INTRODUCTION
Cardiovascular disorders (CVD) and clonal haematopoiesis of indeterminate potential, the very early state with developmental potential of haematological malignancies, exhibit reciprocal interactions.1,2 While the presence of clonal haematopoiesis of indeterminate potential confers a risk for heart failure, it is unclear if CVDs are associated with elevated risk in development of haematological malignancies.
MATERIALS AND METHODS
In the authors’ preliminary study, to determine the association between CVDs and the risk for haematological cancer, they evaluated 27,231 adults. These individuals, who participated in a preventive healthcare screening programme between 2000–2018, were free of CVD and cancer at baseline. Each participant underwent a complete physical evaluation, blood test, and exercise stress test at baseline. CVD was defined as ischaemic heart disease, stroke, atrial fibrillation, or hypertension. Machine learning and traditional survival analyses were applied to evaluate the association between CVD and haematological cancer. For machine learning, the team used the random forest method using Curat package in r, and incorporated all continuous variables gathered into the model (70% training, 30% test). For survival analysis, they first matched each patient with CVD to a control patient by propensity scores to balance for age, sex, smoking, diabetes, renal function, BMI, and length of follow-up. Then, patients with and without CVD were compared using Cox proportional hazard regression.
RESULTS
Using machine learning, they found that the strongest features associated with haematological cancer in the cohort were low estimated glomerular filtration rate, age, high atherosclerosis cardiovascular disease score, and BMI. Of note, systolic and diastolic blood pressure were also strongly associated with haematological cancer. Next, the team successfully matched 5,500 patients with CVD to controls with a median follow-up of 9.2 years (interquartile range: 5–15 years). During follow-up, 1,380 individuals developed cancer. Of them, 186 individuals developed haematological cancer.
They used Cox proportional hazard regression and the Kaplan Mayer method to determine the risk and hazard ratio of developing haematological cancer. Compared with subjects without CVD, patients with CVD were 91% more likely to develop cancer during follow-up, and 62% more likely to develop haematological cancer (hazard ratio: 1.61; 95% CI: 1.21–2.16). Moreover, the 10-year risk of developing haematological cancer was 1.9% for patients with CVD and 1.0% for individuals without CVD. Notably, the increased incidence of haematological cancers included 38% increase in cases of leukaemia but only 11% increase in cases of lymphoma.
CONCLUSION
Herein, by applying machine learning and traditional analysis, the authors show that CVD risk and CVDs are associated with increased incidence of haematological cancer. Their previous study demonstrated, in murine models, that CVDs promote existing solid tumour growth via release of blood-carried factors.3 It was shown in murine models that CVDs modulate the bone marrow microenvironment, affecting haematopoietic developmental lineage trajectories.4,5 Thus, they hypothesise a model in which CVDs modulate the human bone marrow microenvironment via secretion of blood-carrying factors. Transformed marrow microenvironment may display plasticity properties that can potentially augment accelerated development of haematological malignancy from a pre-tumorigenic state (Figure 1).
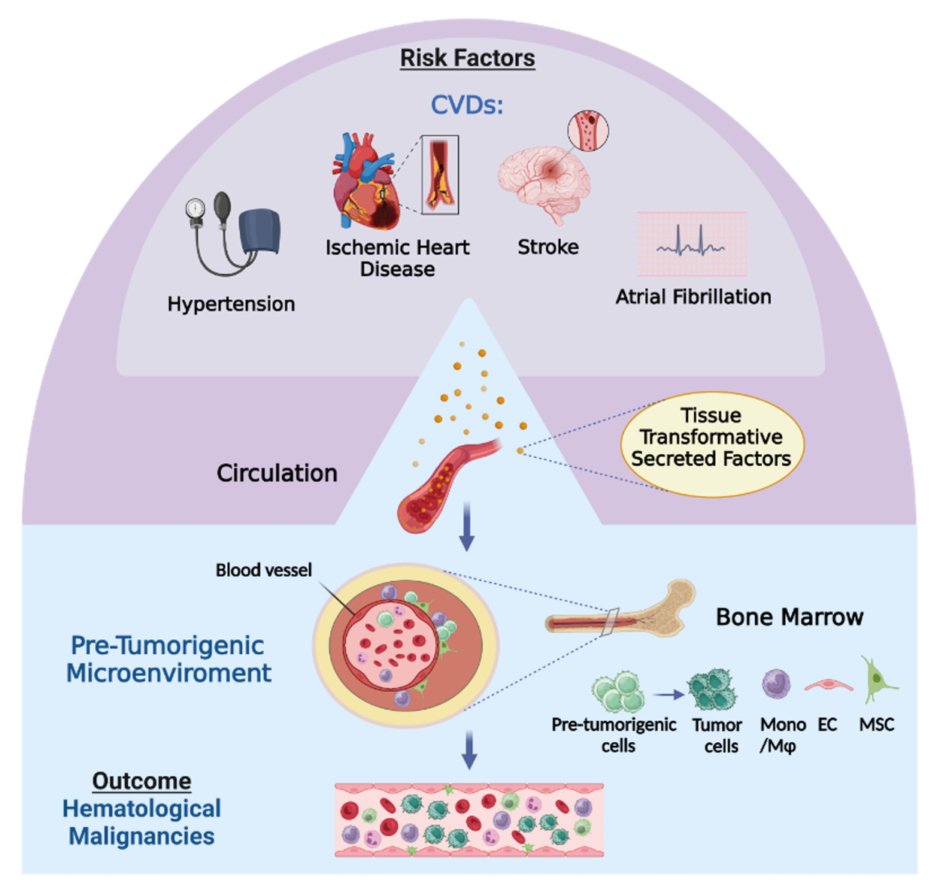
Figure 1: Illustration of hypothetical model for the flow of events by which cardiovascular diseases augment development of haematological malignancies.
The model is based on recent findings and the authors’ results, demonstrating that CVDs are a risk factor for development of haematological malignancies. In the course of CVD pathogenesis, as part of the heart repair efforts cardiac cells alter their secretome and release factors, that are potentially tissue-transformative, into the blood. Upon arrival to the bone marrow, these factors modify cells in the microenvironment in a way that is potentially supportive for development of haematological malignancies from haematopoietic cells in a pre-tumorigenic state. This figure was generated using Biorender.com. CVD: cardiovascular disease.
Currently, basic research studies are conducted to confirm this hypothesis. Deciphering the action mechanisms between CVDs and haematological malignancies could lead to therapeutic advances in cardiovascular and cancer treatment and may ultimately improve patient outcomes.