Digital twins are the output of AI-based prognostic models and are already being given the go ahead by regulatory bodies. How are they set to modernise the clinical trial landscape, and what would greater use mean for patients and the industry?
Words by Cheyenne Eugene
You arrive at the clinical trial waiting room. Excitement and nerves have set in, to the point you don’t notice the distinct pairs everyone sits in. The receptionist hands over some paperwork and gestures for you to sit down. As you flick through the pages, someone sits next to you. A familiar voice greets you, and when you look up you meet your own eyes – eyes not comprised of cells, but pixels. Meet your digital twin…
Now this may sound more like Michael Bay’s The Island than the current reality, but it could lie closer than you think. Digital twin (DT) technology is emerging and it’s on the brink of modernising clinical trials.
Understanding digital twins
DTs are the output of AI prognostic models that simulate the control outcome for an individual patient. The model essentially predicts how a patient would likely evolve over the course of a clinical trial if they were given a placebo. Clinical trials that use DTs are referred to as ‘twin randomised control trials’, or twin RCTs.
At the start of the trial, baseline data is collected from the patient and inputted into the generative AI model built upon a large compendium of historical data from previous trials and observational studies. Using both sets of data, the model can predict the patient’s prognosis if they were to receive the control. The data generated is statistically indistinguishable from those of actual control subjects.
Digital twins are critical for pharmaceutical success
Speaking at Reuters Events: Pharma Clinical 2022, Charles Fisher, Founder and CEO, Unlearn.AI, elaborates on this technology. If the patient receives the active treatment within the trial and an observed outcome is received, the “difference between the observed treatment outcome and the predicted placebo outcome is an estimate for the individual treatment effect”, he explains. This can then be aggregated over the entire group of patients to get an average estimate of the drug’s treatment effect.
Twin RCTs hold the potential for faster, more efficient clinical trials with fewer subjects, providing actionable insights into which patients achieve the greatest benefit of a treatment – all while ensuring comprehensive, reliable evidence with fewer barriers. As such, Haoda Fu, Associate Vice President, Enterprise Lead Machine Learning and AI, Eli Lilly, asserts: “Digital twins are critical for pharmaceutical success.”
The data issue
While DTs hold huge potential for clinical trials, unfortunately, the “AI-based models don’t work by magic”, says Fisher. Instead, they need to be fuelled by huge swathes of data, and it’s this data that also causes the most issues.
“We can only predict things for which we have data to learn from,” Fisher states. While access to data such as electronic health records, historical trial data, registries and observational studies is increasing, data ownership remains a contentious and shifting landscape for the industry. Autonomy over one’s data, including monetisation, is trending, leaving a lingering uncertainty on how DTs will have to adapt in the future.
But that’s not the only obstacle. “Another limitation with AI-based models is that since they learn from data, they don’t learn perfectly,” highlights Fisher. There are questions over what happens to a clinical trial if the predictions from the AI model are wrong, if there are errors or biases in the model that mean it predicts better for some people than it does for others, and if it systematically over- or undercalculates the rate of disease progression. If AI models are biased, Fisher asks: “How could we trust a treatment effect that’s estimated like this from a single-arm clinical trial? The short answer is: we don’t.”
To counteract potential biases, twin RCTs are run with some patients receiving active treatment and others a placebo. This means data from control patients can be compared against the AI-based predicted prognosis for those on the placebo to debias the model. The result is a mathematical framework called PROCOVA.
Twin RCTs that use PROCOVA “reduce the size of the control group by up to 40% while maintaining the same power as a larger study”, explains Fisher. “Some patients receive a placebo, but fewer than in a typical clinical trial. In fact, you need fewer patients overall
to achieve the same precision.”
With that, DTs offer a solution to the long-term trial recruitment and retention impediment faced by R&D teams, and it’s generally thought that this, and other benefits, greatly outweigh the limitations overall.
Future potential
The use of DTs means rigorous evidence is produced in a fraction of the time required for traditional methods, and this has proved appealing to the European Medicines Agency, which supports the use of DTs in a clinical trial setting.
Importantly, DTs should not be confused with synthetic control arms (SCAs) that add patients who were not in the original patient sample of the trial. In fact, the use of SCAs is being limited by regulatory bodies due to increased capacity for errors. Instead, DTs add information about patients enrolled in the trial, and the FDA is putting DTs front and centre of the next phase of clinical trial design innovation.
People often want to have a faster horse instead of realising that there is something called an engine
However, use of DTs is not bound only to clinical trials, and Fu is already witnessing this type of technology starting to be deployed across the pharma supply chain. Offering an example, he says: “In commercial, we use virtual twins to predict customer engagements and next best action.”
Despite inevitable limitations, DTs offer increased analytical power and confidence in results, reduced trial size and cost, and a higher proportion of participants trialling the active treatment, all while keeping regulatory risk in check. “People have to learn and appreciate the new technology,” Fu says, addressing the sceptics. “People often want to
have a faster horse instead of realising that there is something called an engine.”
Clinical trials are thirsty for innovation, and the value proposition of this technology is extended to both the pharma industry and the patients it exists to serve. It’s only a matter of time before there’s widespread adoption. They’re here and ready to meet you: say hello to your digital twin.
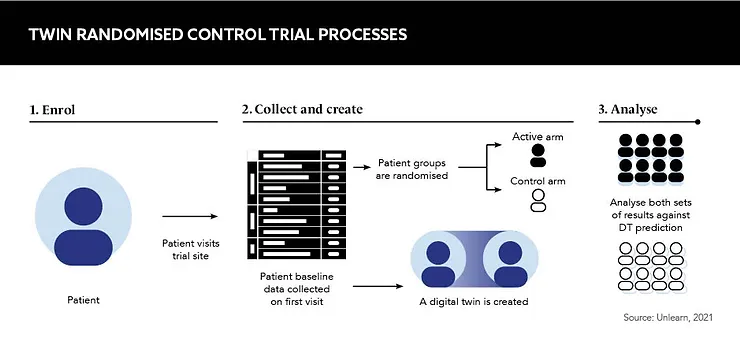