BACKGROUND AND AIMS
The aims of the study were to identify reliable clinical and radiomics features to build machine learning models predicting progression-free survival (PFS) and overall survival (OS), using pre-treatment CT in patients with pathology-confirmed pancreatic adenocarcinoma (PDAC).1
MATERIALS AND METHODS
Pre-treatment portal contrast-enhancement CT-scanner of 253 patients with PDAC were retrospectively analysed between 2010–2019. CT scans were collected from different hospitals in Belgium, with non-uniform scanner models and protocols. Demographic, clinical, and survival data were collected from medical records.
OS was used to stratify patients into a long or short survival groups (OS: ≥10 months; OS: <10 months). For PFS, patients were stratified into a long or short PFS groups (PFS: ≥3 months; PFS: <3 months). Lesions were semi-manually segmented using MIM 6.9.0 software (Cleveland, Ohio, USA), and radiomics features were extracted using RadiomiX research software (supported by Radiomics, Liège, Belgium).
Two-thirds of patients were randomly assigned to training-validation dataset, and the remaining one-third for testing.
A four-step method was applied for feature selection. Firstly, reproducible features were chosen according to recent studies on phantoms and human cohorts.2-4 Secondly, features with good intra-rater reliability were retained based on intra-class correlation coefficient >0.75.5 Thirdly, highly correlated and redundant features were removed using Spearman correlation coefficient >0.95. Finally, the number and names of selected features for the final models were chosen using the wrapper method (WEKA software version 3.8.6 [University of Waikato, Hamilton, New Zealand]),6,7 which finds the best combination of features using a defined classifier (random forest classifier). This procedure was applied in the training-validation dataset with three-fold cross-validation.
Based on random forest, different machine learning models were trained and tested to predict OS and PFS. Model performances were assessed using receiver operating characteristic curves and associated area under the curve (AUC). The AUCs were compared using the DeLong test. Significance was set at p<0.05. This part of the pipeline was computed by using SPSS version 28.0.1.1 (IBM, Armonk, New York, USA) and WEKA software version 3.8.6.
RESULTS
A total of 171 radiomics features were extracted. Out of these, 36 features were retained after assessing inter-scanner reproducibility; 28 features were kept based on intra-rater reliability; and, after evaluation of highly correlated features, 18 features were included. Finally, using the wrapper method, six feature subgroups were selected (clinical and radiomics features, clinical features, and radiomics features [both for OS and PFS]).
Subsequently, six random forest models were trained and tested. The Clinical&Radiomics model was the most predictive for both OS (AUC: 0.75) and PFS (AUC: 0.66). Other models reached lower AUCs (Figure 1 and Table 1).
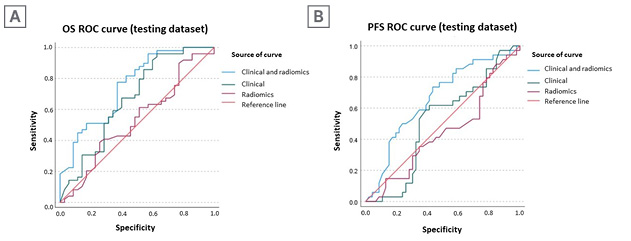
Figure 1: Receiver operating characteristics and area under the curves for overall survival (A) and progression-free survival (B) prediction of the different models.
AUC: area under the curve; CI: confidence interval; OS: overall survival; PFS: progression-free survival; ROC: receiver operating characteristic.

Table 1: Area under the curve for overall survival and progression-free survival prediction of the different models.
AUC: area under the curve; CI: confidence interval; OS: overall survival; PFS: progression-free survival.
It is worth noting that the Clinical&Radiomics model for OS prediction included six clinical features and one radiomic feature (GLCM_homogeneity1);8,9 while the Clinical&Radiomics model for PFS prediction included four clinical features and two radiomic features (GLCM_invDiffMomNor9 and Stats_mean).10
CONCLUSION
Radiomics is an emergent methodology that can be used to predict outcomes. In PDAC, a combination of clinical and radiomics features reached better performances.
Given the significant variability in acquisition protocols and scanners among patients, it is crucial to investigate the reproducibility and repeatability of radiomics features, particularly in the absence of harmonisation techniques.
Future direction of the current project will focus on other outcomes, such as genetic, histological data, and response to treatment.