multi-organ disease that predominantly affects young women of childbearing age. It is also a disease in which epigenetic modulation is emerging as an important mechanism for understanding how the environment interacts with inherited genes to produce disease. Much of the genetic risk for SLE identified in genome-wide association studies has been shown to lie in the non-coding genome, where epigenetic modifications of DNA and histone proteins regulate and co-ordinate transcription on a genome-wide basis. Novel methodologies, including high-throughput sequencing of open chromatin, RNA sequencing, protein microarrays, and gas chromatography-mass spectrometry, have revealed intriguing insights into the pathogenesis of SLE. We review these recent data and their potential contribution to more accurate diagnoses and the development of new therapeutic agents to improve patient outcomes.
INTRODUCTION
Systemic lupus erythematosus (SLE) is a multi-system, complex disease in which the environment interacts with inherited genes to produce a broad spectrum of phenotypes with inter-individual variability. These gene–environment interactions lead to a perturbed immunologic state in which autoantibodies, immune complex deposition, and complement activation contribute to systemic inflammation and target tissue damage. The genetics of SLE have been studied extensively; however, risk loci and single genes identified by genome-wide association studies appear to account for ≤25% of the inherited risk for SLE, suggesting that environment also contributes to risk.1,2 Moreover, most of the genetic risk for SLE lies within non-coding portions of the human genome,3 demonstrating that the disease may manifest due to perturbations in the regulation of transcription, rather than changes to protein-coding genes that lead to nucleic acid substitutions.
The importance of the non-coding genome was demonstrated by recent work by our research group.3 Using standard computational techniques, we queried all the single nucleotide polymorphisms (SNPs) previously shown to convey risk for SLE.1,2 Of the 46 disease-associated SNPs, 30 (65%) were within non-coding regions of the genome. By querying the Roadmap Epigenomics data,4 we demonstrated that most of the linkage disequilibrium blocks containing the disease-associated SNPs were within non-coding regions that were highly enriched for epigenetic signatures associated with functional elements, such as enhancers. These epigenetic signatures were most prominent in B cells and neutrophils and less prominent in CD4+ T cells. These data parallel a recent report by Jiang et al.,5 which demonstrated enrichment for H3K4me1/H3K27ac marks within the linkage disequilibrium blocks containing SNPs associated with juvenile idiopathic arthritis.
The non-coding genome contains numerous functional elements, often identified by specific epigenetic modifications to histone proteins that regulate and co-ordinate transcription on a genome-wide basis.6,7 The critical element in co-ordinating transcription is the regulation of chromatin accessibility, which is regulated by DNA methylation, alterations in histone proteins, and three-dimensional (3D) chromatin architecture mediated by DNA interactions with transcriptional regulators, such as the CCCTC-binding factor (also known as CTCF).8 These processes allow transcription to be fine-tuned to specific physiological circumstances.9 Functional elements that regulate and co-ordinate transcription are typically found in regions of open chromatin,6,7 and both the ENCODE and Roadmap Epigenomics projects focussed considerable efforts on defining these regions.10
Table 1 summarises the most frequently used methods of high-throughput sequencing and mass spectrometry and the information that can be generated using these methods. These methodologies introduce a new era of ‘omics’, allowing for profiling of genetics, proteins, and metabolites to shed light on disease aetiology and pathogenesis. The same techniques that can be used to diagnose patients can also be used to investigate responses to therapy and describe disease courses on molecular levels.
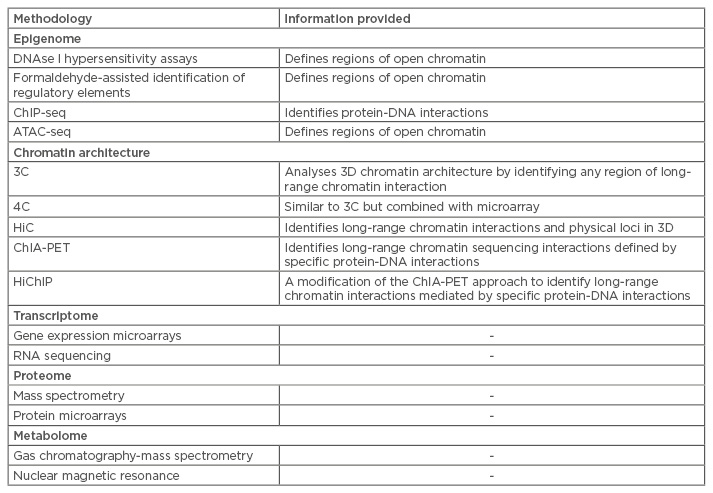
Table 1: Selected methodologies for integrative omics data.
3C: chromosome conformation capture; 3D: three-dimensional; 4C: 3C capture-on-chip; ATAC-seq: assays of transposase-accessible chromatin sequencing; ChiP-seq: chromatin immunoprecipitation sequencing ChIA-PET: chromatin interaction analysis with paired-end tag sequencing; HiC: hydrophobic interaction chromatography; HiChiP: highly integrated chromatin immunoprecipitation.
TECHNOLOGIES AND THEIR USES
Methods used to investigate epigenetic factors that regulate transcription in the context of rheumatic diseases, initially focussed on DNA methylation, are either bisulfate-based (such as MethylC sequencing and reduced representation bisulfite sequencing) or enrichment-based (such as methylated DNA immunoprecipitation sequencing [MeDIP-seq], methylated DNA binding domain sequencing, or methylation-sensitive restriction enzyme digestion followed by sequencing [MRE-seq]) and, as noted, can be combined with sequencing.11,12 Although grossly effective, these methods are not without limitations. In MeDIP-seq, methylated DNA fragments are non-covalently bound to 5-methylcytosine antibodies. Thus, MeDIP-seq does not cover medium–low 5’—C—phosphate—G—3’ (CpG) density regions of the genome well and gives a relatively low resolution, limited by the size of the fragments from immunoprecipitation.12 Moreover, MeDIP-seq requires large amounts of both DNA and antibodies for each assay. MRE-seq and other restriction enzyme-based methods allow interrogation of unmodified and modified areas of genomic DNA, but their coverage and resolution are limited by the specificity of the available enzymes.
Li et al.12 recently suggested that combining DNA methylation and sequencing methods may yield more sensitive results. In particular, the investigators combined MeDIP-seq with MRE-seq to improve the accuracy of detection of differentially methylated regions and coverage of the genome. A key advantage to integration of these methods is that DNA methylation analysis may be performed on a whole genome level and is not restricted to promoters or CpG islands. Moreover, they describe computational protocols to analyse the data generated from both methods that allow them to increase the sensitivity and accuracy of their results.
Traditional methods such as DNAse I hypersensitivity and formaldehyde-assisted identification of regulatory elements assays have been used to identify regions of open chromatin and thus, presumably, regions that are functional and biologically relevant. However, the large number of cells these assays require (often >1×108) to achieve adequate sequencing depth and signal-to-noise ratios have made them impractical for use in translational studies in SLE or other rheumatic diseases. More recently, Buenrostro et al.13 developed a method for broadly surveying open chromatin. This technique, called ‘assays of transposase-accessible chromatin sequencing’ (ATAC-seq), allows researchers to comprehensively survey open chromatin in pathologically relevant cells. By rapidly surveying open chromatin, we may have a comprehensive view of where regulatory elements may be perturbed.14 ATAC-seq uses Tn5 transposases linked to sequencing adapters to selectively insert constructs into nucleosome-free regions, and can be performed on as few as 50,000 cells,15 which makes it highly suitable as a tool to study low-abundance leukocyte subsets. In fact, Scharer et al.16 have described changes in chromatin accessibility that occur at loci surrounding genes involved in B cell activation and differentiation from treatment-naïve adult SLE patients.
Similar advances are being made in techniques to understand how 3D chromatin architecture regulates gene transcription.17 These methods can be either untargeted (chromosome conformation capture [3C], 3C capture-on-chip, hydrophobic interaction chromatography [HiC]) or targeted (chromatin interaction analysis with paired-end tag sequencing [ChIA-PET] and highly integrated chromatin immunoprecipitation [HiChIP]).18-21 Untargeted methods simply map any region of long-range interaction at the chromatin level, but, with the exception of HiC, do so at low resolutions. HiC was developed based on 3C.20 HiC uses 3C to describe not only the genomic sequence of DNA fragments but also where they are physically located in the 3D genomic structure. HiC is also compatible with high-throughput sequencing; this combination identified many long-range interactions between risk loci involved in autoimmune disease and putative target genes in T and B cells.21
Targeted methods investigating chromatin conformation can identify interactions mediated by specific proteins and provide higher resolution maps than untargeted methods. Targeted methods include that described by Li et al.,18 an adaptation of ChIA-PET. ChIA-PET provides high-resolution mapping of long-range DNA interactions mediated by specific proteins. Another method, which the authors designate HiChIP, allows these highresolution maps to be generated with as few as 1×106 cells, or 1% of the number required for ChIA-PET.19
PROGRESS IN UNDERSTANDING THE ROLE OF THE EPIGENOME IN LUPUS
Interest in the role of the epigenome in the pathogenesis of SLE has led to several ground-breaking discoveries looking at DNA methylation.22-24 Coit et al.24 demonstrated, for example, that epigenetic changes in interferon (IFN) response genes seen in adult SLE patients are associated with disease severity. Investigation of DNA methylation in CD4+ T cells also revealed susceptibility loci that may contribute to the differential manifestations of SLE in different ethnicities, including Europeans and African-Americans.22
It is important to note that the epigenetic machinery that regulates gene expression is specific to distinct cell types and that cell-specific expression is a feature of diseases such as SLE.22-24 For example, B cells have essential roles in antigen presentation and cytokine secretion, and produce autoantibodies that are the key to the diagnosis and pathogenesis of SLE. B cell activation and differentiation also correlate with SLE disease activity and response to therapy.25-28 Studies using newer technologies, such as high-throughput sequencing, can also be misleading and/or generate conflicting results. For example, using RNA-seq, Rai et al.29 described dysregulation of specific cytokine pathways in adult SLE patients when stratified by autoantibody profile. IFN transcripts were predominantly dysregulated in patients with only anti-extractable nuclear antigen (ENA) autoantibodies when compared to patients with anti-double-stranded DNA (dsDNA) autoantibodies. Dysregulation of plasma cell-related transcripts were more pronounced in patients with only anti-dsDNA or anti-ENA autoantibodies, when compared with patients who had both sets of autoantibodies. These results conflict with numerous published studies demonstrating that IFN signatures are associated with both anti-ENA and anti-dsDNA autoantibodies,30-32 possibly because Rai et al. performed RNA-seq on total peripheral blood leukocytes.
Although both epigenetic signatures and transcriptomes are cell-specific, new computational approaches have allowed investigators to infer cell-specific patterns even from complex samples, such as whole blood, provided there is information available on the ratios of the different leukocyte subsets. For example, using RNA-seq to examine transcriptomes in whole blood samples, Dozmorov et al.33 used a de-convolution method that allowed them to identify differential expression of immunoglobulin (Ig) genes in SLE B cells, while a monocyte population from the same patients differentially expressed genes comprising a ribosomal signature (Table 2).
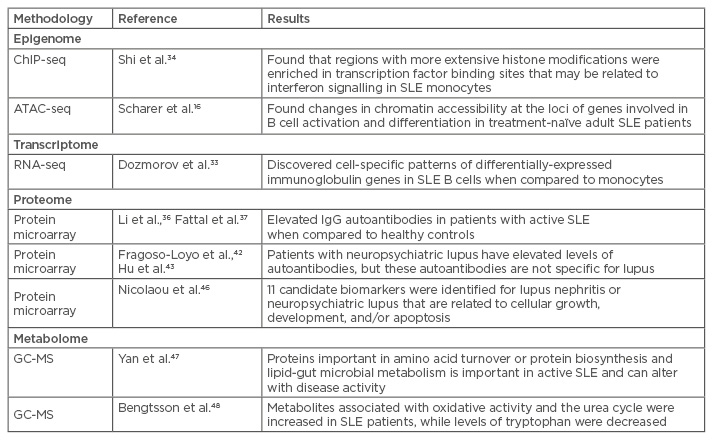
Table 2: Use of omics in lupus to date.
ATAC-seq: assays of transposase-accessible chromatin sequencing; ChIP-seq: chromatin immunoprecipitation sequencing; GC-MS: gas chromatography-mass spectrometry; NPSLE: neuropsychiatric lupus; SLE: systemic lupus erythematosus; Ig: immunoglobulin.
De-convolution methods have not been attempted with histone marks, and cell-specific studies continue to be the standard approach. For example, Shi et al.34 used chromatin immunoprecipitation sequencing (ChIP-seq) to define histone modifications in monocytes of adult SLE patients. ChIP-seq analyses protein interactions with DNA through the genome-wide DNA binding sites for transcription factors and other proteins (e.g. histones). Compared to healthy controls, regions with more extensive histone modification were significantly enriched in transcription factor binding sites that may be related to IFN signalling in adult SLE monocytes. Taken with the information from B cells as described above, these data could help to direct research efforts toward new avenues of therapy targeting different cell populations and intracellular signalling pathways for specific clinical manifestations.
PROTEOMICS AND METABOLOMICS
The transcriptomes and epigenomes of SLE patients only provide a small window into disease pathogenesis and possible response to therapy. Personalised medicine is expected to benefit from the combination of genomic information with regular monitoring of physiologic states by multiple high-throughput methods that query a broad range of cellular processes. Novel approaches using mass spectrometry enable a closer look at the proteome and metabolome (the composition of all small molecule metabolites in human cells).
Protein, or ‘autoantigen’, microarrays allowing for detection of autoantibody profiles in SLE were described over 10 years ago.35 These arrays carry thousands of proteins that can be found in many rheumatic diseases, including SLE. The arrays also allow for detection of antibody isotypes (IgG, IgM, IgE, and IgA). The advantages of microarrays over mass spectrometry for protein profiling are the ability to analyse low abundance proteins and that microarrays are not as time-consuming or labour-intensive to perform. Recently, protein microarrays were used to detect proteomic profiles correlating with specific disease manifestations of SLE. Microarrays were used to distinguish between adults with lupus nephritis, neuropsychiatric lupus (NPSLE), and pulmonary involvement.36-39 Li et al.36 found that SLE patients had increased levels of IgG autoantibodies in their sera. Combining these findings with transcriptional profiling using conventional hybridisation-based microarrays revealed a correlation with elevated expression of IFN genes, indicating that IFN may play a role in class switching of IgM to IgG antibodies in SLE. Fattal et al.37 found increased levels of IgG autoantibodies against dsDNA, single-stranded DNA, Epstein–Barr virus, and hyaluronic acid in the sera of patients with active lupus nephritis when compared to healthy controls. Although Fattal et al. found these levels remained high even after the patients achieved long-term clinical remission, indicating independence from disease activity, much evidence using traditional assays has established that anti-dsDNA antibody levels do fluctuate with disease activity and remission.38-41
Fragoso-Loyo et al.42 found elevated levels of autoantibodies using protein microarrays in the sera of patients with NPSLE. However, these autoantibodies could be seen in other rheumatic diseases; none were specific for NPSLE. Hu et al.43 used a protein microarray with 17,000 distinct proteins to evaluate NPSLE sera. These experiments identified 137 autoantigens (including auto antibodies) associated with SLE. Two of these proteins, anti-60S acidic ribosomal protein P2 and anti-SSA in cerebral spinal fluid (CSF), were significantly correlated with those in sera of NPSLE patients. The findings suggest CSF proteins are potential biomarkers for NPSLE, but there have been conflicting studies.44
There remains a definite challenge in finding biomarkers for pulmonary diseases associated with SLE. Protein microarrays for a broad range of cytokines and chemokines were performed on sera from nine adults with SLE who had known pulmonary involvement. Data were compared from nine adults with SLE without pulmonary involvement.45 A significant increase in CC chemokine ligand 21 (CCL21) and IFN-gamma induced protein 10 (IP-10) levels were seen in patients with SLE and pulmonary involvement. The changes in CCL21 and IP-10 were associated with changes in diffusion capacity of those same patients, indicating that these chemokines may serve as biomarkers for pulmonary disease in patients with SLE.
A systematic review of the published reports on proteomic biomarkers discovered by mass spectrometry-based methods in adult SLE patients found that ≤28 candidate biomarkers had been validated in the laboratory. Eleven candidate biomarkers were identified in more than one study.46 Many of these biomarkers are thought to be significant in the diagnosis of lupus nephritis or NPSLE. The functions of the biomarkers appear to be related to maintenance of cellular functions such as growth, division, and apoptosis. However, to date, these biomarkers require further study to assess their clinical utility and significance in clinical practice.
Metabolomes have been profiled from the sera of a cohort of 80 Chinese adult SLE patients using gas chromatography-mass spectrometry.47 This analysis revealed that proteins associated with changes in amino acid turnover or protein biosynthesis, and lipid and gut microbial metabolism, might act as a ‘metabolic signature’ in SLE patients. This study also demonstrated that metabolomes varied with differences in disease activity. These alterations predominantly involved metabolites such as glutamate, citrate, linoleic acid, and prophylparaben.
Another group48 compared adult SLE patient metabolomes with those from patients with primary Sjögren’s syndrome and systemic sclerosis. These investigators found an increase in the circulating abundance of metabolites associated with oxidative activity and the urea cycle in SLE patients.41 SLE patients also had decreased levels of tryptophan compared to those with Sjögren’s syndrome or systemic sclerosis. These findings suggest that SLE changes the enzyme activity of a decarboxylase and/or activation of the kynurenine pathway, which may be a novel metabolic checkpoint in the pathogenesis of SLE.49 These data suggest new ways of treatment by targeting small molecule metabolites and biosynthesis pathways, in addition to the more traditional methods of targeting immunologic pathways.
Nuclear magnetic resonance has been touted as a new and emerging technique for investigating the metabolome; it is faster, less labour-intensive, and does not require as many separations to obtain data as gas chromatography-mass spectrometry. Nuclear magnetic resonance can also measure up to hundreds of metabolites at once. However, there are no studies to date using this technique in SLE.
AUTHORS’ PERSPECTIVE
The emergence of this new era of omics and personalised medicine in rheumatic disease is exciting. The newer methodologies to examine the epigenome, transcriptome, proteome, and metabolome will generate previously unimagined amounts of data about health and disease states. These new methodologies also allow for more innovative and comprehensive approaches to pathobiology, prognostication, and therapy. The development of the era of personalised medicine, where the wealth of information available from omics data may be applied to the treatment of individual patients, has the potential to dramatically improve patient outcomes.
Over the past decade, information from the genome and epigenome has allowed us to more accurately diagnose and treat cancer patients in a manner uniquely suited to each individual.50 Similar advances may soon be applied to rheumatic disease using results generated from high-throughput sequencing methodologies. When combined with clinical disease correlations, this approach may facilitate monitoring of disease phenotypes. In particular, data generated from the epigenomes and transcriptomes of patients with SLE, combined with proteomics and metabolomics, may allow us to predict how time and treatment alter the natural history of the disorder. This combination may also enhance diagnosis and treatment while improving epidemiologic data on SLE and other human diseases.51 A long-term goal will be to tailor therapies based on individual patient characteristics and more accurately monitor individual responses to individualised therapies, thus improving individual patient outcomes.
However, enthusiasm for personalised medicine and the future of omics in rheumatic disease must be tempered with a word on costs. Currently, these methods are for laboratory and research purposes only, and are not available for clinical use. Each array or sequencing assay is for single use and typically costs thousands of dollars. Data generated from these arrays and assays may result in files that are hundreds of megabytes; data from several patients would require terabytes (1012) of storage on a computer with at least a 2.7 GHz microprocessor. Not only are the datasets large, they can be quite time-consuming to analyse. They require interpretation first by experts in bioinformatics and then careful clinical correlation to specific diseases by subspecialists with a keen understanding of the underlying mechanisms of disease pathogenesis and progression. In addition, there is the added challenge of integration of data from different sources and platforms that requires the development of more sophisticated, robust bioinformatics tools.
Moreover, there is rising concern over privacy issues with the deposition of genomic data into public cloud computing settings.52-54 While accessing and integrating genomic data with clinical phenotypes are important for research, these processes must be handled carefully to avoid inadvertent leakage of sensitive information to unauthorised persons and the improper use of available data. When data are shared between multiple institutions, there is additional concern about data being used beyond agreed-upon research scope and potential processing in unsafe computational environments. Establishment of rules and regulations in this field to protect the donor as well as the user of readily available genomic data will greatly support and enhance the use of these technologies in the future. Combinatorial omics data from SLE patients may give us new ways to subset SLE patients. This will allow for better efficacy in clinical trials requiring a smaller number of patients. Again, we must emphasise that the power of these high-throughput sequencing techniques in SLE appears to be in the provision of ways to advance therapeutics through analysis of earlier responses to therapy in distinct SLE clinical phenotypes and subsets of patients. As most studies are currently, and will continue to be, conducted on patients with long-standing SLE, the use of omics may reflect their disease course. Additionally, some patients may have received heavily immunosuppressive or ablative therapies that will affect the results from omics methodologies. Care must be taken to analyse the correct tissue types and cellular populations. SLE patients may experience a constant low-level of inflammation; omics data may lead us to discover new therapies that could return the genome to ‘normal’ in specific disease remission states and thus improve patient outcomes by reducing the burden of disease.