Meeting Summary
This industry-supported symposium was held during the European Respiratory Society (ERS) International Congress and included presentations from several internationally renowned experts in rare lung diseases. The panel discussed the need to improve clinical decision making to expedite disease recognition, prognostic prediction, and early treatment in interstitial lung disease (ILD) and alpha 1 antitrypsin (AAT) deficiency-related chronic obstructive pulmonary disease (COPD).
Daiana Stolz, Clinic for Pneumology, University Hospital Freiburg, Switzerland, and Marlies S. Wijsenbeek, Erasmus University Medical Centre, Rotterdam, the Netherlands, explained that although high-resolution CT (HRCT) scans may appear similar, ILD from different causes results in significantly different patient outcomes. Therefore, image analysis and the identification of sensitive and specific biomarkers are critical to improving diagnosis and monitoring treatment response and disease progression in ILD.
Charlie Strange, Medical University of South Carolina, Charleston, USA, and Gerry McElvaney, Irish Centre for Genetic Lung Disease, Dublin, Ireland, described the variability in CT-based lung density measurements used to assess the progression of emphysema in patients with AAT deficiency. Clinical trial data indicate that accurate CT lung density measurements are superior to lung function measurements and other endpoints to detect disease progression. However, Strange presented data that showed the considerable impact of acute exacerbations of COPD on CT imaging measurements.
One organisation working to improve the accuracy and value of imaging data in lung diseases is the Open Source Imaging Consortium (OSIC), Saugatuck, Michigan, USA. Elizabeth Estes, who works for OSIC, explained that OSIC aims to build a large, global database of anonymised patient data and CT images in ILD, with plans for future expansion into other rare lung diseases. The ultimate goals of this effort are to encourage collaboration, and to develop machine learning algorithms to improve clinical decision making in rare lung diseases.
The Challenges of Diagnosis and Differentiation in Interstitial Lung Disease
Daiana Stolz and Marlies Wijsenbeek
Stolz explained that complex cases of ILD that are difficult to diagnose are often encountered in a tertiary care pulmonary clinic. Stolz highlighted that two cases of interstitial lung disease (ILD) may appear similar from HRCT, showing lung opacities and interstitial reticulation, yet they can have dramatically different disease course and survival outcomes.
Therefore, there is an unmet need to improve diagnosis and differentiation in ILD through the use of advanced imaging techniques. Stolz stressed that such techniques provide hope for improved disease recognition, prognostic prediction, and expedited treatment. The ultimate aim for such improvements is to deliver personalised medicine by being able to forecast individual therapeutic responses.
As an example, Wijsenbeek described a clinical case of a 52-year-old patient who presented with shortness of breath during mountain climbing in 2017. The following year, the patient had developed a cough and their symptoms persisted. Over the next 3 years, they had five different diagnoses at six different institutions, including dyspnoea on heights, pneumonia, sarcoidosis, idiopathic pulmonary fibrosis (IPF), and fibrotic hypersensitivity pneumonitis (HP). Following diagnosis with HP, a treatment with prednisone was started, which reduced the symptoms of cough. However, the patient’s pulmonary fibrosis was progressing despite management, and they were started on anti-fibrotic treatment. Further diagnostic work-up at the Erasmus Medical Centre, Amsterdam, the Netherlands, revealed a pathogenic variant of the PARN gene and a very short telomere length (below the first percentile). The multidisciplinary team made the diagnosis of HP with progressive pulmonary fibrosis in the context of a pathogenic variant of the PARN gene. The patient’s condition continued to decline, and they were identified as eligible for lung transplantation. Following transplantation, the patient is now doing reasonably well.
Wijsenbeek highlighted that the patient’s medical history illustrates the diagnostic delays often associated with ILD. On average, it takes seven months for ILD to be diagnosed, with nearly one-third of patients (29%) waiting for 2 or more years for a diagnosis.1 More than one-half (55%) of patients with ILD are misdiagnosed, and many (38%) are misdiagnosed more than once.1
Improvement in timely and accurate diagnosis is an important unmet need identified by patients with pulmonary fibrosis.2 Studies have shown that patients with IPF diagnosed in the first year after their symptoms develop live longer than those diagnosed later.3 Short telomere lengths have also been associated with worse outcomes, particularly in patients receiving immunosuppression therapy.4,5
In a panel discussion during the symposium, Wijsenbeek explained that one of the advantages of large data repositories, such as that being developed by the Open Source Imaging Consortium (OSIC),6 is the ability to aggregate data. Wijsenbeek stressed that, in addition to imaging and physiological parameters, factors such as symptoms, quality of life, and patient preferences are also important to consider, and these data can also be included in repositories.
The Open Source Imaging Consortium: A Renaissance Approach to Imaging in Rare Lung Diseases
Elizabeth Estes
OSIC is a global, not-for-profit, co-operative effort between academia, industry, and patient advocacy groups, created to enable radical advancement in early diagnosis, disease course prediction, and disease management in ILD. To achieve these goals, OSIC aims to build the largest, most diverse, global, anonymised ILD database, with the aim of identifying meaningful biomarkers, encouraging the collaboration of competitors in the field, and enabling the cooperation of specialists such as radiologists, pulmonologists, and computer scientists (Figure 1).6,7
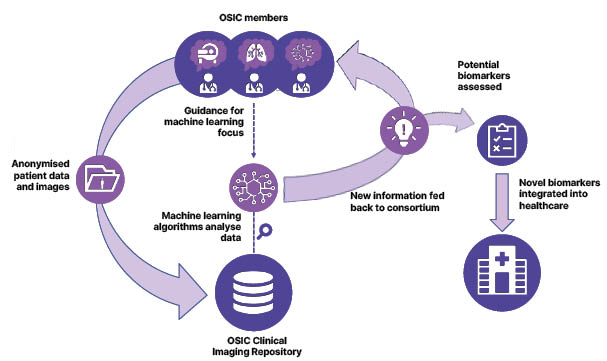
Figure 1: An overview of the process through which OSIC aims to improve diagnostics, prognostics, and prediction in rare lung diseases.6,7
OSIC: Open Source Imaging Consortium.
The Renaissance period of European history cultivated changes in the way people thought by blending together the fields of art, architecture, and science.8 Estes explained that OSIC used the Renaissance as the inspiration for their approach, anticipating the breakthroughs that could be made through the collaboration of specialists that otherwise work independently in ILD.
OSIC is led by Kevin Brown, Simon Walsh, and David Barner, experts in pulmonology, radiology, and computational science, respectively.6 Consortium members include diverse experts from the pharmaceutical and medical device industries, hospitals, universities, and patient advocacy groups.6
One of the greatest challenges for OSIC so far is to ensure the adequate protection of patient privacy and data integrity. To meet this challenge, OSIC has been designed to be fully compliant with the European Union (EU) General Data Protection Regulation and the USA’s Health Insurance Portability and Accountability Act (HIPPA).6 All data is anonymised prior to upload, quality control testing is applied at multiple levels, and the platform is continuously monitored for potential flaws or security issues.6
The initial target of OSIC was to include a minimum of 15,000 CT scans in the ILD database, and Estes confirmed that this threshold has already been exceeded. Machine learning algorithms will be developed from this data to incorporate this information into commercial analysis tools for imaging, treatment, or therapeutics (Figure 1).6,7 The ultimate goal is to enable early diagnosis, enhanced prediction of disease prognosis, and improved disease management in ILD.6,7
As of September 2022, the OSIC database contained more than 3,000 scans from a total of more than 2,000 patients, including >80,000 data points such as smoking status, age, and mortality. Initially, Estes explained, OSIC working groups looked for patterns in forced vital capacity decline, which turned out to be difficult because of the noise-to-signal ratio in this endpoint. However, survival analysis for IPF using the clinical data and images from the database revealed that specific characteristics of the pulmonary vasculature were associated with increased mortality.6 This finding suggests that prognostic markers could be developed from these characteristics, and Estes emphasised that this potential was being openly discussed and investigated among OSIC collaborators.
Survival analysis data were also made available to more than 2,500 machine learning experts worldwide in a crowd-sourced challenge to identify what could be extrapolated from the data.6 In addition to changes in the pulmonary vasculature, the extent, patterns, and degree of pulmonary fibrosis was identified as an important prognostic factor in IPF.6
OSIC is currently working to bring a new patient cohort into the database that will be followed prospectively, as part of the OSIC Project OPUS.6,7 This study is supported by the European Idiopathic Pulmonary Fibrosis and Related Disorders Federation (EU-IPFF), the American Lung Association, and Action for Pulmonary Fibrosis (UK), and recruitment will begin in Spring 2023. The initial target recruitment is 100 patients whose medical records, X-rays, and HRCT scans reaching back for 2 years, which will be uploaded. For prospective data collection, patients will be provided with the patientMpower (Dublin, Ireland) application, which will enable home spirometry, patient-reported outcomes, geographic location, and weather data to be recorded on a daily basis.
Estes stressed that in these early stages of the OSIC repository, it is important to understand what data is meaningful. OSIC’s approach is to set up a core dataset and ask collaborators to recommend additional data they feel would be valuable. Estes reiterated that the OSIC aims to understand which analyses do and do not work to improve lung disease diagnosis and prognosis and make these findings available globally.
The initial goal for implementing OSIC learnings is to provide immediate decision support to clinicians, for example, through an algorithm integrated into the picture archiving and communication system used by radiologists. Since HRCT imaging machines and the expertise required to interpret CT scans is not available in all parts of the world, it can be difficult for clinicians to identify subtle disease characteristics from these images. Estes stressed that machine learning algorithms could make far greater use of the data contained within each image and may be able to identify patterns that a human cannot.
OSIC is working to increase the number of control CT scans in the database, to support analyses using artificial intelligence (AI), and to including X-rays and data for lung diseases beyond IPF, such as alpha 1 antitrypsin (AAT) deficiency, bronchiectasis, rheumatoid arthritis-associated ILD, and sarcoidosis.
Estes reiterated that clinicians should consider OSIC a resource for their potential needs or ideas, with the ultimate goal of making radical progress on behalf of patients, by getting solutions to clinicians at the patients’ bedside.
Exacerbations in Alpha 1 Antitrypsin Deficiency: Impact on Lung Density
Gerry McElvaney and Charlie Strange
Lung densitometry uses CT scanning to assess the X-ray attenuation of pulmonary tissue, reflecting both the degree of inflation and structural lung abnormalities. Decreased attenuation is commonly seen in emphysema and cystic diseases, and increased attenuation suggests infiltration, inflammation, or fibrosis. Strange explained that lung density is best explained by a CT density histogram, in which a shift of the 15th percentile point (PD15) captures the transition from a healthy lung to an emphysematous lung (Figure 2).9,10
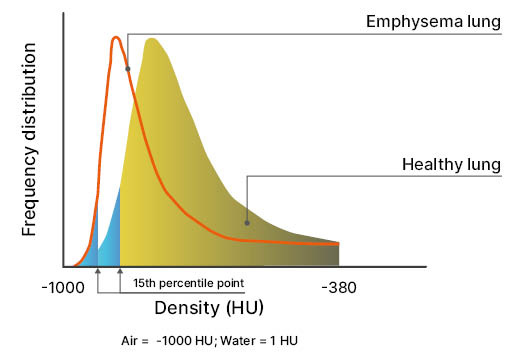
Figure 2: Lung density, assessed through thin slice CT acquisition, is the most direct measure of pulmonary emphysema.
The 15th percentile point is the density value that covers 15% of all densities of the histogram.
Adapted from Stolk et al.10
The RAPID clinical trial programme assessed the effects of an alpha 1 proteinase inhibitor (A1PI)-augmentation treatment on the change in lung density in patients with severe AAT deficiency.9
In 2017, the results were published from a 24-month randomised controlled trial on the long-term safety and efficacy of A1PI treatment for emphysema caused by AAT deficiency (RAPID-OLE).9 The results showed a decrease in the rate of decline in PD15 (normal: 80 g/L) at total lung capacity in patients receiving an A1PI compared with those receiving placebo (‑1.51 g/L per year; n=140).9 Strange explained that this finding indicated that less lung tissue was lost over time in patients receiving AAT versus placebo. In the open-label extension of the trial, patients receiving placebo switched to A1PI, resulting in a significant reduction in the rate of decline of lung density in these patients over the following 24 months.9 Strange emphasised that these data suggest that A1PI augmentation treatment is capable of preserving lung density.
However, Strange explained that variability is often observed in the PD15 lung density measurements of individual patients with AAT deficiency during the progression of emphysema. Strange explained that lung density decline does not always follow a straight line in these patients, suggesting that there must be variables that affect lung density at different time points. One potential variable is the occurrence of acute exacerbations of COPD.
On average, patients with AAT deficiency experience one or two acute exacerbations of COPD per year,11 and each event can last up to 2 weeks. During exacerbations, pulmonary function changes include worsening expiratory flow, increased residual volume, and reduced inspiratory capacity.12 A study by Parker et al.13 showed it takes about 60 days (approximately 2 months) for pulmonary function to return to normal following an acute COPD exacerbation.
To investigate the influence of patient characteristics and acute exacerbations of COPD on PD15 lung density values, a post hoc analysis of the RAPID-OLE data was recently performed (Strange et al., unpublished data).14 Strange explained that in this study, regression lines were generated for each patient to provide expected PD15 values at each timepoint, and the distance from the expected versus the observed PD15 value (the ’residual’) was calculated for each CT scan (Figure 3). The impact of patient baseline characteristics and the temporal proximity to a preceding acute exacerbation of COPD, on the difference between expected versus observed PD15, were investigated.14
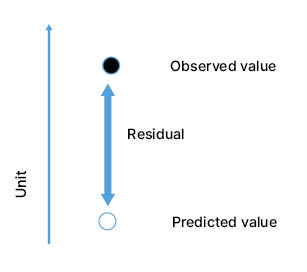
Figure 3: Representation of the residual value, calculated from the expected and predicted 15th percentile point values.
Strange emphasised that while some of the deviations from the expected PD15 following an acute exacerbation event showed a lung density below the predicted value, some showed an increased density. McElvaney explained that clinicians observe different effects of emphysema in different parts of the lung. In the same lung region, some patients will have mucous impaction of airways, some will show the presence of inflammatory cells, and some will have distal hyperinflation. This clinical observation reinforces the idea that CT scans in individuals with AAT deficiency show heterogenous changes that cannot be reduced to a single pattern of emphysema on a CT image.
The presence of a recent acute exacerbation was found to be the most influential factor for large deviations from the expected PD15 value for lung density measurements.14 Strange and McElvaney believe that AI will be helpful in decreasing variability between CT scans, and in connecting those outcomes that are particularly important to patients, with the CT-associated measurements associated with their symptoms. They stressed the importance of identifying factors introducing bidirectional deviations from the expected PD15, as this can help to minimise the number of patients needed for a clinical trial.
Overall, the RAPID-OLE trial data indicate that accurate CT lung density measurements should become the gold standard for evaluating emphysema progression in COPD.11,14 McElvaney emphasised the need to increase the use of CT scans in patients with AAT deficiency and COPD and explained that this would require changes to radiation protocols and other radiographic modalities.
Further studies are needed to reach the full potential of lung density measurements in these patients, and McElvaney suggested that we need to answer a series of key questions (Box 1).

Box 1: Key questions need to reach the full potential of lung density measurements.
AAT: alpha 1 antitrypsin; COPD: chronic obstructive pulmonary disease.
McElvaney explained that there is considerable heterogeneity in disease parameters in patients with AAT deficiency-related COPD, and that more detailed analyses of CT scans could help to differentiate patients. For example, analysis tools should be refined to include measures such as regional lung density. McElvaney stated that it would be interesting to leverage the OSIC repository to analyse whether the pattern of emphysema progression in AAT deficiency, which generally starts in the lower lung and spreads upwards, differs from other types of emphysema, or between genetic subtypes of AAT deficiency.
In addition, McElvaney stressed that the current markers for acute COPD exacerbation rely on old criteria and patient-reported symptoms; there is a need to improve biomarkers for these events. McElvaney suggested that the OSIC repository might be useful to relate CT scan data during (or immediately after) an exacerbation and relate it to other disease parameters, such as pulmonary function and biomarkers. Strong evidence of this type could be used to support changes in clinical practice guidelines.
Closing Remarks
It is clear that timely and accurate diagnosis is a key unmet clinical need for patients with ILD. Visual inspection of lung CT images permits the assessment of multiple structural changes in the lung, forming an integral part of diagnosing the specific form of ILD, which is a prerequisite to tailoring a treatment regimen for the individual patient. However, sensitive and specific biomarkers are also needed to improve diagnosis, assess treatment response, and determine disease prognosis.
The imaging clinical data repository being developed by OSIC will initially focus on ILD, though this repository plans to expand into other lung diseases such as AAT deficiency in the future. Machine learning algorithms applied to such a substantial collection of data promises to identify new patterns and associations in rare lung diseases, and one that has the potential to be enhanced by AI.
In COPD that is associated with AAT deficiency, a better understanding of the impact of diverse parameters on CT assessment of lung density will help improve this technique’s accuracy. When expanded to include conditions beyond ILD, machine learning algorithms applied to the OSIC repository offer the potential to reveal novel information in this arena.
The expert discussions presented in this symposium indicated that the assessment of rare lung diseases should aim to combine data from imaging, physiology, clinical characteristics, and disease progression. AI may support the analysis of these data, increasing the precision and accuracy of diagnosis and prediction of patient prognosis. Imaging biomarkers may represent one of the next generations of biomarkers in lung disease. This is a field that needs to be developed so that it can be of value to patients, clinicians, and regulators, and so that new biomarkers can be included in the future design of clinical trials in rare lung diseases, including ILD and AAT deficiency.