BACKGROUND AND AIMS
While prebiopsy multiparametric MRI (mpMRI) substantially improves detection of clinically significant prostate cancer (csPCa) and reduces unnecessary biopsies and diagnoses of insignificant cancer, there remain ongoing challenges with underdiagnosis, biopsy rates, and overdiagnosis. Major studies indicate that 21–49% of patients may still undergo a potentially avoidable biopsy,1-4 and up to 12% of csPCa may be missed.5
Artificial intelligence (AI) has the potential to support, and improve accuracy and consistency of, clinical interpretation of prostate MRI. This study compares a new AI-based system for detecting Gleason ≥3+4 csPCa using MRI, with human readers and existing computer-aided diagnosis (CAD) literature.
MATERIALS AND METHODS
An AI-based system was developed using a proprietary, multistage architecture designed to produce prostate segmentation for prostate-specific antigen density estimation and fusion biopsy, cancer risk calculation to help reduce unnecessary biopsies, and lesion identification to support biopsy targeting.
Data was obtained from open source, anonymised prostate MRI datasets and divided into training, development validation, and held-out test sets. Segmentation models were trained on axial T2-weighted imaging MRI data and accompanying prostate annotations, from the PROMISE12 and NCI-ISBI 2013 Challenge datasets,6,7 acquired using a variety of 1.5T and 3T scanners. Models for cancer assessment (risk calculation and lesion identification) were trained on PROSTATEx,8 an mpMRI dataset acquired at one centre on two 3T scanners (MAGNETOM Trio and MAGNETOM Skyra, Siemens, Munich, Germany), with histopathology findings from MR-guided biopsy as ground truth.
Accuracy was evaluated on development validation and held-out test sets and compared with literature on radiologist interpretations and similar AI/CAD approaches.
RESULTS
As a prebiopsy rule-out test, the system identified patients with Gleason ≥3+4 csPCa with sensitivity 93% (95% confidence interval: 82–100%), specificity 76% (64–87%), negative predictive value (NPV) 95% (88–100%), and receiver operating characteristic area under the curve (AUC) 0.92 (0.84–0.98), using axial biparametric MRI (bpMRI) data from the PROSTATEx combined development validation and held-out test sets (80 patients; 35% csPCa prevalence). Performance was higher on the 40 patient held-out test set. Performance results for the model using axial mpMRI data were similar. Radiologists at Likert/Prostate Imaging Reporting and Data System 3 (PI-RADS 3) thresholds achieved per-patient sensitivity of 88–93%, specificity of 18–68%, and NPV of 76-97%.1,3,4 Comparable AI/CAD publications report 93% sensitivity using held-out or blinded test data at specificity ranging from 6% to 42%.9,10 The achieved results, including mpMRI data, are summarised in Table 1.
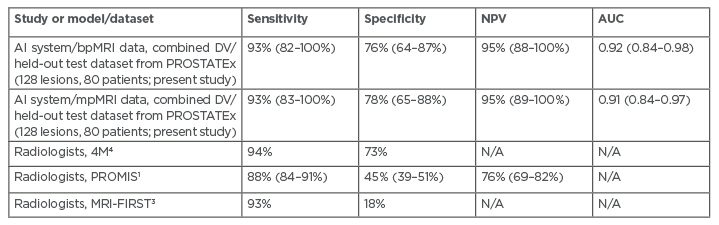
Table 1: Performance of the proposed AI system for identifying patients with Gleason ≥3+4 cancer, compared with major radiology studies. AI: artificial intelligence; AUC: area under the curve; bpMRI: biparametric MRI; DV: development validation; mpMRI: multiparametric MRI; N/A: not available; NPV: negative predictive value.
Target identification was evaluated through submission to the PROSTATEx Grand Challenge, a blinded test with 208 radiologist-identified lesions from 140 patients. The system identified lesions containing csPCa with area under the curve 0.84, using bpMRI data. For prostate gland segmentation, the system achieved 92% average Dice score on held-out test cases from the PROMISE12 dataset (10 patients), in line with the state-of-the-art.
CONCLUSION
The AI system showed promising performance and specificity, suggesting it could help support exclusion of csPCa with high sensitivity and NPV, while assisting the identification of lesions to target for biopsy. Its accuracy appears to exceed published results for similar prostate CAD/AI systems. Limitations of this research include methodological and dataset differences between the reported studies, and small test set sizes. Workflow integration, training, and evaluation with larger, more diverse datasets and prospective studies are recommended, and regulatory approvals are planned. ■