BACKGROUND AND AIMS
Comprehending the rate at which ischaemic myocardium succumbs to necrosis following an abrupt occlusion of a coronary artery is crucial to understand the efficacy and benefits behind the time dependency in ST-elevated myocardial infarction (STEMI) management.1 Over decades, efforts to shave off life-saving minutes from STEMI care centred on reducing door-to-needle and door-to-balloon times.2 Additionally, registry data have shown that between 9% and 31% of patients with STEMI present >12 hours from the symptom onset.3,4 By shifting focus to symptom-to-balloon time and a patient’s self-perception of disease and initiative to seek care, the authors aimed to empower patients and improve outcomes through an accurate, accessible, and cost-effective artificial intelligence-driven single-lead ECG STEMI detection algorithm that can be embedded into wearable devices and employed in a self-administered fashion.
MATERIALS AND METHODS
The authors examined 11,567 12-lead ECG records from Mexico, Colombia, Argentina, and Brazil from April 2014 to December 2019. These were of 10 seconds in length, with a sampling frequency of 500 Hz, and included the following balanced classes: angiographically confirmed and unconfirmed STEMI; branch blocks; nonspecific ST-T abnormalities; and normal and abnormal (200+ current procedural terminology codes, excluding those mentioned above). These were preprocessed by discarding the first and last 250 samples, which may have contained a standardisation pulse. A fifth-order digital low-pass filter with a frequency cut-off of 35 Hz was applied, and the mean was subtracted from each lead. The determined classes were STEMI (including confirmed and unconfirmed STEMI in different locations of the myocardium [anterior, inferior, and lateral]) and Not-STEMI (combination of randomly sampled branch blocks, nonspecific ST-T changes, and normal and abnormal records [25% of each]). A one-dimensional convolutional neural network was then trained and tested with a dataset proportion of 90% and 10%, respectively. A different model was trained and tested for each ECG lead, using the central 4,500 samples of the records. The last dense layer outputted a probability for each report of being STEMI or Not-STEMI. Lead V2 showed the best overall results. The model was further tested through the same methodology using the best lead (V2) with a subset of the previous data, excluding the unconfirmed STEMI ECG records (a total of 7,230 12-lead ECG records for confirmed STEMI only dataset) to seek potential false negative or positive sources. Performance metrics were reported for each experiment and compared.
RESULTS
The combined STEMI data had an accuracy of 91.2%, a sensitivity of 89.6%, and a specificity of 92.9%. In the confirmed STEMI only dataset, accuracy was 92.4%, sensitivity was 93.4%, and specificity was 91.4% (Table 1).
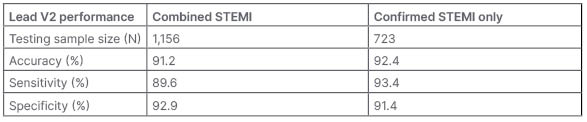
Table 1: Accuracy, sensitivity, and specificity of the combined ST-elevated myocardial infarction and confirmed ST-elevated myocardial infarction only datasets.
STEMI: ST-elevated myocardial infarction.
CONCLUSION
By assiduously improving the model’s input data quality (confirmed only versus both confirmed and unconfirmed STEMI ECGs), the authors continue to assess their algorithm’s performance and reliability for future clinical validation as a potential wearable remote monitoring and early STEMI detection device.