Abstract
Current clinical recommendations provided by the 2016 European Society of Cardiology (ESC) and 2017 American College of Cardiology (ACC)/American Heart Association (AHA)/Heart Failure Society of America (HFSA) are substantially distinguished in the use of circulating biomarkers in the management of heart failure (HF). To date, natriuretic peptides continue being the universal biomarkers used in diagnosis, risk stratification, and prediction of cardiovascular death, all-cause mortality, and HF-related outcomes for patients with both phenotypes of HF. However, biomarkers of fibrosis and inflammation, including soluble suppressor of tumourgenicity 2 and galectin-3, were able to increase predictive ability of natriuretic peptides in HF patients regardless of cardiovascular risk-factor presentation and HF phenotypes. Therefore, there are many various biomarkers describing several pathophysiological processes such as fibrosis, inflammation, oxidative stress, neurohumoral activation, extracellular matrix turnover, and vascular reparation, that play a pivotal role in the natural evolution of HF. This review discusses whether multiple biomarker models are more effective than a single biomarker in improving risk stratification strategies in patients with HF. It emphasises how in routine clinical practice, the multiple biomarker approach to elicit response to therapy of HF and predict clinical outcomes is rare, probably because of the relatively high cost, low affordability, lack of clear recommendations for clinical implementation, and significant disagreements in the interpretation of the data obtained.
INTRODUCTION
The prevalence of heart failure (HF) worldwide is 64.34 million cases, and almost 10 million years have been lost due to HF-related disability.1 The global trend prevalence of HF with reduced ejection fraction (HFrEF) and preserved ejection fraction (HFpEF) over the last decade has shown an increase in HFpEF and tendency to stabilisation of newly diagnosed cases of HFrEF.2 However, the prevalence of HFrEF in developed countries is decreasing, whereas in developing countries it demonstrated steady growth.3 In fact, by the year 2030, in low-to-middle income regions, the prevalence of HF is estimated to rise by over 50%, while high-income countries will have a declined rate of up to 27%.4 Mortality is considerable variable in different regions, i.e., from 34% in Africa to 7% in China, with the overall mortality rate being 16.5%.5 This high variability of HF prevalence and mortality in distinct regions is linked to substantial difference in cardiovascular (CV) risk-factor distribution, affordability of novel technologies in therapy of CV diseases, structure of public health systems, and other factors.5
In addition, the presenting clinical syndromes in HFpEF and HFrEF are not distinguishable from one another, but mortality and co-existing comorbidities substantially differ. In this context, the profile of biomarkers that could be used for diagnosis and prognosis of HFpEF would not resemble their signature in HFrEF. Indeed, predictive ability of natriuretic peptides (NPs) in HFrEF was found as higher than in HFpEF. In contrast, soluble suppressor of tumourgenicity 2 (sST2) and galectin-3 when being added to NPs sufficiently improved final discriminative potency of the model in patients with HFpEF.6
However, there is no agreement in biomarker profile between the American Heart Association/American College of Cardiology (AHA/ACC) and the European Society of Cardiology (ESC).6,7 A contemporary conceptual framework for the diagnosis and management of HFrEF and HFpEF provided by the AHA (2017) includes the first generation of biomarkers such as NPs, sST2, highly sensitive troponin (hs-Tn), and galectin-3.6 Current 2016 ESC HF guidelines indicate the priority of HF having the highest level of evidence before other biomarkers; the discriminative potency of them requires thorough elucidation.7
Reflecting the varying stages of evolving HF, biomarkers can predict its clinical course, short- and long-term prognosis, and risks of all-cause mortality and hospital admission.8 An abundant number of new biomarkers, i.e., mid-regional pro-adrenomedullin, cystatin-C, IL-6, growth differential factor-15 (GDF-15), matrix metalloproteinases, collagen turnover biomarkers, osteonectin, and others, are now clinically available, and continuous monitoring of their levels in peripheral blood is promising in the context of receiving additional prognostic and diagnostic information, which can improve guiding treatment strategies of different phenotypes of HF.8
The aim of this narrative review is to summarise knowledge about prospective potencies of circulating cardiac biomarkers in HF patients that could be useful for routine clinical practice.
METHODS AND METHODOLOGY
The bibliographic database of life science and biomedical information MEDLINE, EMBASE, Medline (PubMed), the Web of Science, and the Cochrane Central were searched for English publications satisfying the keywords of this study. The authors used the following keywords: “heart failure,” “heart failure with reduced ejection fraction,” “heart failure with preserved ejection fraction,” “cardiac cachexia,” “cardiac myopathy,” “cardiovascular risk,” “cardiovascular risk factors,” “cardiac biomarkers,” “circulating biomarkers,” “secretomics,” and “prognosis”. Both authors independently evaluated the quality of the articles, correspondence to the main idea of the study, and constructed the final list of the references.
BIOMARKERS FOR HEART FAILURE
As HF is a multiply complex disease with sophisticated pathogenesis, which is substantially different for HFrEF and HFpEF, there are many biomarkers proposed to determine stages and severity of the disease, predict occurrence, clinical course and outcomes of HFrEF/HFpEF, a risk of CV and HF-related mortality, and a response to treatment (Table 1). Previously, there were suggestions that pro-inflammatory conditions, adipose-tissue dysfunction, oxidative stress and fibrosis predominantly underlie the pathophysiology of HFpEF, whereas neurohumoral activation, cardiac injury, and biomechanical stress much better describe the adverse cardiac remodelling and natural evolution of HFrEF than HFpEF.9,10 This view has been disregarded more recently because biomarkers of biomechanical stress, such as NPs, exhibited their high potency to exclude HFpEF and predict outcomes in both HFrEF and HFpEF, and biomarkers of fibrosis and inflammation, including sST2, galectin-3, increased predictive ability of NPs in HF patients, regardless of CV risk-factor presentation and HF phenotypes.11,12
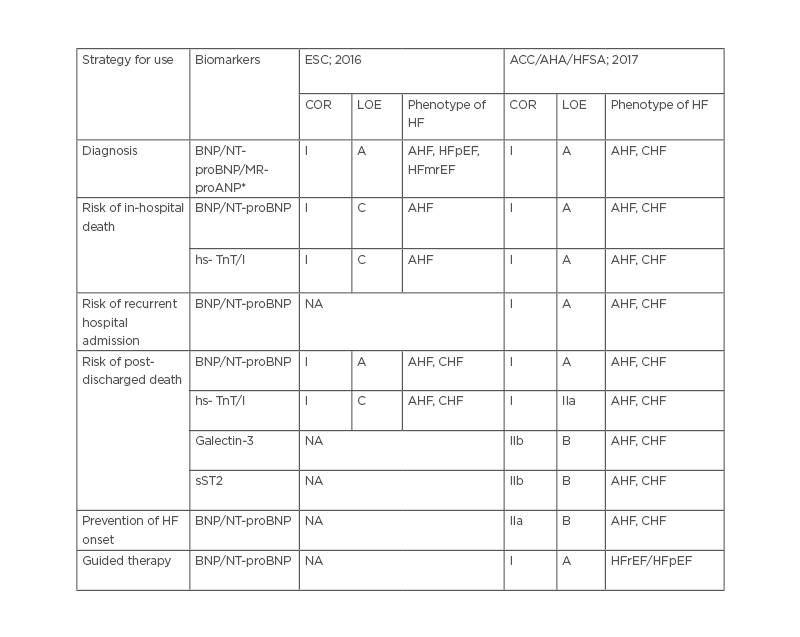
Table 1. 2016 European Society of Cardiology (ESC) and 2017 American College of Cardiology (ACC)/American Heart Association (AHA)/Heart Failure Society of America (HFSA) clinical practice guideline recommendations for the use of biomarkers in the management of heart failure.
*Provided for 2016 ESC recommendation only.
ACC: American College of Cardiology; AHA: American Heart Association; AHF: acute heart failure; BNP: B-type natriuretic peptide; CHF: chronic heart failure; COR: class of recommendation; ESC: European Society of Cardiology; HF: heart failure; HFmrEF: heart failure mid-range ejection fraction; HFpEF: heart failure preserved ejection fraction; HFrEF: heart failure reduced ejection fraction; HFSA: Heart Failure Society of America; hs-TnT : high sensitivity troponin; LOE: level of evidence; MR-proANP: mid-regional pro A-type natriuretic peptide; NA: not applicable; NT-proBNP: N-terminal pro-B-type natriuretic peptide; sST2: soluble suppressor of tumourgenicity 2.
However, NPs remain universal biomarkers that represent diagnosis, risk stratification and prediction of CV death, all-cause mortality and HF-related outcomes for patients with both phenotypes of HF.13,14 There are recommendations for use of the levels ofB-type NP (BNP) >35 pg/mL and N-terminal pro-BNP (NT-proBNP) >125 pg/mL to exclude HF in the acute settings. Higher values of these markers (BNP >100 pg/mL, NT-proBNP >300 pg/mL, and mid-regional pro A-type NP [MR-proANP] >120 pmol/L) are approved for use in diagnosis of chronic HF.6,7 These diagnostic cut-off points are applied strictly, similar to HFrEF and HFpEF, but circulating levels of BNP/NT-proBNP and MR-proANP are frequently lower for patients having HFpEF when compared with those who have HFrEF. A peak value of NT-proBNP >5,000 pg/mL predicts an adverse outcome in HFrEF in-patients, whereas poor prognosis for stable outpatients having HFrEF was suspected when NT-proBNP levels were >1,000 pg/mL. The trend for reducing the levels of NT-proBNP >1,000 pg/mL is now considered a concise indicator of adequate therapy of HFrEF patients, while stability of soaring NT-proBNP levels predicts poor clinical outcomes of the disease.14 Overall, high levels of NT-proBNP provided similar predictive information in patients with HFpEF as in those with HF mid-range EF (HFmrEF) and HFrEF; other biomarkers, including sST2, galectin-3, and high-sensitivity C-reactive protein (hs-CRP), have demonstrated controversial evidence regarding their ability to be carefully tailored to HF phenotypes and comorbidities.15,16 However, negative diagnostic and predictive values of NPs were found to be higher than positive values for prognostication. This implies that single or serial measures of NPs are not enough to completely predict HF evolution, especially among older patients and those who have various CV diseases and comorbidities. These facts are extremely important because there is no descriptive clinical model that can independently predict the clinical endpoint in HFpEF.17
Previous clinical studies have shown that the levels of sST2, galectin-3, and hs-CRP were significantly higher in HFrEF patients when compared with HFpEF individuals.18-21 Although peak levels of sST2, high sensitivity troponin (hs-TnT)/I, galectin-3, and hs-CRP, and their dynamic changes substantially improved predictive potency of NPs among patients with HF, there are serious economic concerns regarding the increased number of biomarkers involved in the multiple diagnostic models.22-27 In addition, there are sustentative disagreements in justifying whether these biomarkers can be adequately discriminative, and have certain calibration, abilities for reclassification, and likelihood analyses in various cohorts of HF individuals, depending on conventional CV risk factors (sex, age, ischaemic versus non-ischaemic aetiology, left-ventricular EF, estimated glomerular filtration rate, hypertension, and dyslipidaemia), comorbidities (diabetes mellitus, abdominal obesity), New York Heart Association (NYHA) functional class, HF medical therapy, and NT-proBNP levels.28 In fact, sST2 and galectin-3 not only predicted all-cause and CV death and HF hospitalisation in both HFrEF and HFpEF, with good performance in Kaplan–Meier analysis in face-to-face comparisons with NT-proBNP and hs-TnT/I, but they yielded significant improvement of comparator models (NT-proBNP and hs-TnT/I) adding prognostic information.29-31 Consequently, sST2 and galectin-3 have been considered as part of a multiple biomarker panel together with NT-proBNP and hs-TnT for most population HF subgroups independently of comorbidity status and CV risk.
CURRENT CLINICAL RECOMMENDATIONS FOR BIOMARKER UTILITY IN HEART FAILURE
Current clinical recommendations provided by ESC (2016) and ACC/AHA/Heart Failure Society of America (HFSA; 2017) are substantially distinguished in the use of circulating biomarkers in the management of HF (Table 1). Both clinical guidelines agreed with the diagnostic strategy of acute and chronic HF based on a measure of circulating levels of NPs additionally to clinical signs and symptoms assay, echocardiographic parameter evaluation and analysis of ECG findings. Therefore, the risk of in-hospital death can also be predicted with NT-proBNP peak level at admission and based on a trend of NT-proBNP level change. However, other utilities of NPs, such as guided therapy and assay of the risk of recurrent hospital admission, were approved in the 2017 ACC/AHA/HFSA clinical guideline, but not in the 2016 ESC HF recommendation. Moreover, sST2 and galectin-3 as alternative biomarkers for additional risk stratification were recommended by the only 2017 ACC/AHA/HFSA clinical guideline, while the evidence for sST2 and galectin-3 remains very weak, as shown by the low grade of evidence, which currently discourages translation to clinical practice.
Thus, the 2017 ACC/AHA/HFSA clinical practice guideline recommends more extensive biomarker strategy for HF management than the 2016 ESC HF recommendation, while there is a gap of evidence regarding use of biomarkers to predict occurrence of different phenotypes of HF, and limited data for risk stratification depending on CV diseases and comorbidities.
BIOMARKER PROFILE AND HEART FAILURE STATUS
There are numerous investigations focusing on the inter-relation between specific biomarkers of fibrosis, inflammation, oxidative stress, neurohumoral activation, extracellular matrix turnover, vascular reparation, and HF status (HFrEF versus HFpEF).32-38 Usually, authors executed univariable and multivariable interactions of baseline biomarker levels and outcomes in HF patients with further correction for the COACH risk engine that included variable anthropometric data, CV diseases (atrial fibrillation, peripheral artery disease, coronary artery disease, dilated cardiomyopathy) and comorbidities (diabetes mellitus, abdominal obesity), estimated glomerular filtration rate, and network analysis. As a result of these investigations, the profile of biomarkers that fitted into HF status for the best was received.37,38 The profile of biomarkers might look like this (Figure 1).
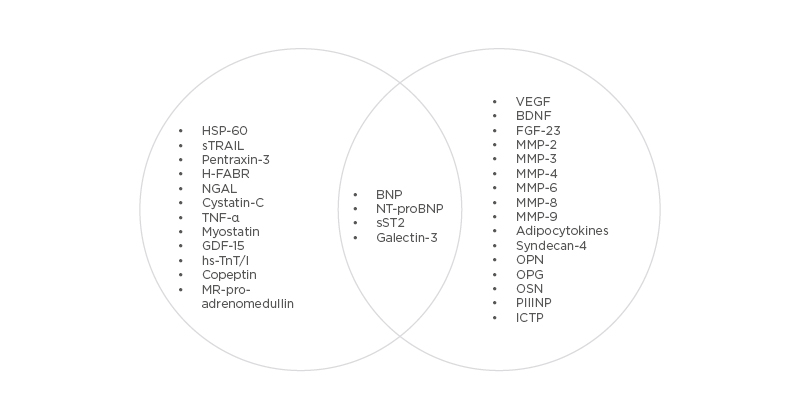
Figure 1: Corresponding biomarker profiles with heart failure status.
BDNF: Brain-derived neurotrophic factor; BNP: B-type natriuretic peptide; FGF-23: fibroblast growth-factor-23; GDF-15: growth differential factor-15; H-FABP: heart-type fatty-acid-binding protein; ICTP: collagen type I carboxy-terminal telopeptide; MMP: matrix metalloproteinase; MR-proANP: mid-regional pro A-type natriuretic peptide; NGAL: neutrophil gelatinase-associated lipocalin; NT-proBNP: N-terminal pro-B-type natriuretic peptide; PIIINP: procollagen type III N-terminal propeptide; sST2: soluble suppressor of tumourgenicity 2; VEGF: vascular endothelial growth factor.
Indeed, various circulating biomarkers in key pathophysiological domains are predictive of outcomes in HFpEF and HFrEF, while their circulating levels were substantially different in patients having distinct HF status. For instance, patients with HFrEF had higher median levels of GDF-15, hs-TnT, heart-type fatty-acid-binding protein, and NT-proBNP, but not sST2, galectin-3, hs-CRP, procollagen peptides, and other abundant biomarkers of extracellular turnover, than those who have HFpEF.21,30,31,39 In contrast, most biomarkers of fibrosis, inflammation and extracellular matrix remodelling have demonstrated higher levels in HFpEF than HFrEF.21,26,30-33,38 A novel paradigm for HFpEF has been described in close connection with CV risk factors and comorbidities, which drive microvascular inflammation, endothelial dysfunction, altered vascular repair, adverse cardiac remodelling, and dysfunction of skeletal muscles and adipose tissue.40 In fact, the presentation and number of risk factors contributing to HFpEF distinguish from those that correspond with HFrEF and demonstrate strong association with age, sex, genetic predisposition, ethnicity, level of education, and region.41,42 In this context, dominant biomarker clusters are required to thoroughly identify individuals at risk of HF occurrence and mortality due to cardiac dysfunction. Consequently, multiple biomarker models appear to be more prognostic than single biomarkers in risk stratification strategies in patients at high risk of HFpEF and those who have overt HFpEF. Obviously, the optimal choice of biomarker combination is strongly needed for effective multiple biomarker strategy to improve HF patient management and outcomes. However, there are limited data to conclude whether multiple biomarker models are better in HFpEF when compared with HFrEF to predict HF-related outcomes and death.38,39
MULTIPLE BIOMARKER MODELS
The panel of biomarkers, which measures diverse biological processes and can be a prognostic tool in HFrEF, was investigated by Ky B et al.43 In a multicentre cohort of 1,513 patients with HFrEF, the levels of several biomarkers such as hs-CRP, myeloperoxidase, BNP, soluble FMS-like tyrosine-kinase receptor-1, Tn-1, soluble toll-like receptor-2, creatinine, and uric acid were measured. Authors created multiple biomarker scores and assessed their performance for prediction of the risk of death, cardiac transplantation, or as a ventricular-assistant device in comparison with conventional clinical risk scores (the Seattle Heart Failure Model [SHFM]) for 2.5 years. Investigators found that patients with HFrEF have the highest tertile of the multiple biomarker score had a 13.7-fold increased risk of adverse clinical outcomes when compared with those who had the lowest tertile (95% confidence interval [CI]: 8.75–21.50). Moreover, these effects were independent of the SHFM and adding the multiple biomarker score to the SHFM markedly improved its discriminative potency.
To characterise HF status, and evaluate a possible relationship between HF status and the risk of all-cause death or HF-related hospital admissions, Chirinos et al.44 used 49 plasma biomarkers received from patients withn HFpEF (n=379) enrolled in the TOPCAT trial. The authors constructed several clusters, which included biomarkers of fibrosis/tissue remodelling (sST2), inflammation (TNF-α, soluble TNF-receptor 1, and IL-6), renal injury/dysfunction (cystatin-C), liver fibrosis (YKL-40), neurohormonal regulators of mineral metabolism/calcification (FGF-23 and osteoprotegerin), intermediary metabolism/adipose-tissue dysfunction (fatty-acid-binding protein-4 and GDF-15), angiogenesis (angiopoietin-2), biomarkers of myocardial injury (hs-TnT), extracellular matrix remodelling (MMP-7), and biomechanical stress (NT-proBNP). Using a machine-learning-derived model, the authors found that a combination of biomarkers was strongly predictive of the risk of HF-related hospital admission and sufficiently improved the risk prediction when added to the Meta-Analysis Global Group in Chronic Heart Failure (MAGGIC) risk score.44 In addition, the model markedly predicted the risk of admission due to HF progression (hazard ratio: 2.74; 95% CI: 1.93–3.90; p<0.0001), which was also independent of the MAGGIC risk score.44
Using a random algorithm Yuan et al.46 found that the combination of creatine kinase-MB, BNP, galectin-3, and sST2 were useful for prediction of HFpEF/HFrEF occurrence.45 In contrast, Zhang et al.46 reported that discriminative ability of sST2 and NT-proBNP for 1-year all-cause death in patients with acute HF was similar and remained significant between patients having ischaemic and non-ischaemic aetiology of HF.
However, galectin-3 did not increase prognostic ability of sST2 and NT-proBNP when added to this combination in patients with ischaemic HFrEF, but not in individuals with non-ischaemic HF regardless of its phenotype. In a cohort of adult patients with HF due to congenital heart disease, a multiple biomarker model constructed from neurohormones (angiotensin II, endothelin-1, norepinephrine, aldosterone, and plasma renin activity), inflammatory biomarkers (hs-CRP, hs-TNF, soluble TNF receptor Types I and II, and IL-6), and BNP, predicted HF mortality.47 Thus, aetiology of acute HF should be considered when an optimal panel of biomarkers has been validated.
Jackson et al.48 measured the levels of biomarkers received from 628 inpatients with acutely decompensated HF. The authors noticed that patients did not always have markedly increased circulating levels of mid-regional pro-adrenomedullin, MR-proANP, copeptin, hs-cTnT, sST2, galectin-3, cystatin-C, combined free light chains, and hs-CRP. Consequently, authors undertook a dichotomisation into low (up to two elevated biomarkers) or high (at least three and more elevated biomarkers) risk groups. It was found that patients with HF from the high-risk group provided much more incremental prognostic value than individuals from the low-risk group (hazard ratio: 2.20; 95% CI: 1.37–3.54; p=0.001). Finally, elevated circulating levels of five biomarkers demonstrated the highest predictive ability for the risk of death.48
Interestingly, in the patient population with HF (n=1,497) enrolled in the CORONA study, a multiple biomarker approach using two panels of biomarkers, which included model 1 (endostatin, IL-8, sST2, TnT, galectin-3, and C-C motif ligand 21) and model 2 (TnT, sST2, galectin-3, pentraxin-3, and soluble TNF-receptor-2), in addition to hs-CRP and NT-proBNP, demonstrated limited attributive potency of inflammatory biomarker panels for identifying the risk of adverse clinical outcomes.49 Therefore, in the PLATO study, elevated baseline levels of NT-proBNP and GDF-15 were strong predictors for all-cause death based on their associations with HF-related death, as well as arrhythmia and sudden cardiac death among patients with acute coronary syndrome.50 Unfortunately, in routine clinical practice, a multiple biomarker approach to elicit response to HF therapy and predict clinical outcomes is very rare, probably due to the relatively high cost, low affordability, lack of clear recommendations for clinical implementation, and significant disagreements in the interpretation of data obtained.
GUIDED THERAPY OF HEART FAILURE
While the current data on using biomarkers to guide HF management remain mixed, more research is necessary to better understand how to utilise biomarkers to improve HF management.51 Guided therapy in clinical practice is mostly based on serial changes of NPs, while other biomarkers such as sST2 and galectin-3 have been considered as a component of this strategy.52 Previous proof-of-concept studies have reported controversial results for biomarker-guided strategies in HF.53 The GUIDE-IT study did not find benefit from biomarker-guided therapy versus usual care in improving the primary endpoints of HF hospital admission or CV mortality in patients with overt HF.54 However, HFrEF patients whose NT-proBNP levels decreased to ≤1,000 pg/mL over 90 days of HF therapy had better outcomes and significantly better quality of life than those who had no reduced levels of the biomarker.55 These findings urge us to reassume whether guide therapy is a powerful tool for the entire population of HF patients. However, the role of a multiple-biomarker strategy in guided therapy is not certain, and well-designed, largescale, multi-centre, randomised clinical trials are definitively required to shed light on these approaches to HF management.
COST/BENEFIT TO USE OF BIOMARKERS
Implementation of biomarker strategies in routine clinical practice corresponds to substantial cost for patients and the health system. Consequently, biomarker-guided decisions should desirably yield economic benefit in HF administration and a better allocation of financial resources. Indeed, the REACH-HF trial has shown that home-based facilitated intervention (with inclusion of predominantly NP biomarkers) for HFrEF was clinically superior in disease-specific 1-year quality of life and, thereby, offers an affordable alternative to traditional centre-based programmes for HFrEF.56 In addition, the risk stratification of elderly patients with HF, based on multiparametric approach, ensured cost benefit in quality of life.57 However, the 2016 ESC and 2017 AHA/ACC guidelines did not concisely emphasise which patients with HF might especially benefit from the biomarker approach. It is reasonable to consider the administration of NPs in patients with HFrEF/HFpEF , while other biomarkers (cardiac Tns, sST2, galectin-3) could have a significant economic impact in predicting all-cause mortality, identifying new patients with HF requiring hospitalisation, optimising treatment, and consequently preserving hospital budget.39,58-60 In fact, uncertain cost/benefit ratio is still one of the unsolved problems for a non-NP biomarker strategy for an unselected real-world population. This is the reason most biomarker models currently cannot be applied in routine clinical practice.
PROSPECTIVES
Several underlying pathophysiological processes (extracellular matrix structural constituents, proteinaceous extracellular matrix) and signalling pathways (regulation of apoptotic process and integrin signalling pathway) involved in the pathogenesis of HFrEF/HFpEF can be described by the signature of non-coding RNAs. Indeed, transcriptome analysis offers great potential in identifying HF biomarkers. Among 1,139 differentially expressed messenger RNAs, He et al.61 identified clusters constructed from nine long non-coding RNAs, three micro-RNAs, and 25 messenger RNAs that were closely associated with progression and outcomes of HF. However, the predictive value of the RNA signature requires elucidation in large clinical trials.62 However, metabolomic and lipidomic phenotyping of patients having HFrEF/HFpEF to indicate a profile of oxidative stress, lactic acidosis, and metabolic syndrome, coupled with mitochondria dysfunction, is a promising approach to stratify them at high risk of poor outcomes.63
CONCLUSION
In conclusion, cardiac biomarkers such as NPs are a promising tool for individualising care in patients with HF, whereas alternative biomarkers (sST2, hs-TnT/I, galectin-3) having weaker clinical evidence than NPs and require more investigation to easily identify the target population in which they would have most cost benefit. A multiple biomarker approach is probably more optimistic for HF-risk stratification and predicting HF-related outcomes than a single biomarker approach, especially in patients with older age, HFpEF, and those having comorbidities. Optimal choice of biomarkers for panels requires in-depth evaluation of economic burden, and not only their discriminative probability for all-cause and CV mortality, HF occurrence, and HF incidence. New biomarkers of the inflammatory axis, matrix remodelling, fibrosis, metabolic axis, and oxidative stress demonstrate uncertainty in their potential therapeutic interventions and are under follow-up investigation. Large clinical trials are required to better understand the role of a multiple-biomarker strategy in HF care to decrease morbidity and mortality, improve quality of life, and propose an easy-to-execute approach for routine clinical practice.