BACKGROUND
This study sought to validate the use of artificial intelligence (AI) for analysis of an echocardiogram, in a clinical scenario.
A three-stage, deep learning pipeline developed in a previous body of work1 ran on data from a different institution was utilised. Echocardiography studies were exported in Digital Imaging and Communications in Medicine (DICOM) format and stored in a folder with a separate DICOM file for each cine image. The first stage of the pipeline was to input a folder of DICOM files and classify 10 frames from each cine into one of 23 different classes representing different echocardiography views. The classification was performed using a VGG-16 convolutional neural network. The ten classifications were averaged to arrive at an end view classification for the cine. Five views of interest were passed to the second stage for further analysis: the apical two, three, and four-chamber view, and the parasternal short and long-axis. Every frame within the cine images of interest was semantically segmented using separately trained U-Net networks. In the third stage of the pipeline, the segmented views were further analysed to calculate left ventricular end systole volume (LVESV), left ventricular end diastole volume (LVEDV), left ventricular ejection fraction (LVEF), left ventricular mass index (LVMI), and left atrial volume index (LAVOLI).
METHODS
Participants were retrospectively enrolled (N=60) from a previous heart failure (HF) study in which 5-minute protocol echocardiography, 5-minute advanced ECG, metabolomic testing, and next-generation sequencing data were collected. Of these participants, 41 were HF patients and 19 acted as controls. Mean LVEF was 39±10% for HF participants and 57±5% for controls. All participants’ echocardiograms were exported in DICOM format and analysed using the deep learning pipeline. A cardiology registrar independently measured the same five metrics as the deep learning pipeline.
RESULTS
Compute time per study was between 4 and 7 minutes using a single graphics processing unit. Eleven (18%) non-physiological LVESV measurements (and the corresponding LVEF measurements) were excluded. AI-generated measurements had strong, significant correlations with manual measures of LVESV (r=0.8), LVEDV (r=0.77), LVEF (r=0.71), LAVOLI (r=0.71), and LVMI (r=0.6) (p<0.005) (Figure 1A). Receiver operating characteristic (ROC) curve analysis showed a similar discrimination for HF between AI and manual LVEF (HF defined as LVEF <35%), and other HF biomarkers (AUC for AI: 0.88, AUC for manual: 0.93, 95% confidence interval: 0.03–0.15, p=0.19) (Figure 1B).
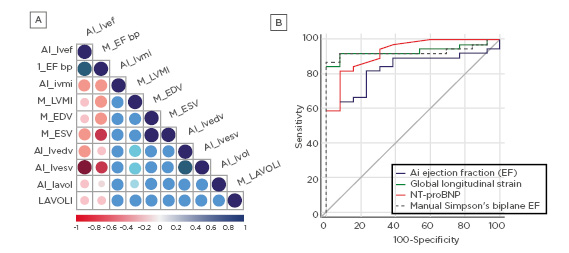
Figure 1: A) Correlation matrix comparing AI and Manual measurements. B) ROC Curve comparing diagnostic tools for HF. LVEF-based diagnostics define HF as <35% LVEF. AI: artificial intelligence; bp: blood pressure; HF: heart failure; LAVOLI: left atrial volume index;LVEF: left ventricle ejection fraction; LVEDF: left ventricular and diastole volume; LVESF: left ventricular and systole volume; LVMI: left ventricular mass index; ROC: receiver operating characteristic.
DISCUSSION
The results demonstrate that AI methods of echocardiography analysis are approaching the accuracy required for clinical utility.