BACKGROUND AND AIMS
Idiopathic pulmonary fibrosis (IPF) is inexorably progressive. Like IPF, other fibrotic lung diseases can exhibit this progression, and these are called progressive fibrotic phenotypes. Early identification of these phenotypes is crucial to initiate treatment promptly.1-4
The severity of traction bronchiectasis, when visually assessed, is a strong predictor of mortality in patients with fibrotic lung disease. However, semi-quantitative methods have limitations, which can be addressed by the application of quantitative methods.5-7
The objective of this investigation is to explore the prognostic utility of a novel deep-learning algorithm in quantifying the severity of traction bronchiectasis in patients with IPF, who are enrolled in the Australian IPF Registry (AIPFR).
MATERIALS AND METHODS
Visual evaluation of high-resolution CT (HRCT) from the AIPFR was performed by two expert thoracic radiologists evaluated. Total airway volume (TAV) was quantified using a novel 3D U-Net-based deep-learning algorithm. Systematic objective fibrotic imaging analysis algorithm (SOFIA) usual interstitial pneumonia (UIP) probability scores were obtained using a previously reported deep-learning algorithm, trained in the identification of UIP features.5 The prognostic utility of automated airway volume quantification was evaluated against conventional measures of disease severity and SOFIA-based UIP probability scores.
RESULTS AND DISCUSSION
In total, 217 HRCTs were amenable to algorithmic airway volume quantification. TAV was an independent predictor of mortality when controlling for visual-based evaluation of total fibrosis extent (hazard ratio [HR]: 1.96; p<0.0001), % predicted forced vital capacity (FVC; HR: 2.15; p<0.0001), or the composite physiologic index (CPI; HR: 1.52; p=0.02). On bivariable analysis, both TAV (HR: 2.13; p<0.0001) and SOFIA-UIP probability (HR: 1.30; p<0.0001) independently predicted mortality. Patients were divided based on INBUILD criteria (Group 1: ‘UIP-like fibrotic pattern’, HRCT showing UIP or probable UIP, or surgical lung biopsy showing possible, probable, or definite UIP; and Group 2: ‘other fibrotic patterns’, remaining patients).1 On bivariable analysis with total fibrosis extent, TAV independently predicted mortality in Group 1 (HR: 1.50; p=0.03), and was the only predictor of mortality (HR: 5.33; p<0.0001) in Group 2. An increase in TAV of 1% of total lung volume was associated with a three-fold increased likelihood of developing progressive disease (FVC/diffusing capacity of the lungs for carbon monoxide decline of 10% and 15%, respectively, at 12 months; odds ratio: 3.04; p=0.009) when controlling for total fibrosis extent.
IPF exhibits an unpredictable and progressive clinical course. This means that, despite treatment, IPF is inherently progressive, akin to other interstitial lung diseases categorised as progressive phenotypes. Recognising progression is crucial to avoid delaying the onset of treatment and preventing clinical deterioration, ensuring timely intervention for the disease.
For IPF, progression is typically defined as a 10% or greater decline in FVC, or a 15% or greater decline in diffusing capacity of the lungs for carbon monoxide over 12 months, or sustained over 18 months, or in cases of death or transplantation.8,9
FVC is the most widely accepted marker and primary endpoint in clinical trials. However, it has limitations, like data loss, variability, and susceptibility to the influence of emphysema, prompting the exploration of alternative markers.
CT holds promise as a prognostic marker. Traction bronchiectasis has proven to be a powerful sign of progression. Yet, most studies evaluating them rely on semi-quantitative methods, which are susceptible to low reproducibility and inter-observer variability, and are unable to capture subtle changes over a short timeframe.5-7,10,11
TAV objectively identifies patients at risk of progression, aiding clinical decision-making and stratification of patients in clinical trials. Compared to FVC, TAV is a reliable short-term evaluation method (Figure 1).
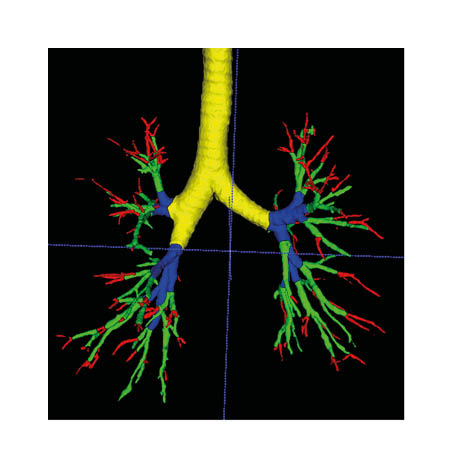
Figure 1: Segmentation of the airway.
The terminal bronchus is shown in red, the small bronchus in green, the medium bronchi in blue, and the large bronchi in yellow.
CONCLUSION
In IPF, automated quantification of TAV predicts mortality independently of total fibrosis extent on HRCT, and can be used to identify patients at risk of progression at 12 months.