BACKGROUND AND AIMS
Cardiac MRI provides a wealth of information for diagnosis and serial management of patients with cardiovascular disease, particularly therapeutic drug toxicity, amyloidosis, myocardial fibrosis, and oedema. The accuracy and reproducibility of cardiovascular magnetic resonance cardiac function and strain analyses from cine images are directly related to the observer’s experience, and help to overcome key barriers to patient access for these management tools. Democratising patient access begins with simplifying and improving the imaging data acquisition process. The authors have engaged in the development of a fully-automated deep learning-powered analysis pipeline that starts from digital imaging and communications in medicine, and generates final reports with no or minimal human intervention. The workflow pipeline derives cardiac structure, function, strain from cine images, and myocardial texture analysis from parametric magnetic maps. Central to this overall result was the establishment of cardiac segmentation masks that could be shared with every process from cardiac function to T1/T2 maps (Figure 1). As an example, the manual segmentation of cardiac images performed by skilled human observers was compared to artificial intelligence deep-learning protocols.
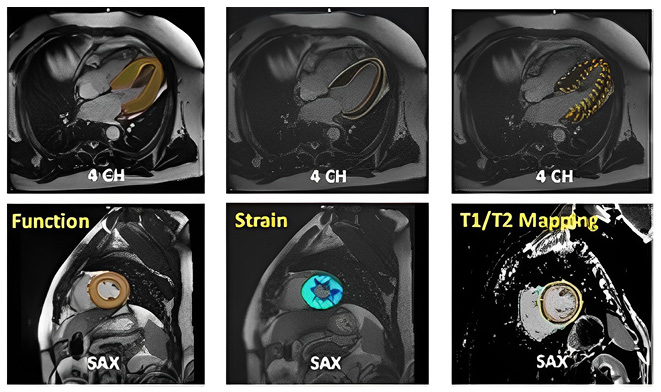
Figure 1: Artificial intelligence feature-tracking segment cardiac borders automatically for structure, function, strain, and myocardial texture analyses.
MATERIALS AND METHODS
Eleven cardio-oncology patients were scanned on a 1.5T scanner (uMR 570 scanner, United Imaging Healthcare Co. Ltd, Shanghai, China) using routine clinical protocols to acquire the multiple sequences and images. The zero-click analysis pipeline is comprised of various neural networks that detects cine images from other sequences; categorises short-axis and long-axis views; detects left ventricular (LV) range from long-axis view, and right ventricular-LV insertion points to setup the American Heart Association (AHA)’s 16 segment model; segments heart chambers and myocardium; calculates cardiac functional parameters; tracks LV myocardium; and calculates myocardium strain parameters. Two experienced medical practitioners performed the same analyses with manual editing.
RESULTS
Agreement between human observers and automated pipeline on cardiac function was excellent for LV end-diastolic volume, LV end-systolic volume, and LV ejection fraction (intra-class correlation coefficient: 0.99, 0.98, 0.98; Pearson’s χ2 test: 0.99, 0.98, 0.98, respectively). The agreement on global circumferential strain, global radial strain, and global longitudinal strain was also excellent (intra-class correlation coefficient: 0.92, 0.85, 0.86; Pearson’s χ2 test: 0.90, 0.85, 0.87, respectively). Within-subject coefficient of variations for human observers was 4.7–16.7% for function analysis, and 14.1–32.2% for strain analysis. Within subject coefficient of variations for automated pipeline was 0.0%.
CONCLUSION
The proposed fully-automated pipeline can flow without technologist intervention to provide highly accurate and reproducible cardiac function and myocardium strain analyses compared to human observations. The proposed workflow shows the promise to be implemented into routine clinical practice to assist medical practitioners.