Abstract
Advanced healthcare delivery prolongs life, but it is also associated with preventable patient harm such as misdiagnosis, suboptimal treatment, and avoidable care-associated injuries. While best practice guidelines are an important tool in reducing unwarranted variation in clinical decision-making, their utility in routine care is limited by the complexity and quality of recommendations, which a clinician is expected to remember or quickly access.
Well-designed, evidence-based clinical decision support (CDS) tools can help clinicians incorporate an often overwhelming reservoir of evidence into their decision-making process. They work best when integrated into standard clinical workflow, so that they do not rely on human memory for their accurate execution. This review focuses on the use of CDS systems in breast and lung cancer, summarising recent findings in the areas of cancer screening, diagnosis, management, and image analysis.
By integrating a vast array of complex data into healthcare workflow, CDS systems allow clinicians to leverage data to provide the best services, timely decision-making, and health-related quality of life for patients with cancer.
However, to realise the full potential of CDS, further well-designed trials are needed to evaluate such tools in real clinical environments, and existing guidelines need to be converted into algorithms that can be interpreted by computers.
ADVANCED HEALTHCARE DELIVERY PROLONGS LIFE, BUT AT WHAT PRICE?
It is clear that the delivery of advanced healthcare prolongs life. The global average life expectancy has almost doubled since 1900, driven by higher standards of living, public health interventions, and improvements in healthcare.1 However, advanced healthcare provision is associated with preventable patient harm, including patient safety incidents, misdiagnosis, delayed diagnosis, and suboptimal treatment. Such events represent a serious problem across all healthcare settings.2 Clinician diagnostic and treatment decisions vary widely. When this variation is associated with deviation from best practice, evidence-based knowledge, unique individual patient needs, or patient preferences, it can appropriately be labelled ‘unwarranted’. Unwarranted variation can be associated with morbidity and mortality (Figure 1).3
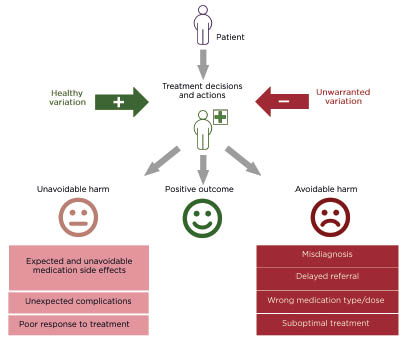
Figure 1: A graphical illustration of variation in treatment decisions and adverse outcomes in healthcare.
In one retrospective review of patient records (N=14,407) in primary care in England, UK, the rate of significant and probably avoidable harm was 35.6 per 100,000 patient-years. Most of these incidents (61%) were due to misdiagnosis, 26% due to medication-related problems, and 11% due to delayed referrals. In most cases (80%), incidents could have been identified sooner, or prevented altogether, if the practitioner had followed evidence-based best practice guidelines.4
Outpatient diagnostic errors occur in approximately 12 million adults in the USA every year. Roughly half of these errors are estimated to be potentially harmful.5 In outpatient settings, delayed cancer diagnosis is considered one of the most harmful types of diagnostic errors.5 One study found that opportunities to diagnose lung cancer were missed in 38% of patients (N=587), resulting in significant diagnostic delays.6
A meta-analysis of global observational studies found that the pooled prevalence for preventable patient harm was 6%, mainly related to medication or other treatments. Twelve percent of events of preventable patient harm were severe or fatal.7 The real incidence rate of patient harm may be even higher, as automated measures of patient safety almost certainly fail to identify all adverse events. An alternative method, the Global Trigger Tool, was developed by the Institute for Healthcare Improvement (IHI). When applied to hospital records from three large tertiary care centres in the USA, results indicated that adverse events occurred in 33% of hospital admissions.8 While this study did not formally judge whether each harm event was avoidable, the study’s authors estimated that at least half of all events detected fell into that category. They also noted that more than 9% of all hospital admissions directly resulted from outpatient care-associated harms (unpublished data).
Although progress has been made in improving the quality of healthcare globally, the World Health Organization (WHO) reports that 10% of patients are still adversely affected during treatment in high-income countries, and a considerable proportion of patients globally do not receive appropriate, evidence-based care.9
Unwarranted variation in patient care, and the resultant patient harm, is a substantial price to pay. However, we do not have to pay that price.10 CDS has the potential to substantially reduce unwarranted variation.
CAN WE JUST FOLLOW THE PUBLISHED BEST PRACTICE GUIDELINES?
Best practice guidelines are an important tool to improve clinical decision-making.11 They have been developed for many cancers and other disease conditions, directing clinicians to the applicable options for a specific patient. However, their utility in routine care is limited by deployment methods, and the quality of the recommendations.
Guidelines are mainly deployed through clinical training; clinicians are expected to remember them. However, cancer is a complex, heterogenous disease that affects patients of all ages and backgrounds, making decision-making difficult.12 In addition, rapid advancements in therapy and our understanding of cancer presents a significant challenge for oncologists. For instance, over 4 million cancer-related papers have been published in peer-reviewed journals,13 with over 178,000 published in 2020 alone.
Unfortunately, this approach to guideline deployment results in roughly half of all patients not receiving recommended care.14-16 Because of the vast array of options for patients with cancer, it is unrealistic to expect clinicians, relying on memory alone, to be able to select the most appropriate treatment for a specific patient at a specific stage of their cancer journey, without significant time outlay. Indeed, literature suggests that when clinicians have only their training, expertise, and experience to rely on, they struggle with cognitive limitations and biases.3
Ideally, all best practice guidelines would be based on high-quality evidence. However, expert consensus often plays a large role. A limited pool of well-designed studies means that recommendations are often dependent on the individual experts involved.17 An evidence review of 421 clinical practice guidelines found them to be of widely variable quality; only 23% could be considered high quality.18
Well-designed, evidence based CDS tools may help clinicians to incorporate the often overwhelming reservoir of evidence into their decision-making process.3 This is especially true if such tools have the potential to learn, modifying guidelines ‘on the fly’ based on data collected during their use.19
WHAT IS CLINICAL DECISION SUPPORT AND HOW CAN IT HELP?
The WHO defines CDS as: “The provision of knowledge and patient-specific information presented at appropriate times to enhance front-line healthcare delivery.”9 CDS encompasses all tools that support healthcare, including clinical guidelines, computerised alerts and reminders, and documentation templates.9 This article will focus on computerised CDS systems for oncology, many of which can be automated by embedding in electronic health records (EHR) or mobile devices.
CDS tools should facilitate the decision-making process, helping clinicians to adhere to guidelines, to quickly diagnose and/or identify the most suitable treatment for a specific disease, and to gather all the relevant information about the patient and their tumour, so that the best treatment decisions are made from the start (Figure 2). The fundamental elements that a CDS tool must consider include tumour characteristics, patient characteristics, prior therapy, molecular profiling results, combination therapy options, and dosage.20 Many algorithms for prediction or classification have been implemented in CDS systems to diagnose different diseases, and most of these systems have a way of ‘learning’ from the data,21 offering the opportunity to refine guidelines based on patterns in data or clinician feedback. In addition, CDS systems have been developed to assist clinicians with the interpretation of imaging data, such as CT scans or MRIs, with the intention of reducing unwarranted variability.22,23
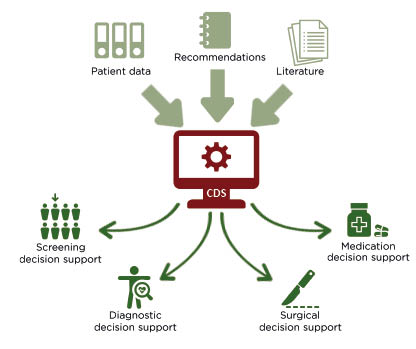
Figure 2: A graphical illustration of how clinical decision support systems are intended to support healthcare practitioners.
CDS; clinical decision support.
CDS systems support clinicians by removing the need to rely on human cognition, as guidelines and protocols are built into standard clinical workflow.3 This implementation strategy is critical to the success of any CDS system.24-26
RECENT RESEARCH INTO THE UTILITY OF CLINICAL DECISION SUPPORT SYSTEMS FOR CANCER
Due to the vast literature available for cancer, the author focused on the use of CDS systems in breast and lung cancer, which have the highest global incidence and highest global mortality, respectively,27 and which represent the huge complexity in treatment decisions for cancer.
Cancer Screening
CDS systems can help primary care practices to identify patients at high risk of cancer, who may benefit from screening tests. For example, in a recent study carried out in the USA, a CDS system implemented algorithms derived from the 2018 National Comprehensive Cancer Network (NCCN) guidelines for genetic evaluation of hereditary cancer. The system was integrated into an EHR and evaluated in a single-centre pilot study (N=143,012). It identified 5,245 (3.7%) patients who met criteria for genetic evaluation. Genetic councillors contacted 71 of those patients, of whom 35% scheduled an appointment, resulting in the identification of pathogenic variants in cancer predisposition genes in two patients.28
Cancer Diagnosis
The first step in the clinical management of cancer is to definitively diagnose the disease. Therefore, supporting clinicians to reduce delayed diagnosis or misdiagnosis, as well as increasing identification of early-stage cancer, has the potential to improve outcomes. A systematic review of studies using CDS systems for cancer diagnosis in primary care between 1998–2018 found that three out of nine studies showed improvements in diagnostic decision-making, three out of nine demonstrated positive effects on secondary outcomes, and one out of nine reported a reduction in time to diagnosis.24
For example, one CDS system that assists radiologists in diagnosing lung cancer from CT scans showed superior nodule-detection sensitivity compared to state-of-the art analysis programs.29 Another CDS tool is currently in use in Birmingham Children’s Hospital, UK, to support non-invasive diagnosis in children presenting with solid body tumours. Early findings suggest that the tool makes the interpretation and analysis of advanced MRI more comprehensive and accessible to clinicians.30
In Baltimore, Maryland, USA, a CDS algorithm categorises lung cancer nodules as malignant or non-malignant using Fleischner Society recommendations, along with smoking history, sex, and nodule location. It can stratify malignancy risk beyond the Fleischner categories and may help clinicians provide more personalised treatment recommendations.31
Finally, a retrospective study in a primary care centre in Saudi Arabia compared clinical outcomes for adults in the 3.5 years before and after implementation of EHR-integrated CDS systems. Findings indicated that CDS systems led to significant increases in breast cancer diagnosis.32
Cancer Management
Cancer is a complex disease with many therapeutic options.33 Therefore, treatment decisions require co-ordinated expertise from diverse health professionals. Multidisciplinary team (MDT) meetings are routinely held to meet this need, but they can be affected by difficulties in transferring expertise across centres, and in evaluating the quality of decision-making.33 CDS systems can support the MDT process by presenting patient data and suggesting the most suitable treatments according to best practice guidelines, potentially using previous cases as evidence.
Clinical decision support systems can improve treatment guideline adherence
For example, an online tool that customises NCCN guidelines for non-small-cell lung cancer (NSCLC) to patients’ clinical and pathologic features was tested at a tertiary care centre in California, USA. Patients seen after the use of the tool (N=76) were more likely to receive smoking cessation counselling and less likely to receive adjuvant therapy than patients seen prior to the use of the tool (N=157), suggesting that the CDS tool increased guideline-concordant care.34
Similarly, a CDS system increased adherence to NCCN breast cancer guidelines by 0.5% (p=0.003) in China (N=1,977 patients). Oncologists changed their initial treatment decision in 5% of patients, citing consideration of the CDS system recommendations, patient factors highlighted by the system, and the decision logic provided as reasons for the change.35 Another CDS tool that combined three breast cancer clinical practice guidelines was tested in Spain and France. In 17% of cases (24 out of 138), clinicians modified their decisions after using the tool, and an external expert determined that in most cases (75%), the new decisions were more appropriate.36
Using an alternative approach, a CDS system was developed in the Netherlands to help multidisciplinary tumour boards construct a shared mental model of individual lung cancer cases. A simulated tumour board using eight primary lung cancer cases found that the system was helpful for accurate diagnosis and classification, helped to cross-validate diagnostic findings, and facilitated cancer staging.37
Clinical decision support tools can predict complications
Accurate assessment of complication risks can support the decision-making process of an MDT. For example, a CDS system incorporating machine learning showed the potential to improve the prediction of significant weight loss following radiotherapy in patients with lung cancer (N=37). In four cases, physicians changed their original prediction after reviewing the CDS system’s output, resulting in an improvement in accuracy (from 0.54 to 0.59).38
In a larger study, the utility of a CDS tool to make relapse risk predictions for breast cancer was assessed in patients for whom adjuvant chemotherapy was uncertain. Use of the tool resulted in changes to initial chemotherapy decisions in 36% of cases (72 out of 200).39
Similarly, an evidence-based neutropenia-risk algorithm developed to assist oncologist decisions regarding cytotoxic chemotherapy was tested on a retrospective cohort of patients with newly diagnosed cancer in the USA (N=126). Mean predicted risk in high-, medium-, and low-risk groups were similar to observed event rates over the following year.40
Clinical decision support systems can support clinical interpretation of genomic mutations
The interpretation of genomic mutations to inform treatment decisions is a formidable task,41 and several CDS systems have been developed to support these decisions. For example, a tool that reports a ‘21-gene recurrence score’ for patients with early-stage breast cancer was assessed across four centres in Spain (N=401). Use of this tool reduced the rate of chemotherapy prescription from 56% to 25%, reducing unnecessary chemotherapy.42 However, since each CDS has a different way of classifying variants and identifying potential treatments, they can produce disparate results. A comparative analysis of three genomic-mutation CDS systems was performed on a cohort of 48 patients in Austria, with breast, colorectal, or lung cancer. The frequency of concordant actionable recommendations was only 4.3–28.0%, depending on the systems being compared, suggesting that further development and testing of CDS algorithms is needed.41
Clinical decision support tools have the potential to predict therapeutic response
Individual patients can respond quite differently to the same treatment, and accurate prediction of this response could support MDT decision-making. A CDS algorithm based on machine learning was developed in South Korea to combine clinical characteristics related to response to anti‑programmed death protein 1 therapy in patients with NSCLC. Compared to separate predictions based on the individual characteristics, the CDS algorithm showed a significantly greater prediction performance.43
Can clinical decision support systems accurately model multidisciplinary team treatment decisions?
Several studies have investigated how well recommendations made by CDS systems align to actual treatment decisions made by MDTs.33,44,45 In Australia, a machine learning model was tested on breast cancer cases (N=1,065). The model more accurately predicted MDT decisions regarding adjuvant cancer therapy than a simple application of guidelines did, suggesting that it has the potential to facilitate the transfer of expert knowledge to more remote centres.33
In a similar study, concordance between CDS-based treatment recommendations and an MDT for patients with breast cancer (N=638) was assessed in a single centre in India. Results suggested a high level of concordance overall (93%), though it was significantly lower in older patients.44
Finally, a study in South Korea investigated the alignment of treatment recommendations from a CDS system versus an MDT in cases of lung cancer (N=405). Results indicated that recommendations were highly concordant (92% agreement) in general. Concordance was lower for patients with Stage II NSCLC and limited disease small-cell lung cancer (83% and 85%, respectively), suggesting that patient preference may be more important in these disease stages.45
Clinical Decision Support for Image Analysis
CT and MRI represent advanced techniques for lesion detection, disease staging, and the assessment of therapeutic response.46 Molecular classification of cancers, and the potential for non-invasive tumour subtyping, are increasingly important. That means that advanced image processing and the integration of imaging and clinical data are increasingly required.22
CDS systems have been developed to assist clinicians in diagnosing cancer using CT or MRI. For example, a deep-learning system designed to support CT-based lung cancer diagnosis outperformed a current state-of-the art analysis program.29 A team at Birmingham Children’s Hospital is evaluating another CDS tool that analyses advanced MRI data to support non-invasive diagnosis in children presenting with solid body tumours. Early findings suggest that the tool facilitates discrimination between benign and malignant tumours with high sensitivity and specificity.30 Additionally, a CDS system has been developed to analyse multiparametric MRI and clinical data to determine the probability that a patient is harbouring clinically significant pancreatic cancer. Initial evaluation suggests that this system has comparable sensitivity and specificity to an experienced radiologist.23
A machine learning approach is also being used to develop a radiomics model for the preoperative prediction of microsatellite instability status from rectal cancer MRIs. This Chinese clinical trial hopes to show that a non-invasive model could be applied in CDS systems to improve diagnostic, predictive, and prognostic accuracy.47
Machine learning techniques are often applied to image processing because of the volume and complexity of the associated data. However, the training of these systems represents a challenge. Training requires vast amounts of quality data to validate performance.22
To address this issue, the International Association for the Study of Lung Cancer (IASLC) is developing the Early Lung Imaging Confederation (ELIC). ELIC will provide access to large numbers of high-quality CT images and associated de-identified clinical information, using a cloud-based infrastructure. The IASLC hopes that this image library will help to improve the reliability of CT-based CDS systems worldwide.46
Utility of Clinical Decision Support to Predict Outcomes in Cancer
Several systematic reviews have been conducted over the past 5 years to assess the impact of CDS systems in cancer. Beauchemin et al.12 reviewed studies of CDS systems used to support therapeutic decision-making in cancer, finding that 56% (five out of nine) demonstrated a significant improvement to process outcomes, and 67% (four out of six) demonstrated significant improvements in patient outcomes.12 A similar review conducted by Klarenbeek et al.48 analysed 61 publications that evaluated the impacts of higher level CDS systems (those using automated clinical guidelines, artificial intelligence, data mining, or statistical methods). In this review, CDS systems were associated with significant improvements for process outcomes and guideline adherence; however, very few of the studies assessed clinical outcomes, and none identified significant improvements with the use of CDS.48
Unfortunately, the quality and utility of CDS systems can be quite variable. Very few are backed up by strong validation data to demonstrate their performance in actual practice. For example, a systematic literature review of systems available for incurable metastatic NSCLC found that few had been validated with recent, external, clinical data, and their calibration and discrimination were often poor.49
LIMITATIONS AND NEXT STEPS
The literature raises several potential facilitators and barriers to the implementation of CDS systems for cancer, summarised in Table 1.
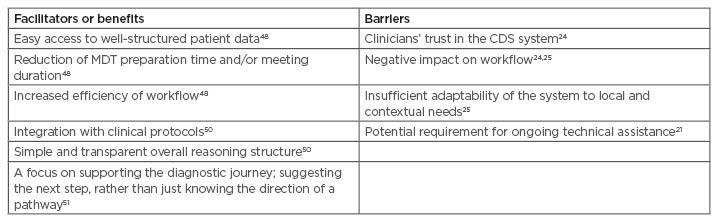
Table 1: Potential facilitators and barriers to the implementation of clinical decision support systems for cancer.
CDS: clinical decision support; MDT: multidisciplinary team.
Implementation strategy is critical to the success of a CDS system.24-26 For example, one CDS system implemented in an outpatient cancer clinic in Norway to support pain management failed to reduce pain intensity, or significantly change opioid prescriptions. This failure was attributed to poor implementation of the system in the clinic.52 Part of the difficulty in successful implementation is the potential for a CDS system to be perceived as a threat to clinical judgment by some clinicians, and fear of implementing new technology.53 Adequate training for the clinicians who will use the CDS system is essential to ensure effective use.
To begin to realise the full potential of CDS in cancer, several steps need to be taken. First, further well-designed trials are needed to evaluate CDS in real clinical environments.24,50,53 There is a paucity of data evaluating clinically relevant outcomes in oncology, and rigorous evaluation is critical to understand the best way to implement CDS systems to improve patient outcomes.54 Second, existing guidelines need to be converted into algorithms that can be interpreted by computers,55 and several attempts have already been made to address this issue.56,57 Finally, the issue of missing data in EHRs needs to be addressed, since many machine learning algorithms require complete datasets.58 One approach to this problem was suggested by Baron et al.,58 who developed a metamodel that produces an aggregate output from cancer modules for which a patient has complete data. The metamodel better predicted survival than many of the individual models. Its performance was similar to that of imputation methods that address missing data, which can be subject to bias.58
CONCLUSIONS
Cancer care delivery could be dramatically better than it is. Unwarranted variation results in a considerable waste of resources, indicating that better care can be delivered more efficiently and at lower cost. Effective precision oncology requires the consideration of a vast array of complex data. By integrating these data into CDS systems, we can leverage rapidly increasing knowledge to provide the best possible healthcare services, timely decision-making, and health-related quality of life for patients with cancer. To realise the full potential of CDS systems, we need to address well-documented limitations in current clinical best practice guidelines and data representation; conduct further well-designed trials to demonstrate the utility of CDS systems in cancer care; and improve our understanding of the best way to implement CDS systems into healthcare systems.