Abstract
Chlamydia trachomatis, a major cause of sexually transmitted infection, poses a range of symptoms including genital discharge, pain during urination, and abdominal pains in women, and can lead to serious health complications such as pelvic inflammatory diseases, infertility, and ectopic pregnancy if left untreated. The need for rapid and accurate detection is imperative so prompt treatment and control of the disease can be achieved. This study conducted an immunoinformatic analysis of proteins of Chlamydia trachomatis (incA, hctA, ompA, omcB, rpoB, and HSP60) for the development of a lateral flow assay-based diagnostic test. Detailed in silico evaluation of selected proteins from publicly available genomic databases was conducted to evaluate their suitability as targets for lateral flow assay-based detection. The series of tests included antigenicity, toxicity, solubility, physicochemical characteristics and molecular docking of the derived constructs, and protein sequence. Chimeric construct was derived from the prediction of linear B cell epitopes, helper T cell major histocompatibility complex II binding epitopes, and IL4 and IL10 inducers using bioinformatic tools at standard thresholds. With a Ramachandra’s score of 95.4% and Z-score of -5.1, results indicate that the construct efficacy is high in potential to provide extreme specificity and sensitivity for the detection of Chlamydia trachomatis in clinical samples as compared to traditional culture-based methods using nucleic acid amplification, hereby providing a quicker and more accurate diagnostic tool for Chlamydia trachomatis infection. Findings offer valuable data for the development of a rapid and reliable diagnostic point-of-care test kit for Chlamydia trachomatis that allows for drastic reduction in clinical wait time and treatment.
Key Points
1. Chlamydiosis, caused by Chlamydia trachomatis, is a highly prevalent sexually transmitted infection with severe reproductive health implications, especially for asymptomatic women who often remain undiagnosed. The urgent need for rapid and accurate diagnostic methods is emphasised by the high incidence rates in regions like Sub-Saharan Africa.2. This study presents an innovative bioinformatics-driven approach aimed at enhancing the diagnosis of Chlamydia trachomatis infections. Through the analysis of target proteins, the research explores the development of a lateral flow assay kit designed for more efficient and accessible detection.
3. Bioinformatics plays a crucial role in bridging the gap between pathogen genomics and practical clinical diagnostics, offering a pathway to more precise and effective healthcare. The designed chimeric construct demonstrated promising antigenic properties, stability, and non-allergenicity, making it a strong candidate for the development of an affordable and rapid diagnostic tool, potentially transforming the landscape of chlamydia screening and control.
INTRODUCTION
Chlamydiosis is one of the most prevalent sexually transmitted illnesses globally caused by the bacteria Chlamydia trachomatis.1,2 Nineteen serovars of Chlamydia trachomatis, categorised based on ompA genotyping, are linked to the bacterium.3 The World Health Organization (WHO) projected about 129 million new cases of Chlamydia trachomatis cases in 2020,1,4,5 with Sub-Saharan Africa having the highest incidence with over 10 million new cases reported each year.5 Chlamydia trachomatis is common among reproductive aged women, with a pooled prevalence of infection of 7.8% in Sub-Saharan Africa.6 Sexually transmitted infections with chlamydial lead to serious health issues, financial difficulties, and social concerns thus, the amount of morbidity linked to a sexually transmitted chlamydial infection is huge worldwide.3 A significant concern is that most women infected with Chlamydia trachomatis are asymptomatic and do not seek medical attention.3 Untreated cases can lead to major consequences for women’s reproductive health, such as pelvic inflammatory disease, tubal factor infertility, and ectopic pregnancy, and a higher chance of contracting other sexually transmitted illnesses.7 Thus, there is a need for rapid and accurate detection to aid prompt treatment and control of the disease. Early screening and diagnosis remain the most important preventive measures.1 The most sensitive assays, nucleic acid amplification tests, are thought to be the best technique for CT detection because of their cell culture-like specificity.8,9 Despite the overall accuracy, these systems have several shortcomings, such as limited turnaround times, high costs, labour-intensive tasks, and the need for sophisticated equipment and highly skilled personnel. Thus, this study aimed to explore more bioinformatics-driven approaches for the enhanced diagnosis of Chlamydia trachomatis infections through analysis of target proteins.
METHODOLOGY
Protein Sequence Retrieval
Seven Chlamydia trachomatis protein sequences (incA, OmpA, hct A, ropB, OmcB, HSP60, HSP61) were downloaded in FASTA file formats from the National Center for Biotechnology Information (NCBI) Protein database (2004), and their accession numbers were recorded.
Antigenicity Prediction
The protein sequences were analysed using VaxiJen v2.0 (The Jenner Institute, Oxford, UK) web server to determine the antigenic and non-antigenic sequences, with the default threshold setting of 0.4 and bacteria selected as the target organism.10 Antigenicity scores of above 0.4 indicate that the peptide is a potential vaccine candidate.11
The antigenicity prediction was performed to ensure the sensitivity of the lateral flow kit being designed.
Prediction of Membrane Topology
The selected antigenic protein sequences were analysed using TMHMM-2.0 (DTU Health Tech, Lyngby, Denmark) for membrane topology prediction.12 Only the outer cell membrane proteins were selected for further analysis.
Linear B Cell Epitope Prediction
B cell epitopes were predicted using the SVMTriP tool (University of Nebraska, Lincoln, Nebraska),13 Immune Epitope Database (IEDB, Maryland, USA),14 and Bepipred Linear Epitope Prediction 2.0 (DTU Health Tech).15 These web servers use algorithms such as Random Forest Regression algorithm15 and Support Vector Machine (SVM)13 to predict antigenic B cell epitopes. Probable epitopes should have scores above the threshold of 0.5 and lengths between 9 and 20 amino acids in order to be chosen for further tests.
Prediction Test for Helper T Cell Major Histocompatibility Complex II Binding Epitopes
The binding of the helper T cell epitopes to major histocompatibility complex (MHC) II alleles were predicted with the aid of the MHC II binding prediction tool on the IEDB Analysis Resource server (Maryland, USA).14 IEDB recommended 2.22 was the selected prediction method. The seven-allele human leukocyte antigen (HLA) reference and peptide length of 15 were chosen. The MHC II reference alleles comprise HLA-DRB1*03:01, HLA-DRB1*07:01, HLA-DRB1*15:01, HLA-DRB3*01:01, HLA-DRB3*02:02, HLA-DRB4*01:01, and HLA-DRB5*01:01. The adjusted rank values were used to sort the peptides and those with a score ≤2.0 were selected as good binders.
Prediction of IL4 and IL10 Inducers
The final construct sequence was analysed to determine its ability to stimulate immune responses, toxicity, and allergenicity. Vaxijen was used to evaluate the physical-chemical properties and predict the ability of the construct sequence to induce immune responses using the method of automatic cross-variance. IL-4Pred and IL-10Pred web servers (Indian Institute Of Technology Delhi, New Delhi, India) were used to assess the ability of the helper T Cell MHC II binding epitopes to induce IL-4 and IL-10 production, respectively.16,17
Construction of the Chimeric Protein
The construct was designed using the epitopes that passed all the analyses described above. The construct comprised AAY sequence for MHC I epitopes merged with GPGPG for MHC II as peptide linker sequences, and KK for B cells epitopes which help in protein folding and its stability.
Solubility and Physicochemical Characteristics
Protein solubility prediction is essential to understand diverse types of biological processes and to explore the impact of different factors (ionic strength, temperature, PH of medium, and electrostatic repulsion) on the productivity of proteins. It also plays an important role in disease analysis and drug development processes.18,19 Solubility index was evaluated using Protein-Sol web tool (The University of Manchester, UK),19 according to the data on Escherichia coli expression to ensure the production of a soluble, folded, and active protein that can be easily extracted from the system. Protein sequence in FASTA format was submitted for solubility determination. Physicochemical characteristics of the chimeric build were determined using ProtParam tool on ExPASy web server (Swiss Institute of Bioinformatics, Lausanne, Switzerland).20 This included the physicochemical properties predicted including aliphatic index, molecular weight, theoretical pI, amino acid and atomic composition, extinction coefficient, estimated half-life, instability index, and grand average of hydropathicity (GRAVY).
Reverse Translation
The chimeric construct in protein format was reverse translated to its nucleotide sequence in order to determine the codon usage index and codon adaptability. This was done with the aid of the EMBOSS Backtranseq tool (EMBL European Bioinformatics Institute, Hixton, UK).21 Amino acid linkers were used to link the chimeric construct to obtain the nucleotide sequence. The EMBOSS Backtranseq tools was then used to reverse translate the protein sequence to its DNA sequence in FASTA format. EMBOSS Backtranseq reads a protein sequence and writes the nucleic acid sequence it is most likely to have come from depending on the codon table selected. In this study E. coli K12 was selected because the reverse translated sequence the Codon Adaptation Index (CAI) will be examined.
Codon Optimisation and Computational Cloning of the Construct
Codon optimisation was achieved using CAI calculator web server by Puigbò P et al.22 CAI calculations and guanine-cytosine percentage were the basis for the codon adaptation sequences analysed. Computational cloning of the optimised epitope sequences were conducted by inserting them into E. coli_pET-24 a(+) vector for expression using the SnapGene version 6.2.1 (Boston, Massachusetts, USA).23 E. coli has been an ideal expression host for large production of recombinant proteins and has been used in the production of inexpensive rapid diagnostic tests.24 The constructs were inserted into the cloning vector and the insertion and restrictions on site were selected and cloned (5,968 bp).
Predicting the Secondary Structure and Tertiary Structure
The structural features of proteins determine a wide range of functions: from binding specificity and conferring mechanical stability, to catalysis of biochemical reactions, transport, and signal transduction.25 In silco pipelines determining functional characteristics of proteins starting from protein sequences benefit heavily from the addition of structural information.26 Protein stability is paramount for ensuring the efficacy and safety of native conformation, preserving their biological activity during manufacturing, storage, and administration. The protein modelling web server SOPMA by Geourjon C and Deléage G27 was used to predict the secondary structure of the chimeric construct by evaluating the repeated order of the adjacent amino acid residues in the polypeptide chain. The 3D structure of the amino acid sequence was assessed with ColabFold web tool by Mirdita M et al.28 Five different models were generated and the rank with the least error (blue) was picked with a very high confidence level (>90) and structural quality.
Validation and Ramachandran Plot and Molecular Docking
The quality of the 3D structure was enhanced by using GalaxyWeb Server (Galux Inc. and Seoul National University, South Korea) a server that predicts protein structure from sequence by template-based modelling as well as refines loop or terminus regions by ab initio modelling.29 The Z scores of the refined structure were obtained using the ProSA-web tool by Wiederstein M and Sippl MJ,30 a widely used tool to check 3D models of protein structures for potential errors. Ramachandran plot was derived using the PROCHECK through UCLA-DOE LAB- SAVES V6.0 (the University of California, Los Angeles, USA) for the evaluation of the tertiary structure.31,32 The interaction between the small molecules and protein of the construct at the atomic level was demonstrated using molecular docking. Several molecular docking web servers were proposed; however, a well-developed and widely used protein-protein and protein-RNA/DNA docking server was selected for the lateral flow design. The HDOCK server by Huang SY and Zou X33 provides a visualisation function whereby users can interactively view the generated docking results. A more negative docking score implies a more possible binding model and roughly, when the confidence score is above 0.5 to 0.7, the two peptides would be possible to bind better. However, when the confidence score is below 0.5, vice versa.34
RESULTS
Retrieval of Sequences
Using specific accession numbers (ABY76794.1, ABY76793.1, WP 009873136.1, WP 009873002.1, WP 015505969.1, WP 013984953.1, QBP33401.1, ABV55512.1, and AAS19616.1), nine protein sequences from Chlamydia trachomatis were retrieved from the NCBI Protein Database in FASTA format.
Antigenicity of the Retrieved Protein Sequences
The score obtained from the prediction of antigenicity of protein sequences using the VaxiJen server predicts the antigenic and non-antigenic amino acid sequences at a threshold of 0.4 based on physicochemical characteristics. Sequences with a score above 0.4 were considered antigenic. All retrieved sequences passed the VaxiJen antigenicity test at a threshold of 0.4, indicating their potential as antigenic targets, which is an essential factor that influences the introduction and effectiveness of the immunological responses.35 Lower thresholds might increase false positives, while higher thresholds might miss potential antigens. The value has been adopted by many studies and databases, ensuring consistency and comparability across different research works.36
Membrane Topology
All sequences qualified as membrane proteins based on TMHMM-2.0 analysis, confirming their potential as targets for immune response. The position of each sequence was accessed to evaluate their location and, according to the result, all selected sequence were extracellular, suggesting the quicker immunological response and detection.37,38
Linear B Cell Epitope Prediction
The retrieved protein sequences were further evaluated for B cell epitopes using SVMTriP and the prediction tools on the IEDB online server. B cell epitopes with scores above 0.5 and lengths between 16–20 amino acids were selected. Non-allergenic epitopes were confirmed using AllerTOP v. 2.0 (Medical University of Sofia, Bulgaria). Only non-allergenic epitopes were chosen.
HTL Epitope Prediction
To select the ideal HTL epitopes from Chlamydia trachomatis proteins, the IEDB tool known as ‘MHC-II Binding Predictions’ was used and this tool generates a “Percentile rank” for each probable peptide.39 The strong binders with a score of ≤2.0 were selected. After testing the HTL epitopes for IL4 and IL10-inducing capability, nine IL4 and eight IL10-inducing peptides were selected for their strong binding affinity (score ≤2.0) and potential to enhance immune response.
Chimeric Construct, Physicochemical Properties of the Chimeric Construct
The chimeric construct, comprising nine B cell epitopes, nine IL4 inducers, and eight IL10 inducers, was designed using specific linkers (KK, GPGPG, and GPGPG) to ensure proper folding and stability. Physicochemical properties were analysed using Protein-Sol and Expasy ProtParam tools, confirming its solubility, antigenicity, and stability (Table 1).
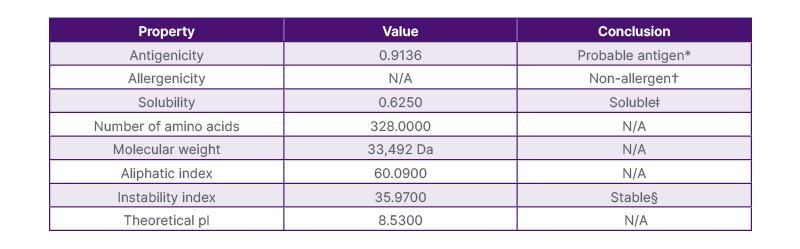
Table 1: Physicochemical properties of the primary construct.
*Antigenicity was determined using VaxiJen with a threshold of 0.4.
†Allergenicity was assessed using AllerTOP v. 2.0.
ǂSolubility was evaluated using Protein-Sol, with values above 0.5 considered soluble.
§An instability index below 40 indicates a stable protein.
The average length of amino acid is 328 with a theoretical pI of 8.53. The structure had a molecular weight of 33.492 KDa; a solubility of 0.625, indicating it is a soluble protein; and an aliphatic index of 60.09, indicating thermostability. Instability index of 35.97 classifying the protein as stable, instability index of ≥40 is considered unstable. Thus, the primary construct is non-allergenic, antigenic, and stable in a variety of temperatures.
The construct’s potential efficacy and safety in therapeutic applications is highlighted by the clinical significance of its high antigenicity and non-allergenicity. Strong and targeted immune responses are influenced by high antigenicity.40 The construct’s non-allergenicity ensures safety, lowers the possibility of negative reactions, and boosts patient compliance, making it appropriate for a variety of people, including those with hypersensitivities. All of these characteristics highlight the construct’s potential as a viable option for clinical application, addressing safety and efficacy issues that are essential for effective therapeutic and preventive measures.41
Reverse Translation and Codon Optimisation
The guanine-cytosine sequences were optimised after reverse translating the primary construct’s protein sequence into nucleotides in E. coli K12 expression systems. The reverse translation and codon optimisation results yielded a guanine-cytosine content of 61.9% with a CAI score of 0.703, indicating the constructs’ stability and excellent possibility of expression in the E. coli K12 expression system. Hence, the construct is stable in designing the lateral flow kit for diagnosis.
Computational Cloning
The team performed computational cloning to assess the capacity of the cloning and expression of the primary construct in an appropriate vector system. They modified the codon of their peptide using the E. coli K12 expression system’s codon usage method in the SnapGene tool and cloned it in pET-24 a (+). The pET-24a (+) vector is used for high-level expression of the primary construct in E. coli, facilitated by the T7 promoter and kanamycin selection marker. The highlighted restriction sites indicate the cloning strategy employed regions that were replaced were PaeR71(158) – BamHI (198) and BamHI (198) – PaeR71(158). The fragments were cut at HindIII (65) – BsrBI (376) and BsrBI (376)-HindIII (65) (Figure 1). Computational cloning in the pET-24a(+) vector confirmed the successful insertion and expression potential of the primary construct.
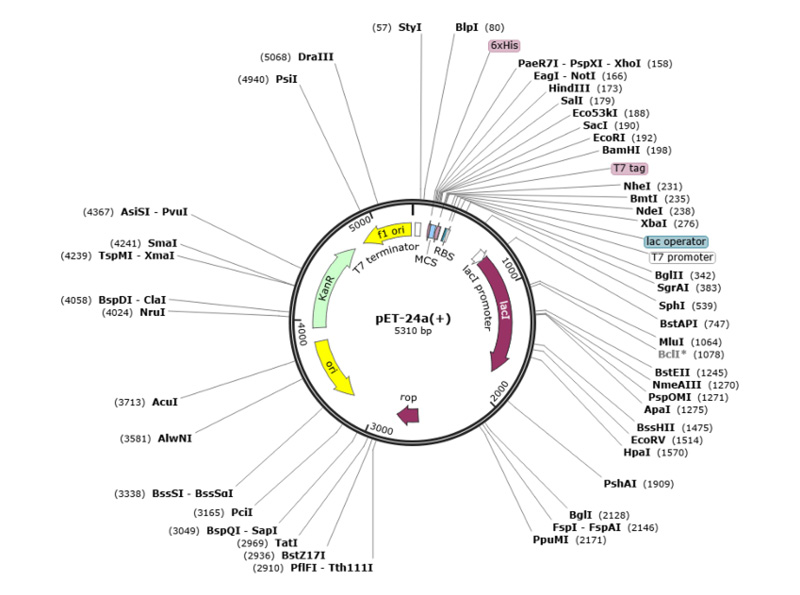
Figure 1: Cloning strategy for the primary construct into the pET-24a (+) expression vector.
The diagram shows the positions of various restriction sites and key features of the vector, including the T7 promoter, lac operator, and kanamycin resistance gene. Restriction sites used for cloning (PaeR71 and BamHI).
Secondary Structure and Tertiary Structure Prediction
The authors predicted the secondary structure of the lateral flow assay construct using the SOPMA server, which explained the distributions with 19.82% alpha helix, 19.51% extended strands, 5.18% beta turns, and 55.49% random coil. Secondary structure prediction revealed a high percentage of random coils and extended strands, indicating the potential for forming antigenic epitopes. Tertiary structure analysis using ColabFold confirmed the structural integrity. Collabfold analysis of the tertiary structure of the lateral flow assay constructed revealed that the predicted local distance difference test is less than 50 and the template modelling score is less than 0.02.
Protein Structure Analysis ProSA-web, and Validation
ProSA-web estimated and verified the whole quality of the basic 3D model and analysed the refined protein structure for errors. ProSA-web analysis validated the 3D model with a Z-score of -5.1, confirming the model’s accuracy and precision (Figure 2).
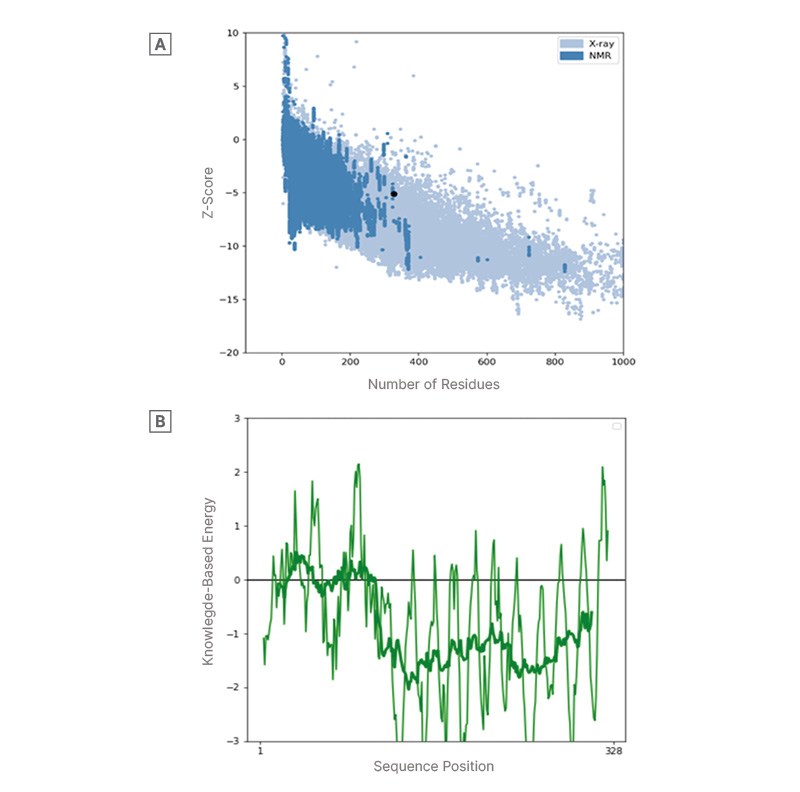
Figure 2: A) Overall 3D model quality and B) local 3D model quality.
Protein structure analysis by ProSA-web. A) The overall 3D model quality is assessed by the Z-score, which compares the model to known structures determined by X-ray and NMR. A Z-score within the range of native proteins indicates a reliable model. B) The local 3D model quality is assessed by the knowledge-based energy profile, with values below 0 indicating energetically favourable and likely correct regions of the protein. The X-axis represents the sequence position (residues 1 to 328), and the Y-axis represents the knowledge-based energy. the Y-axis represents the knowledge-based energy.
Ramachandran plot for the validation of the refined tertiary structure was generated through SAVES v6.0 via PROCHECK. The Ramachandran plot showed 95.4% of amino acids in favourable regions indicating that the stereochemical quality of protein structures by plotting the phi (ϕ) and psi (ψ) dihedral angles of each amino acid residues in the peptide,42 making the construct’s suitability for lateral flow test design. The results further indicated that 3.8% of the amino acids were in the allowable region and 0.8% plotted in the disallowed region, the permitted and prohibited regions of dihedral angles (ϕ and ψ) for amino acid residues, is used to verify the tertiary structure of a protein. It is generally agreed upon that a minimum of 95.4% of residues must lie inside the preferred zones.43 Furthermore, 95.4% threshold is based on statistical analysis of known protein structures and is widely accepted in structural biology as a marker of good-quality models.44
Molecular Docking
The HDOCK server predicts a low sequence ID (14.6%) and a medium TM score (0.66576) for the receptor. The ligand score and Max Sub are 0.333 and 0.053, respectively. Molecular docking using the HDOCK server identified Model 1 with a confidence score of 0.9093 and a docking score of -265.28, suggesting strong binding affinity and stability.
DISCUSSION
Lateral flow test kits are essential tools for rapid and point-of-care diagnostics, relying on the sensitivity, specificity, accuracy, and reliability of their primary, secondary, and tertiary constructs to detect target analytes. From the results, it is established that our primary constructs demonstrate high antigenicity (0.9136), non-allergenicity, and good solubility (0.634), aligning with the report that high-quality monoclonal antibodies enhance test sensitivity and specificity, leading to better diagnostics accuracy.45 The construct’s instability index (35.97) classifies it as stable, with an aliphatic index of 60.09 and a GRAVY score of -0.456, confirming its robustness under various environmental conditions.
The SOPMA analysis of the secondary constructs revealed a favourable distribution of alpha helices (19.82), extended strands (19.51%), beta turns (5.18%), and random coils (55.49%), supporting the protein’s stability and binding potential, consistent with previous studies on protein structure and stability.27,46 Also, supported by Posthuma-Trumpie et al.,47 which highlighted the importance of selecting appropriate secondary constructs, as they affect the sensitivity and specificity of the test.
According to a study by Linares et al.,48 the inclusion of a control line in a lateral flow test kit improves the test’s accuracy and reliability. The tertiary construct, validated by AlphaFold prediction and refined to a 73.5% quality factor, meets these standards. The control lines of the tertiary construct analysis are included to monitor the assay’s performance and reduce the risk of false-positive or false-negative results. Comparing the study by Linares et al.48 to this tertiary construct test analysis, the model 5 ranked 1 from AlphaFold prediction based on post translational modulation score and predicted local distance difference test per-residue confidence level. The predicted local distance difference test was 28.8 and the low post translational modulation was 0.186, this was further refined to meet a 73.5% quality factor. Protein structure analysis and Ramachandran’s plot shows the allowed conformations of amino acid residues in the protein backbone. It is evident that the conformation of the polypeptides and proteins with the standard four regions of the plot correspond to the different secondary structures of proteins: alpha helices, beta sheets, turns, and random coil. Ramachandran et al.,49 established that allowed conformations of amino acid residues in each of these regions are determined by the steric interactions between the atoms in the backbone and the side chains of neighbouring residues which shows that certain combinations of ϕ and ψ angles are sterically unfavourable and thus energetically disfavoured, while others are allowed and energetically favourable. With plot statistics showing 95.4% favoured region, 3.8% allowed region, and 0.8% disallowed region of the total of 328 residues, the conformity to the alpha helix region of the plot characterised by a tight cluster of points around the ϕ=-60° and ψ=-45° angles, hence an ideal helical conformation. The beta sheet region, on the other hand, is characterised by two clusters of points around the ϕ=-120° and ψ=120°, and ϕ=120° and ψ=-120° angles; this shows the two possible orientations of the beta strand.32
SOPMA predictions indicate a stable folding pattern with significant alpha helices and random coils, consistent with previous studies on protein structure and stability.27,46,50 Similarly, Yan et al.,50 used SOPMA to predict the secondary structure of a protein called SARS-CoV-2 Nsp15 and identified potential drug targets for the treatment of COVID-19. Having Alpha helix (Hh) at 19.82%, Extended strand (Ee) at 19.51%, Beta turn (Tt) at 5.18%, Random coil (Cc) at 55.49%, inferring the protein’s overall folding pattern and potential stability as okay. SOPMA reveals a similarity threshold at 8 and having 4 numbers of states suggesting that the protein is involved in binding to other molecules or participating in signalling pathways that require a rigid, stable structure.27,50 The Z-score and residue score obtained further validated the constructed protein structure analysis to ensure the quality and accuracy of our protein model. The ProSA-web tool was used for this validation due to its wide acceptability and ability to evaluate protein structures.51 A high positive Z-score indicates that the structure is significantly more stable than random structures.52 With a Z-score of -5.1 across the X-ray and NMR regions as shown in Figure 2, which is significantly lower than the expected value for a random protein of similar size and composition. On further examination, the residue score plot generated by ProSA-web. The plot indicates that several residues in the protein structure have low negative residue scores, indicating suitability.
A fundamental physicochemical property of this construct is its stability. Stability refers to the protein’s ability to maintain its 3D structure and function under various environmental stresses, including temperature, humidity, and light.53 The construct exhibits strong physicochemical stability, evident from its low instability index (35.97), favourable aliphatic index (60.09), and GRAVY score (-0.456).53 This stability suggests its robustness under diverse environmental conditions, potentially making it suitable for applications requiring long-term storage or use in harsh environments.
Solubility is another crucial property for pharmaceuticals, as it directly affects bioavailability and drug efficacy.54 The predicted high scaled solubility (0.634) of the construct indicates good bio accessibility, suggesting its potential for efficient absorption into the bloodstream upon administration.54 This is a critical factor for maximising drug efficacy.
In the context of lateral flow test development, molecular docking simulations played a key role. The team achieved strong binding affinity and specificity by modelling the interaction between the antigen and this protein construct, as evidenced by the TM score of 0.66576. This score indicates good model quality and comprehensive coverage of both the antigen binding site (receptor, 0.996) and the protein construct itself (ligand, 0.085). This can be particularly useful when designing synthetic protein constructs for use in lateral flow tests.55 With the homology model and TM score of 0.66576, it is established that the binding affinity and specificity of the receptor and ligand molecules are on the correct and medium spectrum exhibiting good coverage by both receptor (0.996) and ligand (0.085). This optimised construct design, facilitated by molecular docking, is essential for achieving the high sensitivity and specificity required for effective lateral flow test performance. This approach aligns well with established methodologies in lateral flow test development, where molecular docking is a valuable tool for optimising antigen-antibody interactions.55,56
CONCLUSION
This study demonstrated that the designed lateral flow test kit is highly effective and viable for the early detection of Chlamydia trachomatis, which can be deployed as a stand-alone or used as combinational therapeutic with the gold standards (nucleic acid amplification tests not excluding strand displacement amplification, PCR, and transcription-mediated amplification) for the rapid testing of Chlamydia trachomatis. The kit as detailed in our research provides public health the accessibility for rapid and widespread screening, facilitating potential increase in test rates thereby controlling spread with reduction in long-term complications.
The development of this lateral flow test kit represents a significant advancement in infectious disease diagnostics considering the speed and convenience, cost effectiveness, and portability supporting the privilege for onsite testing including remote and underserved areas where laboratory facilities are not available.
Future research should reflect opportunities with immunoinformatics where expanded use through broader applications of molecular test kits becoming the norm for major diagnostics, providing global health impact the use of scalable deployment and policy integration enhancing overall disease surveillance and control not just for chlamydia but for other pathogens.