Abstract
Despite enormous enthusiasm, machine learning models are rarely translated into clinical care and there is minimal evidence of clinical or economic impact. New conference venues and academic journals have emerged to promote the proliferating research; however, the translational path remains unclear. This review undertakes the first in-depth study to identify how machine learning models that ingest structured electronic health record data can be applied to clinical decision support tasks and translated into clinical practice. The authors complement their own work with the experience of 21 machine learning products that address problems across clinical domains and across geographic populations. Four phases of translation emerge: design and develop, evaluate and validate, diffuse and scale, and continuing monitoring and maintenance. The review highlights the varying approaches taken across each phase by teams building machine learning products and presents a discussion of challenges and opportunities. The translational path and associated findings are instructive to researchers and developers building machine learning products, policy makers regulating machine learning products, and health system leaders who are considering adopting a machine learning product.
INTRODUCTION
Machine learning is a set of statistical and computational techniques that is becoming increasingly prominent in the lay press and medical research. Outside of healthcare, machine learning was quickly adopted to recommend movies and music, annotate images, and translate language. In healthcare, in which the stakes are high, although the enthusiasm surrounding machine learning is immense,1 the evidence of clinical impact remains scant. New platforms were created to disseminate machine learning research in healthcare, such as the Machine Learning for Healthcare Conference (MLHC), and certain academic journals have been created to provide a platform for the proliferating research. This enthusiasm for novel technologies unfortunately overshadows the challenging path to successfully translate machine learning technologies into routine clinical care.
National and international efforts are underway to ensure that appropriate guardrails are in place for machine learning to become part of routine care delivery. The International Medical Device Regulators Forum (IMDRF) has defined Software as a Medical Device as “software intended to be used for medical purposes that performs its objectives without being part of a hardware medical device.”2 European regulatory agencies and the U.S. Food and Drug Administration (FDA) are embracing Software as a Medical Device frameworks to regulate machine learning technologies, and national strategies for machine learning are emerging.3-6 Regulations are actively being developed and implemented, with new guidance from the FDA in September 20197 and upcoming changes in European Union Medical Device Regulation (EU MDR) in March 2020.8 While regulators and medical professional societies proactively shape the machine learning ecosystem, many challenges remain to achieve the anticipated benefits.
This narrative review proposes a general framework for translating machine learning into healthcare. The framework draws upon the authors’ experience building and integrating machine learning products within a local setting as well as 21 case studies of machine learning models that are being integrated into clinical care. This review focusses on machine learning models that input data from electronic health records (EHR) applied to clinical decision support tasks, rather than models applied to automation tasks.9 Automation tasks are cases in which “a machine operates independently to complete a task,” whereas clinical decision support tasks are cases in which “a machine is concerned with providing information or assistance to the primary agent responsible for task completion.”9 Distinct from prior systematic reviews of EHR models,10,11 the current review focusses on models that have been productised and integrated into clinical care rather than the large body of academic work of published models that are not integrated. The review builds upon related work that highlights how academic and industry partners collaborate to develop machine learning products,12 as well as the need for engagement from front-line clinicians and standard reporting.13
Case studies were selected amongst 1,672 presentations at 9 informatics and machine learning conferences between January 2018 and October 2019. The conferences include American Medical Informatics Association (AMIA) Annual Symposia and Summits, MLHC, Health Information and Management Systems Society (HIMSS) Machine Learning and Artificial Intelligence Forum, and the Health AI Deployment Symposium. Machine learning technologies were included as case studies if they met two criteria: 1) they tackle a clinical problem using solely EHR data; and 2) they are evaluated and validated through direct integration with an EHR to demonstrate clinical, statistical, or economic utility. Machine learning technologies that analysed images were excluded. This review also advances prior work to propose best practices for teams building machine learning models within a healthcare setting14 and for teams conducting quality improvement work following the learning health system framework.15 However, there is not a unifying translational path to inform teams beyond success within a single setting to diffuse and scale across healthcare. This review fills that gap and highlights how teams building machine learning products approach clinical translation and discusses challenges and opportunities for improvement.
MACHINE LEARNING APPLIED TO CLINICAL DECISION SUPPORT TASKS
Machine learning has been described as “the fundamental technology required to meaningfully process data that exceed the capacity of the human brain to comprehend.”16 Machine learning models are often trained on millions of pieces of information. Existing knowledge about individual data elements and relationships between data elements are not explicitly programmed into the model and are instead learned through repeated iterations of mapping between inputs and outputs. This is in contrast to algorithms that comprise predictors and weights that are agreed upon by medical experts. Collaboration between machine learning and clinical experts is crucial and there are a range of modelling techniques that incorporate varying amounts of clinical expertise into model specifications.17 Machine learning models can be trained in a supervised and unsupervised fashion. Supervised models assume that the output labels, for example a disease, are known up front, whereas unsupervised models assume that the output labels are unknown. An example of a supervised model is identifying which patients will develop sepsis, a known entity, whereas an example of an unsupervised model is identifying unknown subgroups of asthma patients. Most models integrated into clinical workflows as clinical decision support are supervised machine learning models.
This review focusses on models applied to clinical decision support tasks rather than models applied to automation tasks. Not only does automation involve heightened regulatory burden,18 but machine learning is initially expected to impact healthcare through augmenting rather than replacing clinical workflows.19 The distinction between decision support and automation is critical: “while it may be assumed that decision support is simply a stepping stone on the progression towards full automation, the truth is that decision support systems have fundamentally different considerations that must be accounted for in design and implementation.”9 Recommendations for the design and implementation of machine learning as clinical decision support are only beginning to emerge.20
Machine learning can be applied to a wide variety of clinical decision support tasks in the inpatient and outpatient setting. Of the 21 case studies, 14 apply primarily to the inpatient setting and 7 apply primarily to the outpatient setting. Some models, such as a 30-day readmission model, can inform clinical decisions in the inpatient and outpatient setting. Examples of inpatient applications include prediction of intensive care unit transfer, acute kidney injury, sepsis, and Clostridium difficile infection, while examples of outpatient applications include prediction of chronic kidney disease progression, death, surgical complications, and colon cancer. Table 1 presents how models from the 21 case studies can be translated into clinical care and provides example clinical workflows. Additional details about each model are provided in the next section. This workflow summary is designed to be illustrative and more comprehensive overviews of models and potential workflows can be found elsewhere.10,21 Configurations are categorised as either centralised or decentralised. In centralised workflows, the user of the clinical decision support is removed from direct in-person patient interactions. The user may be a physician, nurse, or care manager involved in managing the health of a population or cohort of patients. Centralised workflows are often involved in ‘command centres’ or ‘air-traffic controls’. In decentralised workflows, the user of the clinical decision support is directly involved in in-person patient interactions and is typically a nurse or physician. Decentralised clinical decision support tends to be directly embedded within the EHR. However, there is no single best workflow for a model and in fact many implementations of clinical decision support fail to improve outcomes.
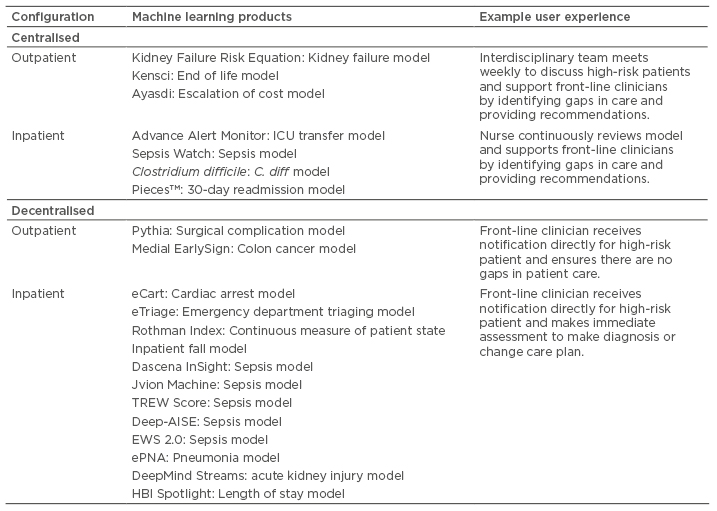
Table 1: Types of workflow configurations for machine learning applied to clinical decision support tasks.
AISE: AISepsis Expert; eCART: electronic Cardiac Arrest Risk Triage; EWS: Early Warning Score; ICU: intensive care unit; TREW: targeted real-time early warning.
THE TRANSLATIONAL PATH
The pathway for translating machine learning applied to clinical decision support tasks is based on an examination of 21 machine learning products and the authors’ own experience integrating machine learning products into clinical care.22-24 In this section, machine learning technologies are referred to as products rather than models, recognising the significant effort required to productise and operationalise models that are often built primarily for academic purposes. Table 2 summarises the products, provides context on the origin of the team and development effort, and highlights translational milestones. To map between individual products and the translational path, milestones for each product are marked within three phases: 1) design and develop; 2) evaluate and validate; 3) diffuse and scale. These phases are described in more detail below.
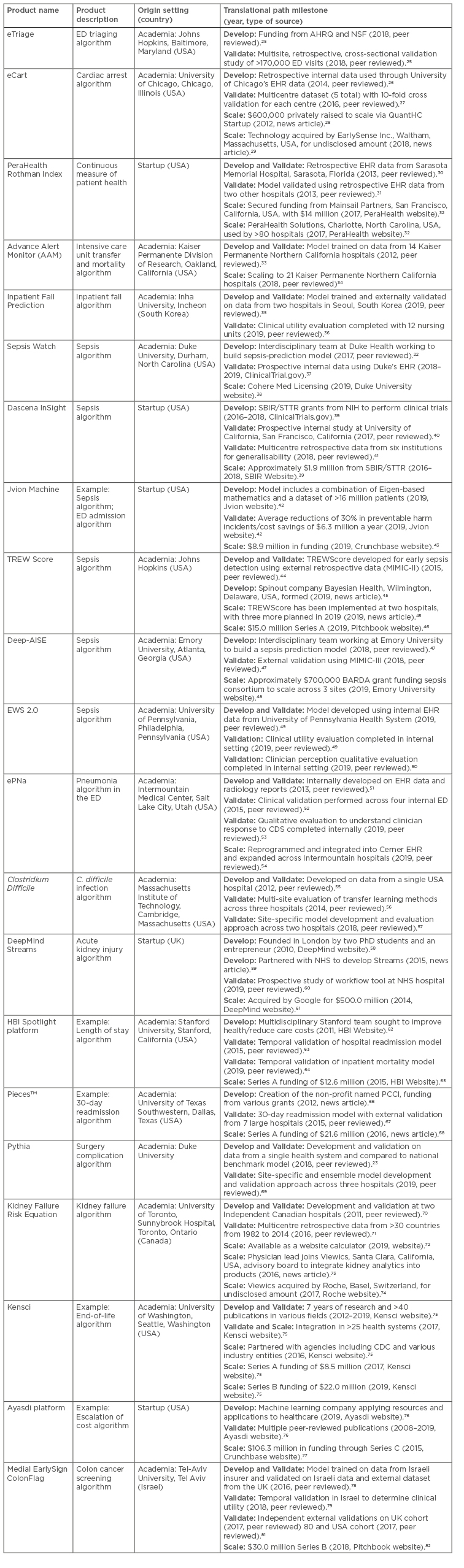
Table 2: A table examining the experience of 14 machine leaning products actively undergoing translation into clinical care. The table includes the name, a brief description of the machine learning product, the origin, and the path to translation.
AHRQ: Agency for Healthcare Research and Quality; BARDA: Biomedical Advanced Research and Development Authority; CDC: U.S. Centers for Disease Control and Prevention; CDS: clinical decision support; ED: emergency department; EHR: electronic health record; MIMIC: Medical Information Mart for Intensive Care; NSF: National Science Foundation; PCCI: Parkland Center for Clinical Innovation; SBIR: Small Business Innovation Research; STTR: Small Business Technology Transfer; TREW: targeted real-time early warning.
Milestone highlights also track the type of source as peer review, online marketing by the product development entity, or industry news. The products are designed to solve a variety of clinical and operational problems ranging from sepsis to escalation of cost and capture experiences from across the globe. The important role of academic research in commercialising products cannot be understated, as 16 of the 21 products were originally developed in academic settings. Many of those products are then externally licensed and are being scaled and diffused via commercial entities. Notably, these products have raised more than $200 million in private venture capital, but several products have also been funded through government grants as well as health systems.
The translational path focusses on products that are actively being integrated into healthcare delivery settings and are often also being diffused and scaled beyond the original development context. The translational path, depicted in Figure 1, contains four phases discussed in detail below: 1) design and develop; 2) evaluate and validate; 3) diffuse and scale; 4) and continuing monitoring and maintenance. Each step contains a set of activities that teams building machine learning products often complete. The activities do not define requirements for any single product, but are representative of the activities completed across the 21 products in Table 2. The path is not linear and may be highly iterative.
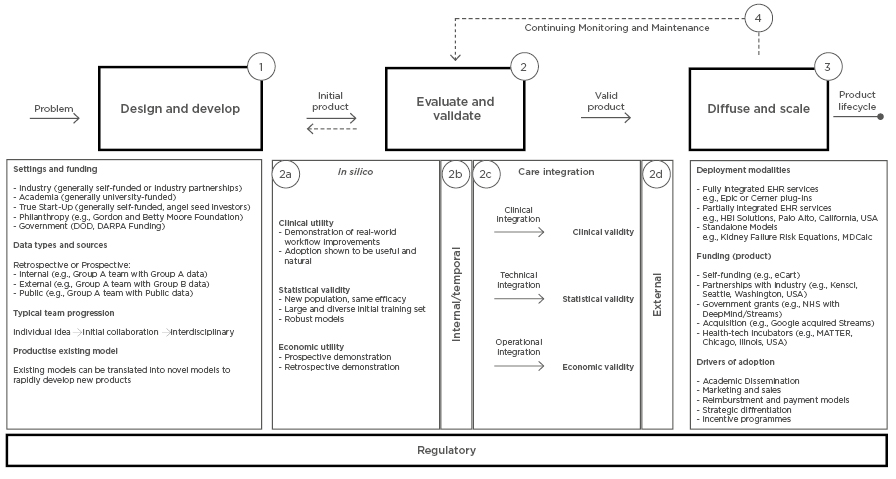
Figure 1: A path for the design, development, evaluation, validation, diffusion, and scaling of machine learning models to be used in clinical care. The translational path begins on the left with the ‘problem’ to be addressed by the product and concludes with the ‘product lifecycle’ on the right. This path is designed to be a comprehensive representation of the current landscape, but is not meant to specify the steps taken by any individual product. Numbers denote the steps of the path.
DARPA: Defense Advanced Research Projects Agency; DOD: Department of Defense; eCART: electronic Cardiac Arrest Risk Triage; EHR: Electronic Health record.
1. Design and Develop
The first step to building a machine learning product is identifying the right problem to solve. Healthcare is a data rich environment and even though a model can be developed to generate an insight, for the insight to support clinical decisions it must be actionable and must have the potential to impact patient care. Many of the products in Table 2 generate insights for conditions that require immediate action in the inpatient setting, such as cardiac arrest, sepsis, and deterioration. Products focussed on the outpatient setting tackle problems associated with high costs to healthcare payers or providers, such as surgical complications, hospital readmissions, and end-stage renal disease.
The setting and funding of the team shapes many aspects of how the machine learning product is designed and developed. For example, in an academic setting it may be easier to cultivate collaborations across domains of expertise early on in the process. However, academic settings may have difficulty recruiting and retaining the technical talent required to productise complex technologies. Funding sources can also vary as products proceed through different stages of the translational path. For example, many of the products in Table 2 were initially funded internally or externally through grants and secured private investment once the product was licensed to an outside company. Setting also significantly impacts the data available to develop a machine learning product. In an academic setting, teams may have access to internal data, whereas teams situated outside of a healthcare system need to obtain data through partnerships. Public datasets also play an important role in promoting product development. Three sepsis products (Deep-AISepsis Expert [AISE], targeted real-time early warning [TREW] Score, Insight) used Medical Information Mart for Intensive Care (MIMIC)-III data for training or evaluation.83
Finally, there are cases in which existing algorithms are productised while more sophisticated machine learning techniques are developed as product enhancements. A notable example of this is DeepMind Streams, which originally productised a national acute kidney injury algorithm.84 In parallel, DeepMind implemented and evaluated the workflow solution as well as developed machine learning methods to enhance the product.60,85 Similarly, the regression-based Kidney Failure Risk Equation (KFRE) used to predict progression of chronic kidney disease, was productised by Viewics inc., Santa Clara, California, USA, while additional technologies were built in parallel.
2. Evaluate and Validate
An initial round of machine learning product evaluation and validation, Step 2a, can be completed entirely on retrospective data. These experiments are called ‘in silico’ and demonstrate three dimensions of validity and utility.21 Clinical utility addresses the question: can the product improve clinical care and patient outcomes? This requires that the team marketing or developing a machine learning product can calculate baseline performance on data relevant to the adopting organisation. Statistical validity addresses the question: can the machine learning product perform well on metrics of accuracy, reliability, and calibration? This requires that there is agreement on important and relevant model performance measures and a sense of what makes a product perform well enough for adoption. Finally, economic utility addresses the question: can there be a net benefit from the investment in the machine learning product? The economic utility can be demonstrated through cost savings, increased reimbursement, increased efficiency, and through brand equity. All forms of utility and validity are ultimately in the eye of the beholder. As such, relationships and communication between the machine learning product team and organisational stakeholders are critical.
The evaluation and validation of machine learning products requires multiple iterations. Demonstrating utility or validity on retrospective, in silico settings does not guarantee that the product will perform well in a different setting57 or in a different time period.86,87 The utility and validity of the product must be reassessed across time (Step 2b) and space (Step 2d). Evaluating a machine learning product on a hold-out and temporal validation set (Step 2a) is recommended before integrating a product into clinical care.14 Evaluating a machine learning product on external geographic datasets (Step 2d) can help drive adoption in new settings. Of the products listed in Table 2, Sepsis Watch, Advanced Alert Monitor, ePNa, and Early Warning Score 2.0 were integrated within the healthcare organisation that developed the product without external validation. On the other hand, electronic Cardiac Arrest Risk Triage (eCART),26,27 KFRE,71 InSight,41 Rothman Index,31 PiecesTM readmission model,67 Deep-AISE,47 and eTriage25 completed peer-reviewed external validations. The two products with the most extensive external validations include the KFRE, which was validated on a multi-national dataset consisting of >30 countries,71 and ColonFlag, which was validated on cohorts in the USA,81 UK,80 and Israel.88 These external validations evaluate the same model in different geographical contexts. However, several teams are taking a different approach to validating models across settings. The teams working on C. difficile and surgical complication models are building generalisable approaches by which site-specific models are developed and validated.57,69
The production environment of a health information technology system often differs dramatically from the environment that stores retrospective or even day-old data. Significant effort and infrastructure investment are required to integrate products into production EHR systems (Step 2c). One study estimated the cost to validate and integrate the KFRE into clinical workflows at a single site at nearly $220,000.24 Redundant costs across sites, as a result of a lack of interoperability and lack of infrastructure, would require similar investment by institutions following a similar approach. Furthermore, the ‘inconvenient truth’ of machine learning in healthcare was pointedly described as “at present the algorithms that feature prominently in research literature are in fact not, for the most part, executable at the front lines of clinical practice.”89 Finally, before being integrated into clinical care, a machine learning product needs to be evaluated and validated in a ‘silent’ mode, “in which predictions are made in real-time and exposed to a group of clinical experts.”14 This period is crucial for finalising workflows and product configurations as well as serving as a temporal validation (Step 2b). An example of a silent mode evaluation is an eCart feasibility study.90
Although represented as a single arrow, the ‘Clinical Integration’ step (Step 2c) can often be the most difficult step in the entire translational path. Most implementations of clinical decision support do not have the intended effect because of the difficulty with clinical integration. What differentiates the products listed in Table 2 from most machine learning models is that these products have undertaken clinical integration. Only one product, InSight (Dascena, Oakland, California, USA),40 conducted a single-site, randomised control trial with 142 patients and demonstrated positive results. This single study needs to be followed up with larger trials from every team trying to drive adoption of a machine learning product.
3. Diffuse and Scale
There are many machine learning products that focus on solving a local problem. The next challenge is to diffuse and scale across settings, which requires special attention to deployment modalities, funding, and drivers of adoption. As described earlier, machine learning products that ingest structured data from EHR require significant integration effort and infrastructure. This has driven the rapid adoption of models and algorithms sold and distributed by EHR vendors.91 To scale, machine learning products must be able to ingest data from different EHR and must also support on-premise and cloud deployments. For this reason, many models are also distributed as stand-alone web applications that require manual entry to calculate risk.
During this stage, machine learning product teams seek external investment and financial resources. As shown in Table 2, tens of millions of dollars are often raised by companies trying to scale products. The resources are required for both scaling deployment of the product as well as navigating the drivers of adoption. Several adoption strategies include academic dissemination, marketing and sales, and partnerships with regulators and payers to create reimbursement mechanisms. The “nonadoption, abandomnent, and challenges to the scale-up, spread, and sustainability of health and care technologies” is an example of a technology adoption framework that covers seven domains and has been recently applied to machine learning products.92,93 To date, no machine learning product ingesting EHR data has successfully diffused and scaled across healthcare. The products listed in Table 2 are as far along as any and will be closely watched over the coming years.
4. Continuing Monitoring and Maintenance
The translational path for a machine learning product does not have a finish line. Data quality, population characteristics, and clinical practice change over time and impact the validity and utility of models. Model reliability and model updating are active fields of research and will be integral to ensure the robustness of machine learning products in clinical care.94,95 Another example of model maintenance is updating outcome definitions to retrain models as scientific understanding of disease progresses. For example, many of the sepsis products listed in Table 2 use sepsis definitions that pre-date Sepsis-3, the most recent international consensus definition.96 Similar to other technology innovations, the product lifecycle continues and will need to adapt to changes in market dynamics and organisational needs. Similarly, the product will evolve over time and will require continued validation and iteration. Throughout the process, teams developing machine learning products need to work closely with regulators and operate within evolving regulatory frameworks.14
CHALLENGES AND OPPORTUNITIES
The translational path described above is not well trodden and, for better or for worse, the products listed in Table 2 are establishing norms for the industry. Across the 21 products, there are opportunities to improve how machine learning products are translated into clinical care. Outside of the scope of this review, the path of IDx-DR, a machine learning product used to automatically diagnose diabetic retinopathy, is instructive. IDx-DR has conducted a randomised control trial and has received regulatory approval in both the USA and EU as a medical device.97,98 IDx-DR is now actively being scaled and diffused. Unfortunately, many products in Table 2 are pursuing ‘stealth science’ to protect trade secrets and avoiding regulatory or academic scrutiny.99 While stealth science is not uncommon amongst biomedical innovations, lack of transparency is particularly concerning with machine learning. This narrative review was unable to provide standard metrics of adoption, because many of the figures marketed by product developers have no peer-reviewed evidence. Machine learning products, which often lack inherent interpretability, need evidence that ensures validity as well as safety and efficacy.
There are three opportunities to enhance how all machine learning products that ingest structured EHR data are translated into clinical care. First, data quality systems and frameworks need to be adopted to ensure that machine learning models have face validity. Significant effort and resources are required to transform the raw data extracted from EHR into a usable format for training machine learning models.24 Distributed research networks that leverage EHR data for clinical research have developed frameworks for assessing quality of EHR data, but these frameworks have not been adopted by machine learning product developers.100-102 Incorporating high quality data into a model is as important as incorporating that same data into a pharmaceutical clinical trial. However, reporting the results of data quality assessments rarely accompanies reporting of model performance. Second, without interoperability across EHR systems, machine learning products will continue to face significant challenges scaling and diffusing across systems. New regulatory and policy mechanisms need to drive interoperability between EHR systems. Third, products listed in Table 2 that predict the same outcome cannot be easily compared. Reporting of machine learning models often fails to follow establish best practices and model performance measures are not standardised across publications.21,103,104 Data sources and data transformations also impact model performance across studies. Furthermore, there is no current standard definition of accuracy and patient health outcomes against which to measure the products. There is a head-to-head comparison of the Advance Alert Monitor and eCart on the same dataset,105 but this practice is exceedingly rare. Benchmark datasets, funding mechanisms, and agreement on model and clinical performance measures must be established to facilitate comparisons across products and settings.
Finally, there are a host of ethical challenges entailed in each step throughout the translation of machine learning in clinical care. First, patients are largely left unaware when personal data is shared with machine learning model developers, whether through a waiver of consent within an academic organisation or through a business agreement with an industry partner. These privacy concerns are prompting legal challenges and revisions of healthcare privacy law across the USA and Europe.106 Second, the dataset used to train a model107 or the outcome that a model predicts108 can have significant implications for how models lessen or worsen disparities in healthcare. Unfortunately, these biases have been discovered in models that run on hundreds of millions of patients. There are additional ethical challenges in machine learning that are described in more detail in related reviews.107,109 Teams building machine learning products need to consider these challenges early and often and incorporate ethical and legal perspectives into their work.
CONCLUSION
Despite enormous enthusiasm surrounding the potential for machine learning to transform healthcare, the successful translation of machine learning products into clinical care is exceedingly rare. Evidence of clinical impact remains scant. This review examines the experience of 21 machine learning products that integrate with EHR to provide clinical decision support. The steps and activities involved in the design and development, evaluation and validation, and scale and diffusion of the machine learning products are described. This translational path can guide current and future efforts to successfully translate machine learning products into healthcare.